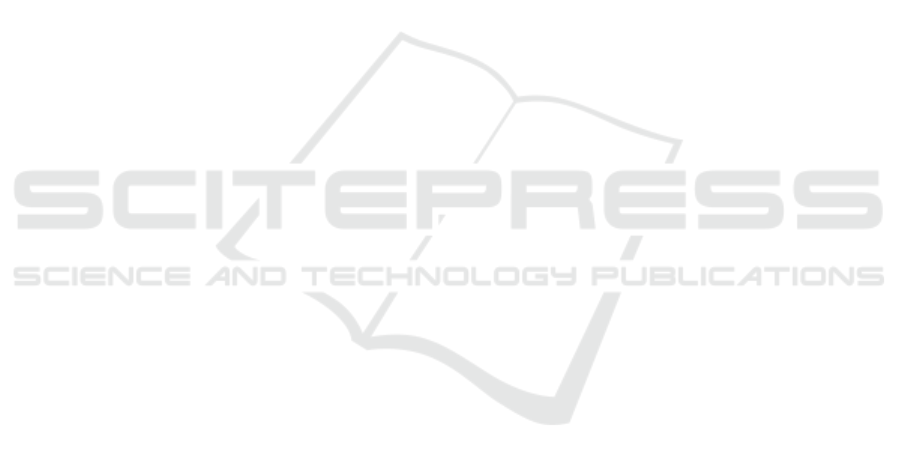
and nitrogen status estimation in precision agriculture:
A review. Computers and Electronics in Agriculture,
151:61 – 69.
Chua, S. N. D., Lim, S. F., Lai, S. N., and Chang,
T. K. (2019). Development of a child detection sys-
tem with artificial intelligence using object detection
method. Journal of Electrical Engineering & Tech-
nology, 14(6):2523–2529.
Ciaparrone, G., Snchez, F. L., Tabik, S., Troiano, L., Tagli-
aferri, R., and Herrera, F. (2020). Deep learning in
video multi-object tracking: A survey. Neurocomput-
ing, 381:61 – 88.
Ding, Y. and Xiao, J. (2012). Contextual boost for pedes-
trian detection. In 2012 IEEE Conference on Com-
puter Vision and Pattern Recognition, pages 2895–
2902.
Espejo-Garcia, B., Martinez-Guanter, J., Prez-Ruiz, M.,
Lopez-Pellicer, F. J., and Zarazaga-Soria, F. J. (2018).
Machine learning for automatic rule classification of
agricultural regulations: A case study in spain. Com-
puters and Electronics in Agriculture, 150:343 – 352.
Fujiyoshi, H., Hirakawa, T., and Yamashita, T. (2019). Deep
learning-based image recognition for autonomous
driving. IATSS Research, 43(4):244 – 252.
Girshick, R. (2015). Fast r-cnn. In 2015 IEEE International
Conference on Computer Vision (ICCV), pages 1440–
1448.
Gong, Z., Lin, H., Zhang, D., Luo, Z., Zelek, J., Chen,
Y., Nurunnabi, A., Wang, C., and Li, J. (2020). A
frustum-based probabilistic framework for 3d object
detection by fusion of lidar and camera data. IS-
PRS Journal of Photogrammetry and Remote Sensing,
159:90 – 100.
Hendry and Chen, R.-C. (2019). Automatic license plate
recognition via sliding-window darknet-yolo deep
learning. Image and Vision Computing, 87:47 – 56.
Hu, J., Huang, J., Gao, Z., and Gu, H. (2018). Position
tracking control of a helicopter in ground effect us-
ing nonlinear disturbance observer-based incremental
backstepping approach. Aerospace Science and Tech-
nology, 81:167 – 178.
Kopp, M., Tuo, Y., and Disse, M. (2019). Fully automated
snow depth measurements from time-lapse images ap-
plying a convolutional neural network. Science of The
Total Environment, 697:134213.
Li, X., Zeng, Z., Shen, J., Zhang, C., and Zhao, Y. (2018).
Rectification of depth measurement using pulsed ther-
mography with logarithmic peak second derivative
method. Infrared Physics & Technology, 89:1 – 7.
Li, Z., Dong, M., Wen, S., Hu, X., Zhou, P., and Zeng, Z.
(2019). Clu-cnns: Object detection for medical im-
ages. Neurocomputing, 350:53 – 59.
Partel, V., Kakarla, S. C., and Ampatzidis, Y. (2019). Devel-
opment and evaluation of a low-cost and smart tech-
nology for precision weed management utilizing arti-
ficial intelligence. Computers and Electronics in Agri-
culture, 157:339 – 350.
Patrcio, D. I. and Rieder, R. (2018). Computer vision and
artificial intelligence in precision agriculture for grain
crops: A systematic review. Computers and Electron-
ics in Agriculture, 153:69 – 81.
Redmon, J. and Farhadi, A. (2017). Yolo9000: Better,
faster, stronger. In 2017 IEEE Conference on Com-
puter Vision and Pattern Recognition (CVPR), pages
6517–6525.
Reiss, D., Hoekzema, N., and Stenzel, O. (2014). Dust
deflation by dust devils on mars derived from opti-
cal depth measurements using the shadow method in
hirise images. Planetary and Space Science, 93-94:54
– 64.
Ren, S., He, K., Girshick, R., and Sun, J. (2015). Faster
r-cnn: Towards real-time object detection with region
proposal networks. In Cortes, C., Lawrence, N. D.,
Lee, D. D., Sugiyama, M., and Garnett, R., editors,
Advances in Neural Information Processing Systems
28, pages 91–99. Curran Associates, Inc.
Sadgrove, E. J., Falzon, G., Miron, D., and Lamb,
D. W. (2018). Real-time object detection in agricul-
tural/remote environments using the multiple-expert
colour feature extreme learning machine (mec-elm).
Computers in Industry, 98:183 – 191.
Shin, J.-Y., Kim, K. R., and Ha, J.-C. (2020). Seasonal fore-
casting of daily mean air temperatures using a coupled
global climate model and machine learning algorithm
for field-scale agricultural management. Agricultural
and Forest Meteorology, 281:107858.
Shinde, S., Kothari, A., and Gupta, V. (2018). Yolo based
human action recognition and localization. Proce-
dia Computer Science, 133:831 – 838. International
Conference on Robotics and Smart Manufacturing
(RoSMa2018).
Silva, J. V., de Castro, C. G. G., Passarelli, C., Espinoza,
D. C., Cassiano, M. M., Raulin, J.-P., and Valio, A.
(2020). Optical depth measurements at 45 and 90
ghz in casleo. Journal of Atmospheric and Solar-
Terrestrial Physics, 199:105214.
Taiana, M., Nascimento, J. C., and Bernardino, A. (2013).
An improved labelling for the inria person data set for
pedestrian detection. In Sanches, J. M., Mic
´
o, L.,
and Cardoso, J. S., editors, Pattern Recognition and
Image Analysis, pages 286–295, Berlin, Heidelberg.
Springer Berlin Heidelberg.
Wang, L., Fan, X., Chen, J., Cheng, J., Tan, J., and Ma, X.
(2020). 3d object detection based on sparse convolu-
tion neural network and feature fusion for autonomous
driving in smart cities. Sustainable Cities and Society,
54:102002.
Wu, X., Sahoo, D., and Hoi, S. C. (2020). Recent advances
in deep learning for object detection. Neurocomput-
ing.
Zhao, Y., Mehnen, J., Sirikham, A., and Roy, R. (2017).
A novel defect depth measurement method based on
nonlinear system identification for pulsed thermo-
graphic inspection. Mechanical Systems and Signal
Processing, 85:382 – 395.
Zhou, T., Ruan, S., and Canu, S. (2019). A review: Deep
learning for medical image segmentation using multi-
modality fusion. Array, 3-4:100004.
Deep Learning Algorithm for Object Detection with Depth Measurement in Precision Agriculture
497