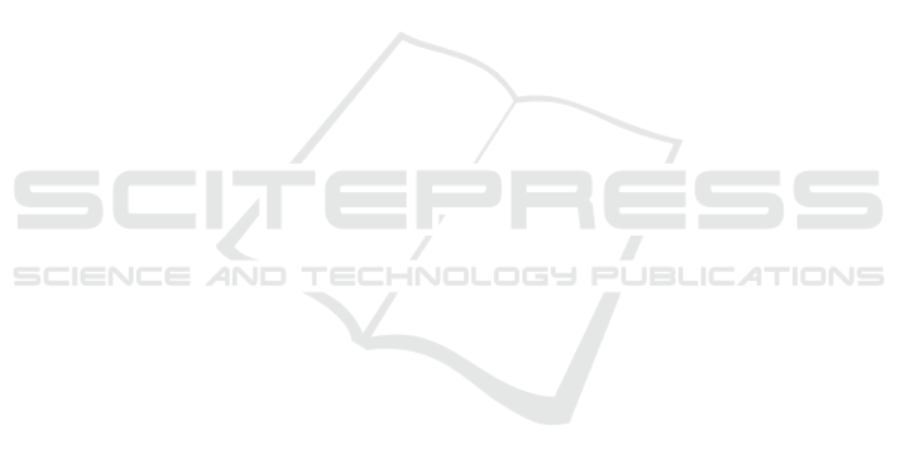
5 CONCLUSION AND
PERSPECTIVES
This work addresses the general problem of the pro-
tection of the privacy of a user during a remote exami-
nation. It seeks to define the different forms it can take
fraud, as well as the expected properties of a secure
biometric anti-cheat system that respects the privacy
of learners. To conclude, the integration of biomet-
rics into distance learning systems will help teachers
to effectively control student authentication, course
tracking, provide certificates in an automated manner,
analyse student behaviour during exams, the valida-
tion of the certificates of success as well as the detec-
tion of fraud with a high level of accuracy. The pro-
posed solution is effective against identity theft and
fraud attempts. Indeed, this system is able to detect
fraud automatically, it respects the privacy and confi-
dentiality of the data exchanged and solves an impor-
tant part of a major problem.
Concerning perspectives, we intend to evaluate
the system performance after merging several modal-
ities (face, keystroke dynamics, gaze, gestures and so
on) then, create a fraud model using a larger database.
In addition, the different scores can be combined to
generate a confidence index on the identity of the
learner during the examination. After all, other so-
lutions could be included to detect spoofing attacks.
REFERENCES
Arnautovski, L. (2019). Face recognition technology in the
exam identity authentication system-implementation
concept. Proceedings of Papers, page 50.
Belguechi, R., Cherrier, E., and Rosenberger, C. (2012).
Texture based fingerprint biohashing: Attacks and ro-
bustness. In 2012 5th IAPR International Conference
on Biometrics (ICB), pages 196–201. IEEE.
Belguechi, R., Rosenberger, C., and Ait-Aoudia, S. (2010).
Biohashing for securing minutiae template. In 2010
20th International Conference on Pattern Recogni-
tion, pages 1168–1171. IEEE.
Bicego, M., Lagorio, A., Grosso, E., and Tistarelli, M.
(2006). On the use of sift features for face authentica-
tion. In 2006 Conference on Computer Vision and Pat-
tern Recognition Workshop (CVPRW’06), pages 35–
35. IEEE.
Carey, P. (2018). Data protection: a practical guide to UK
and EU law. Oxford University Press, Inc.
Chauvel, C. 70% des
´
el
`
eves trichent pendant leur scolarit
´
e.
Giot, R., Hemery, B., and Rosenberger, C. (2010). Low
cost and usable multimodal biometric system based on
keystroke dynamics and 2d face recognition. In 2010
20th International Conference on Pattern Recogni-
tion, pages 1128–1131. IEEE.
Giot, R., Rosenberger, C., and Dorizzi, B. (2012). Re-
connaissance du genre par analyse de dynamique de
frappe au clavier sur texte libre.
Gupta, A., Khanna, A., Jagetia, A., Sharma, D., Alekh, S.,
and Choudhary, V. (2015). Combining keystroke dy-
namics and face recognition for user verification. In
2015 IEEE 18th International Conference on Com-
putational Science and Engineering, pages 294–299.
IEEE.
Idrus, S. Z. S., Cherrier, E., Rosenberger, C., and Bours, P.
(2013). Soft biometrics for keystroke dynamics. In
International Conference Image Analysis and Recog-
nition, pages 11–18. Springer.
Kotropoulos, C., Tefas, A., and Pitas, I. (2000). Morpho-
logical elastic graph matching applied to frontal face
authentication under well-controlled and real condi-
tions. Pattern Recognition, 33(12):1935–1947.
Liu, Z., Luo, P., Wang, X., and Tang, X. (2015). Deep
learning face attributes in the wild. In Proceedings
of the IEEE international conference on computer vi-
sion, pages 3730–3738.
Migdal, D. and Rosenberger, C. (2018). Towards a personal
identity code respecting privacy.
P. Beust, V. Cauchard, I. D. (2016). Enseigner autrement.
Parkhi, O. M., Vedaldi, A., and Zisserman, A. (2015). Deep
face recognition.
Saad, M., Charrier, C., and Bovik, A. C. (2012). Eval-
uation de la qualit
´
e des images sans r
´
ef
´
erence
par mod
´
elisation statistique. COmpression
et REpr
´
esentation des Signaux Audiovisuels
(CORESA).
Viola, P. and Jones, M. (2001). Rapid object detection us-
ing a boosted cascade of simple features. In Proceed-
ings of the 2001 IEEE computer society conference on
computer vision and pattern recognition. CVPR 2001,
volume 1, pages I–I. IEEE.
SECRYPT 2020 - 17th International Conference on Security and Cryptography
458