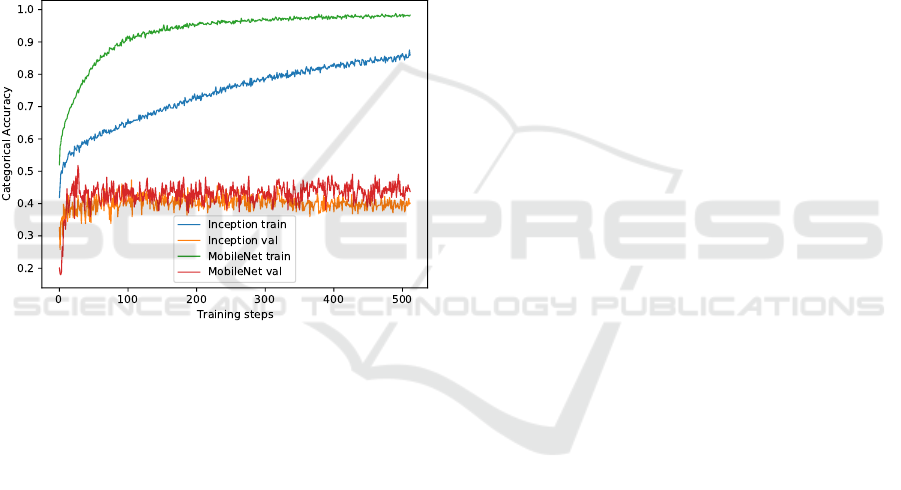
3.2 Multiclass Classification with CNNs
The spectrograms generated for the respective classes
now form the new corpora and serve as input for the
training of the selected models. For this purpose, the
following six classes were deliberately chosen to rep-
resent the technical sounds: Drill, Hammer, Knock,
Sawing, Scrape, Clapping. The multiclass problem
for technical sounds was now addressed with the In-
ceptionV3 model and the MobileNet model.
The runs delivered the following results regarding cat-
egorical accuracy, as can be seen in figure 6.
The Inception Model on the evaluation set approached
the categorical accuracy of 0.5. The training with Mo-
bileNet was convincing with a faster run and delivered
accuracy values on the evaluation set also above 0.5 .
Figure 6: Training of both, the InceptionV3 and the Mo-
bileNet model.
4 CONCLUSIONS
The position paper has introduced two technical
sound classification approaches that included meth-
ods to classify technical sound events by Recurrent
Neural Networks and by Convolutional Neural Net-
works. Experiments on different data sets showed the
advantages of the proposed methods over sound data
transforming to image data. Most of the sound record-
ings have been recorded under real conditions. This
is a great advantage and corresponds to the use case.
However, it is both a difficult situation and a great
challenge, because the sound sources were recorded
from different environments and distances. They con-
tain other interfering noises and the quality can vary
greatly in some cases. One of the most important dis-
coveries so far is the reduction of corpora to selected
classes for RNNs, which improved the classification
results. In the case of the CNNs the results are still
in need of improvement. This will be further pur-
sued in future work. Other models such as VGG19
and DenseNet will be examined and applied. An-
other possibility could be a combined use of so-called
convolutional recurrent neural networks, which is de-
scribed in (Choi et al., 2017). Other options could be
the use of hybrid architectures, which are introduced
in (Choi et al., 2017) and (Feng et al., 2017).
ACKNOWLEDGEMENTS
The work presented in this article is supported and
financed by Zentrales Innovationsprogramm Mittel-
stand (ZIM) of the German Federal Ministry of Eco-
nomic Affairs and Energy. The authors would like
to thank the project management organisation AiF in
Berlin for their cooperation, organisation and budget-
ing.
REFERENCES
Abu-El-Haija, S., Kothari, N., Lee, J., Natsev, P., Toderici,
G., Varadarajan, B., and Vijayanarasimhan, S. (2016).
Youtube-8m: A large-scale video classification bench-
mark. CoRR, abs/1609.08675.
Araujo, A., N
´
egrevergne, B., Chevaleyre, Y., and Atif, J.
(2018). Training compact deep learning models for
video classification using circulant matrices. CoRR,
abs/1810.01140.
AudioSet, G. (2020). Google AudioSet: A large-scale
dataset of manually annotated audio events. Accessed
on 01.03.2020.
Choi, K., Fazekas, G., Sandler, M., and Cho, K. (2017).
Convolutional recurrent neural networks for music
classification. In 2017 IEEE International Confer-
ence on Acoustics, Speech and Signal Processing
(ICASSP), pages 2392–2396.
Feng, L., Liu, S., and Yao, J. (2017). Music genre classifi-
cation with paralleling recurrent convolutional neural
network. CoRR, abs/1712.08370.
Gemmeke, J. F., Ellis, D. P. W., Freedman, D., Jansen,
A., Lawrence, W., Moore, R. C., Plakal, M., and
Ritter, M. (2017). Audio set: An ontology and
human-labeled dataset for audio events. In Proc. IEEE
ICASSP 2017, New Orleans, LA.
Hershey, S., Chaudhuri, S., Ellis, D. P. W., Gemmeke,
J. F., Jansen, A., Moore, R. C., Plakal, M., Platt,
D., Saurous, R. A., Seybold, B., Slaney, M., Weiss,
R. J., and Wilson, K. W. (2016). CNN architec-
tures for large-scale audio classification. CoRR,
abs/1609.09430.
Hochreiter, S. and Schmidhuber, J. (1997). Long short-term
memory. Neural Computation, 9(8):1735–1780.
DeLTA 2020 - 1st International Conference on Deep Learning Theory and Applications
88