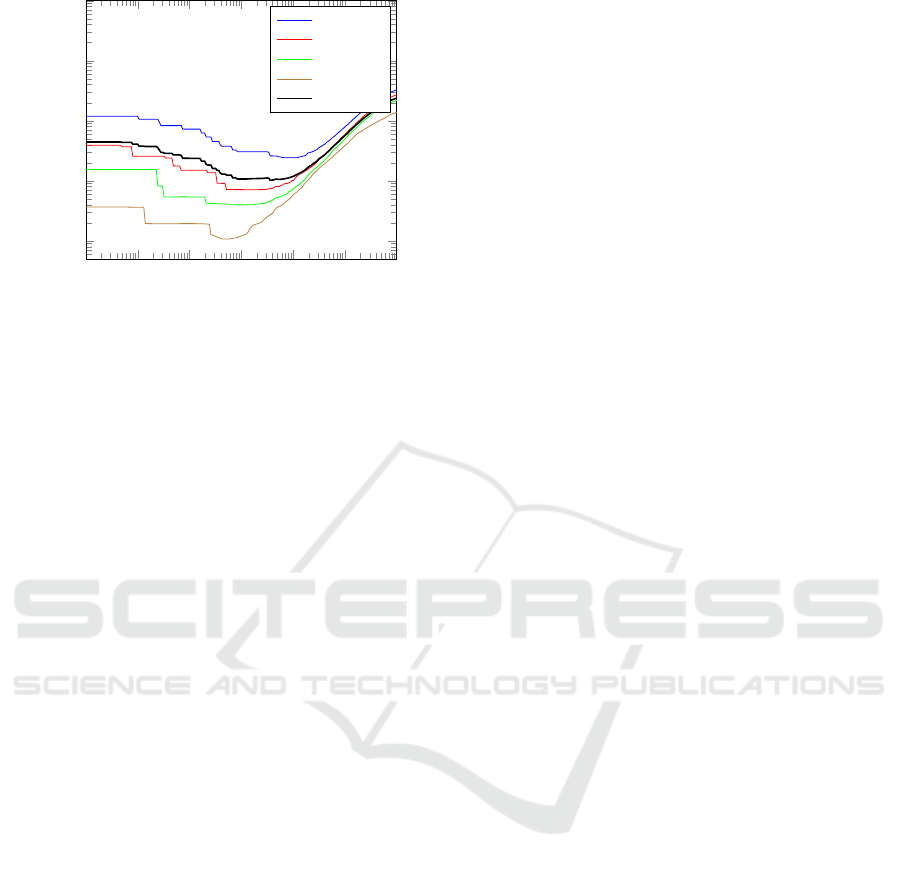
10
−3
10
−2
10
−1
10
0
10
1
10
2
10
3
10
1
10
2
10
3
10
4
10
5
τ (days)
kg
r
1
r
2
r
3
r
4
average
Figure 10: Estimated ammonium mass that is out of speci-
fication until the detection of the fault.
6 CONCLUSIONS
A fault detection strategy has been proposed to detect
faults in the output ammonium sensor of waste water
treatment plants. The plant is assumed to be oper-
ated in closed loop by a controller that measures the
ammonium, and changes the aeration of the reactor.
The faults are assumed to be a slow drift of the mea-
surement. The only signals that are assumed to be
measured are the ammonium sensor, the aeration and
the incoming volumetric water flow. The input am-
monium concentration is not measured. The BSM1
model is used to simulate the behavior of the plant
and validate the proposal.
The fault detection scheme is based on a predic-
tion model that predicts the output ammonium, and
the comparison of the filtered prediction error with a
threshold. The predictor is based on a Volterra se-
ries based IIR model, whose parameters are obtained
through least squares identification.
Despite the modeling errors that produce a bias
in the prediction error, plus the important uncertainty
in the input ammonium concentration, that follows a
quasi periodic daily and weekly pattern, the proposed
fault detector detects the slow varying fault. The pa-
per shows that the detection time depends on the time
constant of the filter, and concludes that there is an
optimum value that minimizes the fault detection time
for a given sensor drift. This optimum value depends
on the magnitude of the fault, so we have proposed
to use the constant time that minimizes the estimated
poured volume of ammonium out of control specifica-
tions for the range of proposed faults. Future research
lines come from studying the behavior of our proposal
in other scenarios as rainy days or storms.
ACKNOWLEDGEMENTS
Supported by MICINN project number TEC2015-
69155-R from the Spanish government, Uni-
versitat Jaume I (UJI-B2018-39) and the grant
ACIF/2018/245 from Generalitat Valenciana.
REFERENCES
Alex, J., Benedetti, L., Copp, J., Gernaey, K., Jeppsson, U.,
Nopens, I., Pons, M., Rieger, L., Rosen, C., Steyer,
J., et al. (2008). Benchmark simulation model no. 1
(bsm1). Report by the IWA Taskgroup on benchmark-
ing of control strategies for WWTPs, pages 19–20.
Behzad, H., Casavola, A., Tedesco, F., Sadrnia, M. A., and
Gagliardi, G. (2018). A fault-tolerant sensor reconcil-
iation scheme based on self-tuning lpv observers. In
ICINCO (1), pages 121–128.
Dovzan, D., Logar, V., Hvala, N., and Skrjanc, I. (2011).
Monitoring and sensor fault detection in a waste-water
treatment process based on a fuzzy model. Elek-
trotehniski Vestnik, 78(3):142–146.
Garc
´
ıa, C. M., Puig, V., and Astorga-Zaragoza, C.-M.
(2017). Fault estimation using a takagi-sugeno in-
terval observer: Application to a pem fuel cell. In
ICINCO.
Henze, M., Grady Jr, C. L., Gujer, W., Marais, G., and
Matsuo, T. (1987). A general model for single-
sludge wastewater treatment systems. Water research,
21(5):505–515.
Jia, X., Cheng, Q., and Hou, Y. (2018). Sensor fault
detection based on state estimation observer in dis-
crete nonlinear systems. In 2018 International Con-
ference on Control, Automation and Information Sci-
ences (ICCAIS), pages 542–547. IEEE.
Kazemi, P., Giralt, J., Bengoa, C., and Steyer, J.-P. (2020).
Data-driven fault detection methods for detecting
small-magnitude faults in anaerobic digestion process.
Water Science and Technology.
Moliner-Heredia, R., Pe
˜
narrocha-Al
´
os, I., and Sanchis-
Llopis, R. (2019). Economic model predictive control
of wastewater treatment plants based on bsm1 using
linear prediction models. In 2019 IEEE 15th Interna-
tional Conference on Control and Automation (ICCA),
pages 73–78. IEEE.
Nagy-Kiss, A. M., Ichalal, D., Schutz, G., and Ragot, J.
(2015). Fault tolerant control for uncertain descrip-
tor multi-models with application to wastewater treat-
ment plant. In 2015 American Control Conference
(ACC), pages 5718–5725. IEEE.
Nagy-Kiss, A. M., Marx, B., Mourot, G., Schutz, G., and
Ragot, J. (2012). Observer synthesis for uncertain
nonlinear systems. application to waste-water treat-
ment plants. IFAC Proceedings Volumes, 45(13):485–
490.
Pinto, R., Rossetti, R. J., and Gonc¸alves, G. (2016). Wire-
less sensor network simulation for fault detection in
industrial processes. In 2016 6th International Con-
ference on Simulation and Modeling Methodologies,
Ammonium Sensor Fault Detection in Wastewater Treatment Plants
687