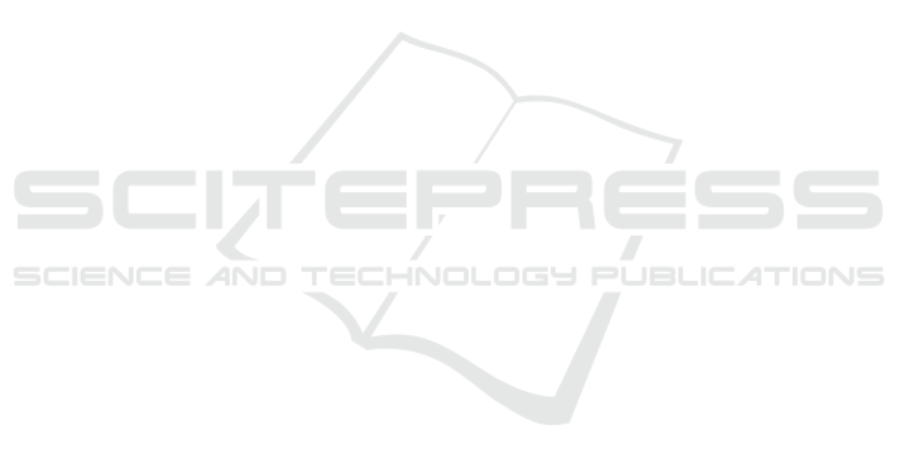
ACKNOWLEDGEMENTS
The authors would like to acknowledge NVIDIA for
supporting their research with the TITAN Xp Graph-
ics processing unit.
REFERENCES
Abadi, M., Barham, P., Chen, J., Chen, Z., Davis, A.,
Dean, J., Devin, M., Ghemawat, S., Irving, G., Isard,
M., et al. (2016). Tensorflow: A system for large-
scale machine learning. In 12th {USENIX} Sympo-
sium on Operating Systems Design and Implementa-
tion ({OSDI} 16), pages 265–283.
Ahmed, E., Jones, M., and Marks, T. K. (2015). An
improved deep learning architecture for person re-
identification. In Proceedings of the IEEE Conference
on Computer Vision and Pattern Recognition, pages
3908–3916.
Ashby, M. P. J. (2017). The value of cctv surveillance cam-
eras as an investigative tool: An empirical analysis.
European Journal on Criminal Policy and Research,
23(3):441–459.
Bazzani, L., Cristani, M., and Murino, V. (2013).
Symmetry-driven accumulation of local features for
human characterization and re-identification. Com-
puter Vision and Image Understanding, 117(2):130–
144.
Bazzani, L., Cristani, M., Perina, A., Farenzena, M.,
and Murino, V. (2010). Multiple-shot person re-
identification by hpe signature. In Proceedings of the
20
th
International Conference on Pattern Recognition,
pages 1413–1416. IEEE.
Bedagkar-Gala, A. and Shah, S. K. (2011). Multiple per-
son re-identification using part based spatio-temporal
color appearance model. In Proceedings of the IEEE
International Conference on Computer Vision Work-
shops (ICCV Workshops), pages 1721–1728. IEEE.
Bromley, J., Guyon, I., LeCun, Y., S
¨
ackinger, E., and Shah,
R. (1994). Signature verification using a” siamese”
time delay neural network. In Proceedings of the
Advances in Neural Information Processing Systems,
pages 737–744.
Cheng, D., Gong, Y., Zhou, S., Wang, J., and Zheng,
N. (2016). Person re-identification by multi-channel
parts-based cnn with improved triplet loss function.
In Proceedings of the IEEE Conference on Computer
Vision and Pattern Recognition, pages 1335–1344.
Ding, S., Lin, L., Wang, G., and Chao, H. (2015). Deep
feature learning with relative distance comparison
for person re-identification. Pattern Recognition,
48(10):2993–3003.
Forss
´
en, P.-E. (2007). Maximally stable colour regions for
recognition and matching. In Proceedings of the IEEE
Conference on Computer Vision and Pattern Recogni-
tion, pages 1–8. IEEE.
Gray, D., Brennan, S., and Tao, H. (2007). Evaluating ap-
pearance models for recognition, reacquisition, and
tracking. In Proceedings of the IEEE International
Workshop on Performance Evaluation for Tracking
and Surveillance (PETS), volume 3, pages 1–7. Cite-
seer.
Guo, Y. and Cheung, N.-M. (2018). Efficient and deep per-
son re-identification using multi-level similarity. In
Proceedings of the IEEE Conference on Computer Vi-
sion and Pattern Recognition, pages 2335–2344.
Jiang, Y., Wang, J., Liang, Y., and Xia, J. (2019). Combin-
ing static and dynamic features for real-time moving
pedestrian detection. Multimedia Tools and Applica-
tions, 78(3):3781–3795.
Kang, J., Cohen, I., and Medioni, G. (2004). Object reac-
quisition using invariant appearance model. In Pro-
ceedings of the 17
th
International Conference on Pat-
tern Recognition, 2004. ICPR 2004., volume 4, pages
759–762. IEEE.
Kingma, D. P. and Ba, J. (2014). Adam: A
method for stochastic optimization. arXiv preprint
arXiv:1412.6980.
Koestinger, M., Hirzer, M., Wohlhart, P., Roth, P. M., and
Bischof, H. (2012). Large scale metric learning from
equivalence constraints. In Proceedings of the IEEE
Conference on Computer Vision and Pattern Recogni-
tion, pages 2288–2295. IEEE.
Li, D., Chen, X., Zhang, Z., and Huang, K. (2017). Learn-
ing deep context-aware features over body and latent
parts for person re-identification. In Proceedings of
the IEEE Conference on Computer Vision and Pattern
Recognition, pages 384–393.
Li, W. and Wang, X. (2013). Locally aligned feature trans-
forms across views. In Proceedings of the IEEE Con-
ference on Computer Vision and Pattern Recognition,
pages 3594–3601.
Li, W., Zhao, R., and Wang, X. (2012). Human reidentifica-
tion with transferred metric learning. In Proceedings
of the Asian Conference on Computer Vision, pages
31–44. Springer.
Li, W., Zhao, R., Xiao, T., and Wang, X. (2014). Deep-
reid: Deep filter pairing neural network for person re-
identification. In Proceedings of the IEEE Conference
on Computer Vision and Pattern Recognition, pages
152–159.
Ma, B., Su, Y., and Jurie, F. (2012). Local descriptors en-
coded by fisher vectors for person re-identification.
In Proceedings of the European Conference on Com-
puter Vision, pages 413–422. Springer.
Meng, J., Wu, S., and Zheng, W.-S. (2019). Weakly su-
pervised person re-identification. In Proceedings of
the IEEE Conference on Computer Vision and Pattern
Recognition, pages 760–769.
Munjal, B., Amin, S., Tombari, F., and Galasso, F. (2019).
Query-guided end-to-end person search. In Proceed-
ings of the IEEE Conference on Computer Vision and
Pattern Recognition, pages 811–820.
Qian, X., Fu, Y., Jiang, Y.-G., Xiang, T., and Xue, X.
(2017). Multi-scale deep learning architectures for
person re-identification. In Proceedings of the IEEE
International Conference on Computer Vision, pages
5399–5408.
SMSNet: A Novel Multi-scale Siamese Model for Person Re-Identification
111