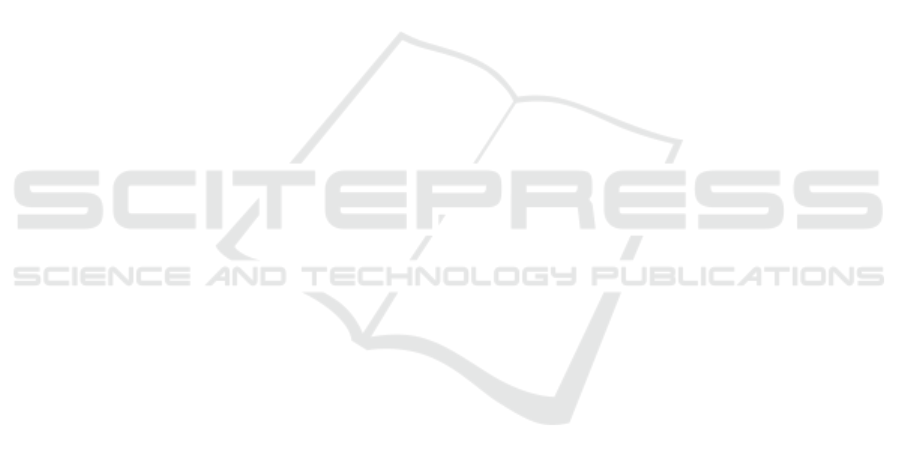
on Informatics in Control, Automation and Robotics
(ICINCO).
Abdu-Aguye, M. G., Gomaa, W., Makihara, Y., and Yagi, Y.
(2019). On the feasibility of on-body roaming models
in human activity recognition. In ICINCO (1), pages
680–690. SciTePress.
Abdu-Aguye, M. G., Gomaa, W., Makihara, Y., and Yagi,
Y. (2020a). Adaptive pooling is all you need: An em-
pirical study on hyperparameter-insensitive human ac-
tion recognition using wearable sensors, accepted. In
IJCNN. The 2020 International Joint Conference on
Neural Networks.
Abdu-Aguye, M. G., Gomaa, W., Makihara, Y., and Yagi, Y.
(2020b). Detecting adversarial attacks in time-series
data, accepted. In ICASSP. IEEE 45th International
Conference on Acoustics, Speech, and Signal Pro-
cessing.
Ashry, S., Elbasiony, R., and Gomaa, W. (2018). An LSTM-
based Descriptor for Human Activities Recognition
using IMU Sensors. In Proceedings of the 15th In-
ternational Conference on Informatics in Control, Au-
tomation and Robotics - Volume 1: ICINCO, pages
494–501. INSTICC, SciTePress.
Ashry, S. and Gomaa, W. (2019). Descriptors for human
activity recognition. In 2019 7th International Japan-
Africa Conference on Electronics, Communications,
and Computations, (JAC-ECC), pages 116–119.
Ashry, S., Ogawa, T., and Gomaa, W. (2020). Charm-deep:
Continuous human activity recognition model based
on deep neural network using imu sensors of smart-
watch. IEEE Sensors Journal.
Bruno, B., Mastrogiovanni, F., Sgorbissa, A., Vernazza, T.,
and Zaccaria, R. (2013). Analysis of human behavior
recognition algorithms based on acceleration data. In
Robotics and Automation (ICRA), 2013 IEEE Interna-
tional Conference on, pages 1602–1607. IEEE.
Cilla, R., Patricio, M. A., Garc
´
ıa, J., Berlanga, A., and
Molina, J. M. (2009). Recognizing human activi-
ties from sensors using hidden markov models con-
structed by feature selection techniques. Algorithms,
2(1):282–300.
Elbasiony, R. and Gomaa, W. (2019). A survey on hu-
man activity recognition based on temporal signals of
portable inertial sensors. In International Conference
on Advanced Machine Learning Technologies and Ap-
plications, pages 734–745. Springer.
Feng, Y., Chang, C. K., and Chang, H. (2016). An adl
recognition system on smart phone. In International
Conference on Smart Homes and Health Telematics,
pages 148–158. Springer.
Gaikwad, K. (2012). Hmm classifier for human activ-
ity recognition. Computer Science & Engineering,
2(4):27.
Gomaa, W., Elbasiony, R., and Ashry, S. (2017). Adl clas-
sification based on autocorrelation function of iner-
tial signals. In Machine Learning and Applications
(ICMLA), 2017 16th IEEE International Conference
on, pages 833–837. IEEE.
Hochreiter, S. and Schmidhuber, J. (1997). Long short-term
memory. Neural computation, 9(8):1735–1780.
Juang, B. . and Rabiner, L. R. (1985). A probabilistic dis-
tance measure for hidden markov models. AT T Tech-
nical Journal, 64(2):391–408.
Kabir, M. H., Hoque, M. R., Thapa, K., and Yang, S.-
H. (2016). Two-layer hidden markov model for hu-
man activity recognition in home environments. In-
ternational Journal of Distributed Sensor Networks,
12(1):4560365.
Karaman, S., Benois-Pineau, J., Dovgalecs, V., M
´
egret,
R., Pinquier, J., Andr
´
e-Obrecht, R., Ga
¨
estel, Y., and
Dartigues, J.-F. (2014). Hierarchical hidden markov
model in detecting activities of daily living in wear-
able videos for studies of dementia. Multimedia tools
and applications, 69(3):743–771.
Khan, A. M., Lee, Y.-K., Lee, S. Y., and Kim, T.-
S. (2010). A triaxial accelerometer-based physical-
activity recognition via augmented-signal features and
a hierarchical recognizer. IEEE transactions on infor-
mation technology in biomedicine, 14(5):1166–1172.
Kreil, M., Sick, B., and Lukowicz, P. (2014). Dealing
with human variability in motion based, wearable ac-
tivity recognition. In 2014 IEEE International Con-
ference on Pervasive Computing and Communication
Workshops (PERCOM WORKSHOPS), pages 36–40.
IEEE.
Lara, O. D. and Labrador, M. A. (2012). A survey
on human activity recognition using wearable sen-
sors. IEEE communications surveys & tutorials,
15(3):1192–1209.
Machado, I. P., Gomes, A. L., Gamboa, H., Paix
˜
ao, V.,
and Costa, R. M. (2015). Human activity data
discovery from triaxial accelerometer sensor: Non-
supervised learning sensitivity to feature extraction
parametrization. Information Processing & Manage-
ment, 51(2):204–214.
Mohammed, S. A. and Gomaa, W. (2016). Exploration of
unknown map for gas searching and picking up ob-
jects using khepera mobile robots. In ICINCO (2),
pages 294–302.
Mohammed, S. A. and Gomaa, W. (2017). Exploration of
unknown map for safety purposes using wheeled mo-
bile robots. In ICINCO (2), pages 359–367.
Sahraeian, S. M. E. and Yoon, B. (2011). A novel low-
complexity hmm similarity measure. IEEE Signal
Processing Letters, 18(2):87–90.
Zhang, M. and Sawchuk, A. A. (2012). Usc-had: a daily ac-
tivity dataset for ubiquitous activity recognition using
wearable sensors. In Proceedings of the 2012 ACM
Conference on Ubiquitous Computing, pages 1036–
1043. ACM.
Improved IMU-based Human Activity Recognition using Hierarchical HMM Dissimilarity
709