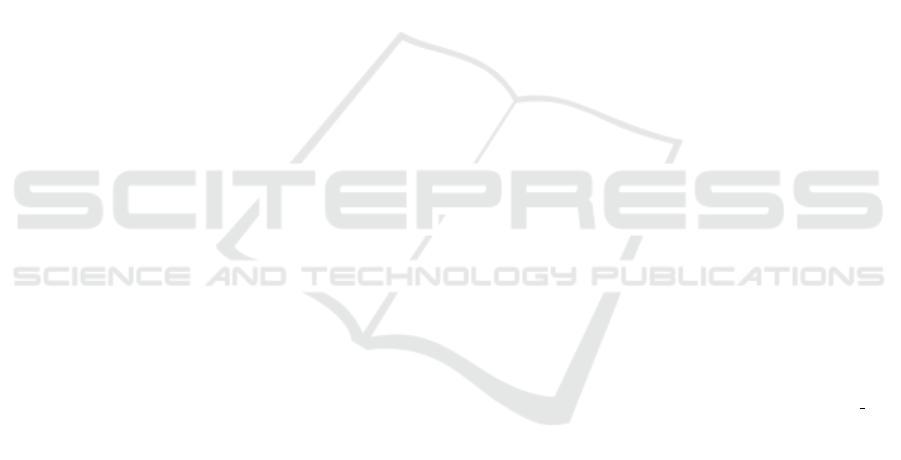
random perturbations of known gene ontological an-
notations. In KDIR 2014 - Proceedings of the In-
ternational Conference on Knowledge Discovery and
Information Retrieval, Rome, Italy, pages 107–116.
SciTePress.
Domeniconi, G., Masseroli, M., Moro, G., and Pinoli, P.
(2016a). Cross-organism learning method to discover
new gene functionalities. Computer methods and pro-
grams in biomedicine, 126:20–34.
Domeniconi, G., Moro, G., Pagliarani, A., and Pasolini, R.
(2017). On deep learning in cross-domain sentiment
classification. In Proceedings of the 9th International
Joint Conference on Knowledge Discovery, Knowl-
edge Engineering and Knowledge Management - (Vol-
ume 1), Funchal, Madeira, Portugal, 2017, pages 50–
60. SciTePress.
Domeniconi, G., Moro, G., Pasolini, R., and Sartori, C.
(2014b). Iterative refining of category profiles for
nearest centroid cross-domain text classification. In
International Joint Conference on Knowledge Discov-
ery, Knowledge Engineering, and Knowledge Man-
agement, pages 50–67. Springer.
Domeniconi, G., Moro, G., Pasolini, R., and Sartori, C.
(2015). A Study on Term Weighting for Text Cate-
gorization: A Novel Supervised Variant of tf. idf. In
DATA, pages 26–37.
Domeniconi, G., Semertzidis, K., Lopez, V., Daly, E. M.,
Kotoulas, S., et al. (2016b). A novel method for
unsupervised and supervised conversational message
thread detection. In DATA, pages 43–54.
Fisher, M. J. and Marshall, A. P. (2009). Understand-
ing descriptive statistics. Australian Critical Care,
22(2):93–97.
Gunning, D. (2017). Explainable Artificial Intelligence
(XAI). Defense Advanced Research Projects Agency
(DARPA), nd Web, 2.
Halko, N., Martinsson, P.-G., and Tropp, J. A. (2011).
Finding structure with randomness: Probabilistic al-
gorithms for constructing approximate matrix decom-
positions. SIAM review, 53(2):217–288.
Jia, R. and Liang, P. (2017). Adversarial exam-
ples for evaluating reading comprehension systems.
arXiv:1707.07328.
Lan, Z., Chen, M., Goodman, S., Gimpel, K., Sharma,
P., et al. (2019). Albert: A lite bert for
self-supervised learning of language representations.
arXiv:1909.11942.
Landauer, T. K. and Dumais, S. T. (1997). A solution to
Plato’s problem: The latent semantic analysis theory
of acquisition, induction, and representation of knowl-
edge. Psychological review, 104(2):211.
Landauer, T. K., Foltz, P. W., and Laham, D. (1998). An in-
troduction to latent semantic analysis. Discourse pro-
cesses, 25(2-3):259–284.
Liu, B. and Zhang, L. (2012). A survey of opinion mining
and sentiment analysis. In Mining text data, pages
415–463. Springer.
Liu, H., Yin, Q., and Wang, W. Y. (2018). Towards explain-
able nlp: A generative explanation framework for text
classification. arXiv:1811.00196.
Maaten, L. v. d. and Hinton, G. (2008). Visualizing data
using t-SNE. Journal of machine learning research,
9(Nov):2579–2605.
Mathews, S. M. (2019). Explainable Artificial Intelli-
gence Applications in NLP, Biomedical, and Malware
Classification: A Literature Review. In Intelligent
Computing-Proc. of the Computing Conference.
Montavon, G., Samek, W., and M
¨
uller, K.-R. (2018). Meth-
ods for interpreting and understanding deep neural
networks. Digital Signal Processing, 73:1–15.
Moosavi-Dezfooli, S.-M., Fawzi, A., Fawzi, O., and
Frossard, P. (2017). Universal adversarial perturba-
tions. In CVPR, pages 1765–1773.
Moro, G., Pagliarani, A., Pasolini, R., and Sartori, C.
(2018). Cross-domain & in-domain sentiment anal-
ysis with memory-based deep neural networks. In
Proceedings of the 10th International Joint Confer-
ence on Knowledge Discovery, Knowledge Engineer-
ing and Knowledge Management, IC3K 2018, Volume
1: KDIR, Seville, Spain, 2018, pages 125–136.
Nassif, A. B., Shahin, I., Attili, I., Azzeh, M., and Shaalan,
K. (2019). Speech recognition using deep neural net-
works: A systematic review. IEEE Access, 7:19143–
19165.
Nguyen, A., Yosinski, J., and Clune, J. (2015). Deep neu-
ral networks are easily fooled: High confidence pre-
dictions for unrecognizable images. In CVPR, pages
427–436.
Pagliarani, A., Moro, G., Pasolini, R., and Domeniconi, G.
(2017). Transfer learning in sentiment classification
with deep neural networks. In Knowledge Discovery,
Knowledge Engineering and Knowledge Management
- 9th International Joint Conference, IC3K 2017, Fun-
chal, Madeira, Portugal, 2017, Revised Selected Pa-
pers, pages 3–25.
Papernot, N., McDaniel, P., Jha, S., Fredrikson, M., Celik,
Z. B., et al. (2016). The limitations of deep learning
in adversarial settings. In EuroS&P, pages 372–387.
Patel, D. and Vaezi, M. (2014). Idiopathic achala-
sia. https://www.orpha.net/consor/cgi-bin/OC
Exp.
php?lng=EN&Expert=930. Accessed 6 Apr 2020.
Reimers, N. and Gurevych, I. (2019). Sentence-bert:
Sentence embeddings using siamese bert-networks.
arXiv:1908.10084.
Riccucci, S., Carbonaro, A., and Casadei, G. (2007).
Knowledge acquisition in intelligent tutoring system:
A data mining approach. In Mexican International
Conference on Artificial Intelligence, pages 1195–
1205. Springer.
Safavian, S. R. and Landgrebe, D. A. (1991). A survey
of decision tree classifier methodology. IEEE Trans.
Systems, Man, and Cybernetics, 21:660–674.
Weiss, S. M., Indurkhya, N., and Zhang, T. (2015). Funda-
mentals of predictive text mining. Springer.
DATA 2020 - 9th International Conference on Data Science, Technology and Applications
132