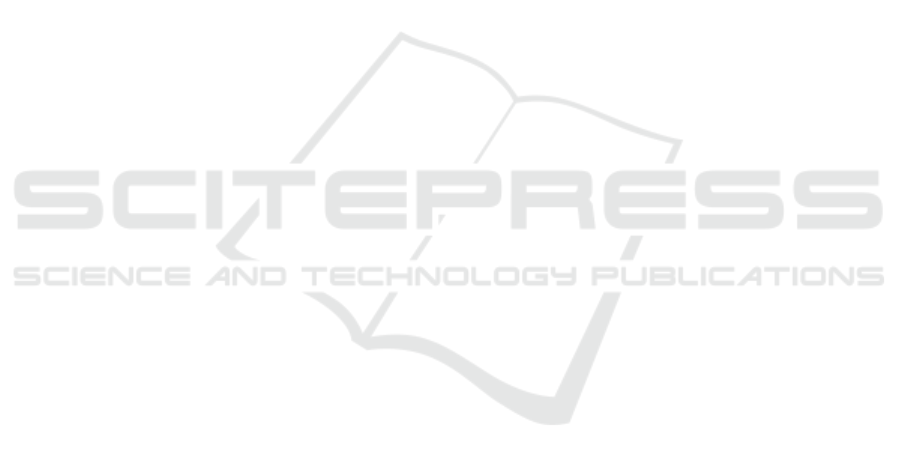
Gan, G., Ma, C., and Wu, J. (2007). Data clustering: theory,
algorithms, and applications, volume 20. Siam.
Gan, J., Li, A., Lei, Q.-L., Ren, H., and Yang, Y. (2017).
K-means based on active learning for support vector
machine. In 2017 IEEE/ACIS 16th International Con-
ference on Computer and Information Science (ICIS),
pages 727–731. IEEE.
Geinitz, S., Margraf, A., Wedel, A., Witthus, S., and Drech-
sler, K. (2016). Detection of filament misalignment in
carbon fiber production using a stereovision line scan
camera system. In Proc. of 19th World Conference on
Non-Destructive Testing, Munich, Germany.
Grira, N., Crucianu, M., and Boujemaa, N. (2004). Unsu-
pervised and semi-supervised clustering: a brief sur-
vey. A review of machine learning techniques for pro-
cessing multimedia content, 1:9–16.
Gu, Q. and Han, J. (2013). Clustered support vector ma-
chines. In Artificial Intelligence and Statistics, pages
307–315.
Hahsler, M. and Bola
˜
nos, M. (2016). Clustering data
streams based on shared density between micro-
clusters. IEEE Transactions on Knowledge and Data
Engineering, 28(6):1449–1461.
Halkidi, M., Batistakis, Y., and Vazirgiannis, M. (2001). On
clustering validation techniques. Journal of intelligent
information systems, 17(2-3):107–145.
Huang, Z. (1998). Extensions to the k-means algorithm for
clustering large data sets with categorical values. Data
mining and knowledge discovery, 2(3):283–304.
Jain, A. K. (2010). Data clustering: 50 years beyond k-
means. Pattern recognition letters, 31(8):651–666.
Ji, X., Henriques, J. F., and Vedaldi, A. (2019). Invariant
information clustering for unsupervised image classi-
fication and segmentation. In Proceedings of the IEEE
International Conference on Computer Vision, pages
9865–9874.
Joachims, T. (1999). Transductive inference for text classi-
fication using support vector machines. In Icml, vol-
ume 99, pages 200–209.
Laskov, P., Gehl, C., Kr
¨
uger, S., and M
¨
uller, K.-R. (2006).
Incremental support vector learning: Analysis, imple-
mentation and applications. Journal of machine learn-
ing research, 7(Sep):1909–1936.
Le, Q. V. (2013). Building high-level features using large
scale unsupervised learning. In 2013 IEEE interna-
tional conference on acoustics, speech and signal pro-
cessing, pages 8595–8598. IEEE.
Lee, J. and Lee, J.-H. (2014). K-means clustering based
svm ensemble methods for imbalanced data prob-
lem. In 2014 Joint 7th International Conference on
Soft Computing and Intelligent Systems (SCIS) and
15th International Symposium on Advanced Intelli-
gent Systems (ISIS), pages 614–617. IEEE.
Margraf, A., Geinitz, S., Wedel, A., and Engstler, L.
(2017a). Detection of surface defects on carbon fiber
rovings using line sensors and image processing algo-
rithms. In Proceedings of the SAMPE Europe Confer-
ence 2017 Stuttgart.
Margraf, A., Stein, A., Engstler, L., Geinitz, S., and H
¨
ahner,
J. (2017b). An Evolutionary Learning Approach to
Self-configuring Image Pipelines in the Context of
Carbon Fiber Fault Detection. In Proceedings of
the 16th IEEE International Conference for Machine
Learning and Applications.
M
¨
uller-Schloer, C. and Tomforde, S. (2017). Organic Com-
puting: Technical Systems for Survival in the Real
World. Autonomic Systems. Birkh
¨
auser, Cham, 1st
edition.
MVTec (2018). Halcon a product of mvtec.
Schubert, E., Sander, J., Ester, M., Kriegel, H. P., and Xu,
X. (2017). Dbscan revisited, revisited: why and how
you should (still) use dbscan. ACM Transactions on
Database Systems (TODS), 42(3):19.
Stein, A., Margraf, A., Moroskow, J., Geinitz, S., and
H
¨
ahner, J. (2018). Toward an organic computing ap-
proach to automated design of processing pipelines. In
ARCS Workshop 2018; 31th International Conference
on Architecture of Computing Systems, pages 1–8.
Teodorescu, H.-N., Dascalescu, L., Hulea, M., and Plo-
peanu, M. C. (2013). Correlations between the elec-
tric charging properties and the optically determined
structure of non-woven fabrics. Journal of Electro-
statics, 71(4):635–647.
Trivedi, S., Pardos, Z. A., and Heffernan, N. T. The utility
of clustering in prediction tasks.
Xu, R. and Wunsch, D. C. (2005). Survey of clustering
algorithms.
Yu, H., Yang, J., and Han, J. (2003). Classifying large data
sets using svms with hierarchical clusters. In Proceed-
ings of the ninth ACM SIGKDD international confer-
ence on Knowledge discovery and data mining, pages
306–315.
Zhang, T., Ramakrishnan, R., and Livny, M. (1996). Birch:
an efficient data clustering method for very large
databases. ACM Sigmod Record, 25(2):103–114.
Towards Self-adaptive Defect Classification in Industrial Monitoring
327