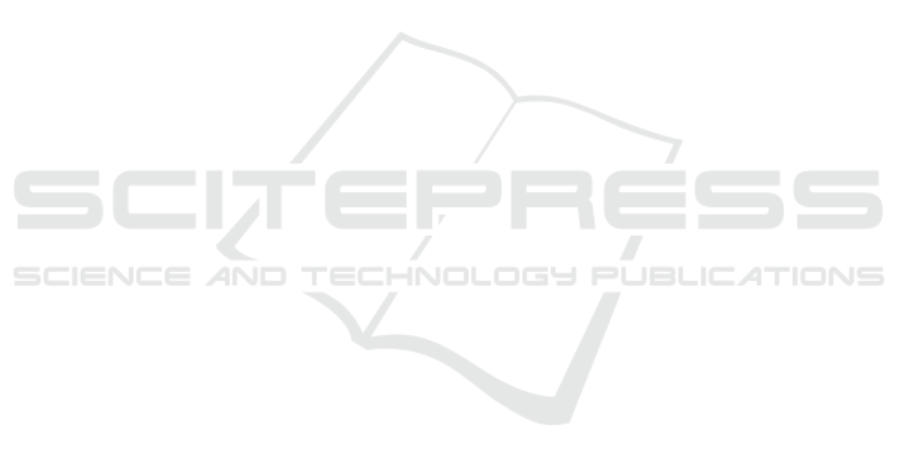
Eysenbach, B., Gupta, A., Ibarz, J., and Levine, S. (2018).
Diversity is all you need: Learning skills without a
reward function. CoRR, abs/1802.06070.
Goodfellow, I. J., Pouget-Abadie, J., Mirza, M., Xu, B.,
Warde-Farley, D., Ozair, S., Courville, A., and Ben-
gio, Y. (2014). Generative Adversarial Networks.
ArXiv e-prints.
Haarnoja, T., Zhou, A., Abbeel, P., and Levine, S.
(2018). Soft Actor-Critic: Off-Policy Maximum En-
tropy Deep Reinforcement Learning with a Stochastic
Actor. ArXiv e-prints.
He, K., Zhang, X., Ren, S., and Sun, J. (2015). Deep Resid-
ual Learning for Image Recognition. arXiv e-prints,
page arXiv:1512.03385.
Jeong, H. and Lee, D. D. (2016). Efficient learning of stand-
up motion for humanoid robots with bilateral symme-
try. In IEEE/RSJ International Conference on Intelli-
gent Robots and Systems, IROS, Daejeon, South Ko-
rea, October 9-14, pages 1544–1549. IEEE.
Mart
´
ınez-Gonz
´
alez,
´
A., Villamizar, M., Can
´
evet, O., and
Odobez, J. (2018). Real-time convolutional networks
for depth-based human pose estimation. In IEEE/RSJ
International Conference on Intelligent Robots and
Systems, IROS, Madrid, Spain, October 1-5, pages
41–47. IEEE.
Mounsif, M., Lengagne, S., Thuilot, B., and Adouane,
L. (2019). Universal Notice Network: Transfer-
able Knowledge Among Agents. 6th 2019 Interna-
tional Conference on Control, Decision and Informa-
tion Technologies (IEEE-CoDIT).
OpenAI, Akkaya, I., Andrychowicz, M., Chociej, M.,
Litwin, M., McGrew, B., Petron, A., Paino, A., Plap-
pert, M., Powell, G., Ribas, R., Schneider, J., Tezak,
N., Tworek, J., Welinder, P., Weng, L., Yuan, Q.,
Zaremba, W., and Zhang, L. (2019). Solving Ru-
bik’s Cube with a Robot Hand. arXiv e-prints, page
arXiv:1910.07113.
Radford, A., Metz, L., and Chintala, S. (2016). Unsu-
pervised representation learning with deep convolu-
tional generative adversarial networks. In Bengio, Y.
and LeCun, Y., editors, 4th International Conference
on Learning Representations, ICLR 2016, San Juan,
Puerto Rico, May 2-4, 2016, Conference Track Pro-
ceedings.
Salimans, T., Goodfellow, I., Zaremba, W., Cheung, V.,
Radford, A., and Chen, X. (2016a). Improved tech-
niques for training gans. In Proceedings of the
30th International Conference on Neural Information
Processing Systems, NIPS’16, page 2234–2242, Red
Hook, NY, USA. Curran Associates Inc.
Salimans, T., Goodfellow, I., Zaremba, W., Cheung, V.,
Radford, A., Chen, X., and Chen, X. (2016b). Im-
proved techniques for training gans. In Lee, D. D.,
Sugiyama, M., Luxburg, U. V., Guyon, I., and Garnett,
R., editors, Advances in Neural Information Process-
ing Systems 29, pages 2234–2242. Curran Associates,
Inc.
Schulman, J., Wolski, F., Dhariwal, P., Radford, A., and
Klimov, O. (2017). Proximal policy optimization al-
gorithms. CoRR, abs/1707.06347.
Silver, D., Huang, A., Maddison, C., Guez, A., Sifre, L.,
van den Driessche, G., Schrittiwiser, J., Antonoglu,
I., Panneershelvam, V., Lanctot, M., Dieleman, S.,
Grewe, D., Nham, J., Kalchbrenner, N., Sustkever, I.,
Lillicrap, T., Leach, M., Kavukcuoglu, K., Graepel,
T., and Hassabis, D. (2015). Mastering the game of
go with deep neural networks and tree search. The
Journal of Nature.
Simonyan, K. and Zisserman, A. (2014). Very deep con-
volutional networks for large-scale image recognition.
CoRR, abs/1409.1556.
Trapit, B., Jakub, P., Szymon, S., Ilya, S., and Igor, M.
(2017). Emergent complexity via multi-agent com-
petition. arXiv - OpenAI Technical Report.
Zintgraf, L. M., Shiarlis, K., Kurin, V., Hofmann, K., and
Whiteson, S. (2018). CAML: Fast Context Adaptation
via Meta-Learning. ArXiv e-prints.
ICINCO 2020 - 17th International Conference on Informatics in Control, Automation and Robotics
96