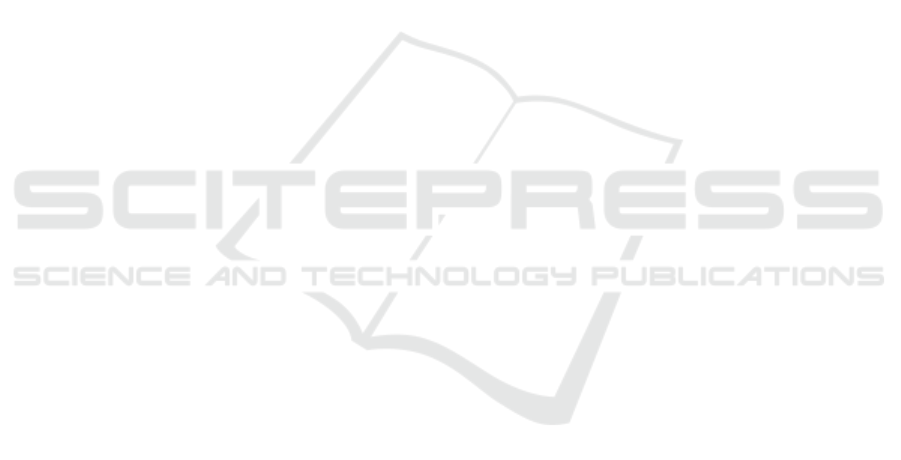
Jing, X.-Y., Wu, F., Dong, X., Shan, S., and Chen, S.
(2017). Semi-supervised multi-view correlation fea-
ture learning with application to webpage classifica-
tion. In Thirty-First AAAI Conference on Artificial In-
telligence.
K
¨
a
¨
ari
¨
ainen, M. (2005). Generalization error bounds using
unlabeled data. In International Conference on Com-
putational Learning Theory, pages 127–142. Springer.
Keele, S. et al. (2007). Guidelines for performing system-
atic literature reviews in software engineering. Tech-
nical report, Technical report, Ver. 2.3 EBSE Techni-
cal Report. EBSE.
Kim, S., Song, Y., Kim, K., Cha, J.-W., and Lee, G. G.
(2006). Mmr-based active machine learning for bio
named entity recognition. In Proceedings of the Hu-
man Language Technology Conference of the NAACL,
Companion Volume: Short Papers, pages 69–72.
Leng, Y., Xu, X., and Qi, G. (2013). Combining active
learning and semi-supervised learning to construct
svm classifier. Knowledge-Based Systems, 44:121–
131.
Li, Y. and Guan, C. (2008). Joint feature re-extraction and
classification using an iterative semi-supervised sup-
port vector machine algorithm. Machine Learning,
71(1):33–53.
Liu, P., Zhang, H., and Eom, K. B. (2016). Active deep
learning for classification of hyperspectral images.
IEEE Journal of Selected Topics in Applied Earth Ob-
servations and Remote Sensing, 10(2):712–724.
Liu, Q., Liao, X., and Carin, L. (2008). Semi-supervised
multitask learning. In Advances in Neural Information
Processing Systems, pages 937–944.
Liu, Y. and Kirchhoff, K. (2013). Graph-based semi-
supervised learning for phone and segment classifica-
tion. In INTERSPEECH, pages 1840–1843.
Madani, O., Pennock, D. M., and Flake, G. W. (2005). Co-
validation: Using model disagreement on unlabeled
data to validate classification algorithms. In Advances
in neural information processing systems, pages 873–
880.
Minakawa, M., Raytchev, B., Tamaki, T., and Kaneda, K.
(2013). Image sequence recognition with active learn-
ing using uncertainty sampling. In The 2013 Interna-
tional Joint Conference on Neural Networks (IJCNN),
pages 1–6. IEEE.
Morsillo, N., Pal, C., and Nelson, R. (2009). Semi-
supervised learning of visual classifiers from web im-
ages and text. In Twenty-First International Joint Con-
ference on Artificial Intelligence.
Nigam, K., McCallum, A., and Mitchell, T. (2006).
Semi-supervised text classification using em. Semi-
Supervised Learning, pages 33–56.
Rajan, S., Ghosh, J., and Crawford, M. M. (2008). An active
learning approach to hyperspectral data classification.
IEEE Transactions on Geoscience and Remote Sens-
ing, 46(4):1231–1242.
Ranzato, M. and Szummer, M. (2008). Semi-supervised
learning of compact document representations with
deep networks. In Proceedings of the 25th interna-
tional conference on Machine learning, pages 792–
799.
Rigutini, L., Maggini, M., and Liu, B. (2005). An em based
training algorithm for cross-language text categoriza-
tion. In The 2005 IEEE/WIC/ACM International Con-
ference on Web Intelligence (WI’05), pages 529–535.
IEEE.
Rosset, S., Zhu, J., Zou, H., and Hastie, T. J. (2005). A
method for inferring label sampling mechanisms in
semi-supervised learning. In Advances in neural in-
formation processing systems, pages 1161–1168.
Schuurmans, D. and Southey, F. (2002). Metric-based meth-
ods for adaptive model selection and regularization.
Machine Learning, 48(1-3):51–84.
Settles, B. (2012). Active learning, volume 6 of synthesis
lectures on artificial intelligence and machine learn-
ing. Morgan & Claypool.
Shi, L., Mihalcea, R., and Tian, M. (2010). Cross lan-
guage text classification by model translation and
semi-supervised learning. In Proceedings of the 2010
Conference on Empirical Methods in Natural Lan-
guage Processing, pages 1057–1067. Association for
Computational Linguistics.
Sindhwani, V., Niyogi, P., and Belkin, M. (2005). A co-
regularization approach to semi-supervised learning
with multiple views. In Proceedings of ICML work-
shop on learning with multiple views, volume 2005,
pages 74–79. Citeseer.
Stikic, M., Larlus, D., and Schiele, B. (2009). Multi-graph
based semi-supervised learning for activity recogni-
tion. In 2009 International Symposium on Wearable
Computers, pages 85–92. IEEE.
Tang, J., Hong, R., Yan, S., Chua, T.-S., Qi, G.-J., and Jain,
R. (2011). Image annotation by k nn-sparse graph-
based label propagation over noisily tagged web im-
ages. ACM Transactions on Intelligent Systems and
Technology (TIST), 2(2):1–15.
Tang, J., Li, H., Qi, G.-J., and Chua, T.-S. (2008). Integrated
graph-based semi-supervised multiple/single instance
learning framework for image annotation. In Pro-
ceedings of the 16th ACM international conference on
Multimedia, pages 631–634.
Tang, J., Li, H., Qi, G.-J., and Chua, T.-S. (2009). Image
annotation by graph-based inference with integrated
multiple/single instance representations. IEEE Trans-
actions on Multimedia, 12(2):131–141.
Tur, G., Hakkani-T
¨
ur, D., and Schapire, R. E. (2005). Com-
bining active and semi-supervised learning for spo-
ken language understanding. Speech Communication,
45(2):171–186.
Varadarajan, B., Yu, D., Deng, L., and Acero, A. (2009).
Maximizing global entropy reduction for active learn-
ing in speech recognition. In 2009 IEEE International
Conference on Acoustics, Speech and Signal Process-
ing, pages 4721–4724. IEEE.
Weston, J., Ratle, F., Mobahi, H., and Collobert, R. (2012).
Deep learning via semi-supervised embedding. In
Neural networks: Tricks of the trade, pages 639–655.
Springer.
ICSOFT 2020 - 15th International Conference on Software Technologies
560