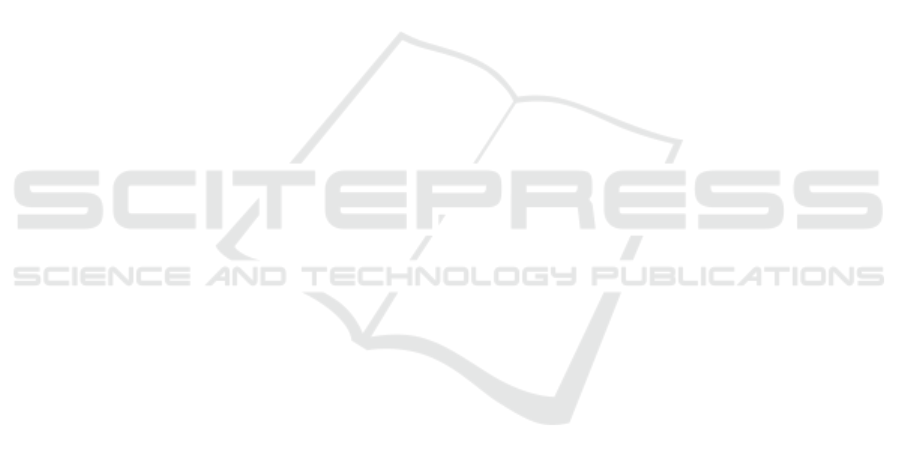
results are shown in Table 3. Notice that we can-
not show any improvement in the performance for
the GHMM while the performance of the GGHMM
is similarly good compared to the 3 dimensional case.
Thus, we conclude that the dimensions of the obser-
vation space does not have a significant effect on the
performance for these realizations.
Overall, we are able to show that the GGHMM
performs better than the GHMM under specific cir-
cumstances. While both models have their advan-
tages, there may be some benefit in using a GGHMM
for the identification of states of a system with ex-
tereme deviations over the GHMM. On the other
hand, the increased training time for the GGHMM
also needs to be considered when deploying such a
model in production.
6 CONCLUSION
In this work, we have introduced an extension to the
hidden Markov model in order to identify the states
of a non-stationary dynamical system with observa-
tions that have heavy-tailed distributions. Such sys-
tems pose a significant challenge to researchers in
computational fields and even incremental advance-
ments may be highly lucrative.
Our proposed model, the Gaussian-Gamma hid-
den Markov model, can be considered as a variant
of the hidden Markov model where we increase the
complexity of the model in order to accommodate our
prior knowledge on heavy-tailed distributions. The
increased complexity of our model can be efficiently
handled by formulating the observation density as an
exponential family. Our model can potentially be
used to model various non-stationary dynamic sys-
tems with multiple regimes and heavy-tailed distri-
butions and is capable of representing heteroscedastic
processes within each state and is highly flexible. Fur-
thermore, the model is mostly analytically tractable
aside from requiring an auxiliary root finding algo-
rithm. This allows it to be trained relatively quickly
compared to the state-of-the-art deep neural networks
and requires much less data in order to be trained.
We have shown the application of GGHMM in
state identification for synthetically generated data.
Results show that the GGHMM has a performance
comparable with the GHMM in the state identifica-
tion task. In terms of improvements to the GGHMM,
one obvious way of improvement can come from ex-
plicitly modelling the dependencies between the se-
quential latent scale variables. However, since this
would introduce intractable calculations we have left
it as a future research.
Another source of improvement may be to also
learn the number latent states in the system. Such
models use the hierarchical Dirichlet process as their
latent representation of the state transition system
(Teh et al., 2006). For an unknown number of states,
the intuition that the states are persistent can be mod-
elled using the work in (Fox et al., 2007). For more
efficient learning in such models, practical considera-
tions are presented in (Ulker et al., 2011).
REFERENCES
Bilmes, J. (2000). A gentle tutorial of the em algorithm
and its application to parameter estimation for gaus-
sian mixture and hidden markov models. Technical
Report ICSI-TR-97-021, University of Berkeley, 4.
Bishop, C. M. (2006). Pattern Recognition and Ma-
chine Learning (Information Science and Statistics).
Springer-Verlag, Berlin, Heidelberg.
Bulla, J. (2011). Hidden markov models with t components.
increased persistence and other aspects. Quantitative
Finance, 11(3):459–475.
Cemgil, A. T., Fevotte, C., and Godsill, S. J. (2007). Vari-
ational and stochastic inference for bayesian source
separation. Digital Signal Processing, 17(5):891 –
913. Special Issue on Bayesian Source Separation.
Chatzis, S., Kosmopoulos, D., and Varvarigou, T. (2009).
Robust sequential data modeling using an outlier tol-
erant hidden markov model. IEEE transactions on
pattern analysis and machine intelligence, 31:1657–
69.
Fox, E., Sudderth, E., Jordan, M., and Willsky (2007).
The sticky hdp-hmm: Bayesian nonparametric hidden
markov models with persistent states. Technical Re-
port 2, MIT Laboratory for Information & Decision
Systems, Cambridge, MA 02139.
Rabiner, L. R. (1990). A Tutorial on Hidden Markov Mod-
els and Selected Applications in Speech Recognition,
page 267–296. Morgan Kaufmann Publishers Inc.,
San Francisco, CA, USA.
Shoham, S. (2002). Robust clustering by determinis-
tic agglomeration em of mixtures of multivariate t-
distributions. Pattern Recognition, 35:1127–1142.
Teh, Y. W., Jordan, M. I., Beal, M. J., and Blei, D. M.
(2006). Hierarchical dirichlet processes. Journal of
the American Statistical Association, 101(476):1566–
1581.
Ulker, Y., Gunsel, B., and Cemgil, A. T. (2011). An-
nealed smc samplers for nonparametric bayesian mix-
ture models. IEEE Signal Processing Letters, 18(1):3–
6.
Xu, L. and Jordan, M. I. (1996). On convergence properties
of the em algorithm for gaussian mixtures. Neural
Comput., 8(1):129–151.
Zhang, H., Jonathan Wu, Q. M., and Nguyen, T. M. (2013).
Modified student’s t-hidden markov model for pattern
recognition and classification. IET Signal Processing,
7(3):219–227.
ICPRAM 2021 - 10th International Conference on Pattern Recognition Applications and Methods
142