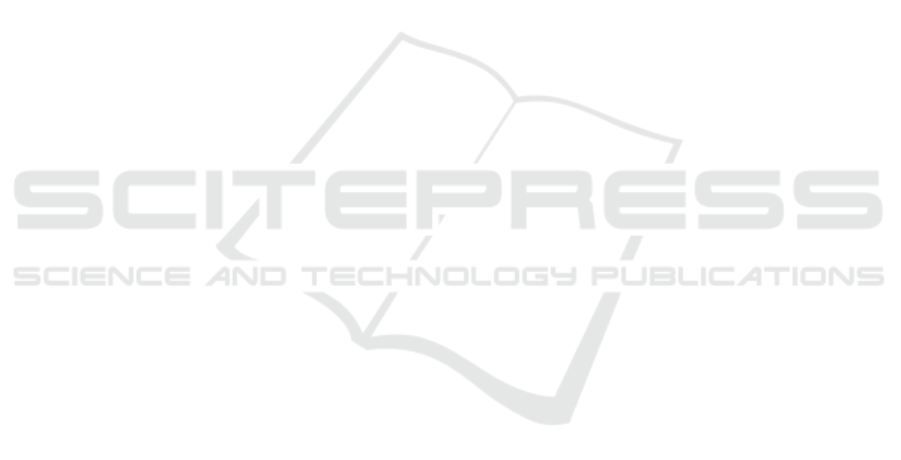
REFERENCES
Carreira, J. and Zisserman, A. (2017). Quo vadis, action
recognition? a new model and the kinetics dataset. In
2017 IEEE Conference on Computer Vision and Pat-
tern Recognition (CVPR), pages 4724–4733.
Cho, K., van Merri
¨
enboer, B., Gulcehre, C., Bahdanau, D.,
Bougares, F., Schwenk, H., and Bengio, Y. (2014).
Learning phrase representations using RNN encoder–
decoder for statistical machine translation. In Pro-
ceedings of the 2014 Conference on Empirical Meth-
ods in Natural Language Processing (EMNLP), pages
1724–1734.
Donahue, J., Hendricks, L. A., Rohrbach, M., Venugopalan,
S., Guadarrama, S., Saenko, K., and Darrell, T.
(2017). Long-term recurrent convolutional networks
for visual recognition and description. IEEE Trans.
Pattern Anal. Mach. Intell., page 677–691.
Dong, Y., Hu, Z., Uchimura, K., and Murayama, N. (2011).
Driver inattention monitoring system for intelligent
vehicles: A review. IEEE Transactions on Intelligent
Transportation Systems, 12(2):596–614.
Farneb
¨
ack, G. (2003). Two-frame motion estimation based
on polynomial expansion. In Proceedings of the 13th
Scandinavian Conference on Image Analysis, page
363–370. Springer-Verlag.
Glorot, X. and Bengio, Y. (2010). Understanding the diffi-
culty of training deep feedforward neural networks. In
In Proceedings of the International Conference on Ar-
tificial Intelligence and Statistics (AISTATS’10). Soci-
ety for Artificial Intelligence and Statistics.
He, K., Zhang, X., Ren, S., and Sun, J. (2015). Delving deep
into rectifiers: Surpassing human-level performance
on imagenet classification. In Proceedings of the 2015
IEEE International Conference on Computer Vision
(ICCV), page 1026–1034.
Hochreiter, S. and Schmidhuber, J. (1997). Long short-term
memory. Neural computation, 9:1735–80.
Jaeger, H. (2002). Tutorial on training recurrent neural net-
works, covering bppt, rtrl, ekf and the echo state net-
work approach. GMD-Forschungszentrum Informa-
tionstechnik, 2002., 5.
Krizhevsky, A., Sutskever, I., and Hinton, G. E. (2012). Im-
agenet classification with deep convolutional neural
networks. In Advances in Neural Information Pro-
cessing Systems 25, pages 1097–1105. Curran Asso-
ciates, Inc.
Lin, J., Gan, C., and Han, S. (2019). Tsm: Temporal shift
module for efficient video understanding. In Proceed-
ings of the IEEE International Conference on Com-
puter Vision, pages 7083–7093.
Liu, J., Shahroudy, A., Perez, M., Wang, G., Duan, L.-
Y., and Kot, A. C. (2019). Ntu rgb+d 120: A large-
scale benchmark for 3d human activity understanding.
IEEE Transactions on Pattern Analysis and Machine
Intelligence.
Loshchilov, I. and Hutter, F. (2019). Decoupled weight
decay regularization. In International Conference on
Learning Representations.
Mehri, S., Kumar, K., Gulrajani, I., Kumar, R., Jain, S.,
Sotelo, J., Courville, A., and Bengio, Y. (2016). Sam-
plernn: An unconditional end-to-end neural audio
generation model.
Mohajerin, N. and Waslander, S. L. (2017). State initializa-
tion for recurrent neural network modeling of time-
series data. In 2017 International Joint Conference on
Neural Networks (IJCNN), pages 2330–2337.
Molchanov, P., Yang, X., Gupta, S., Kim, K., Tyree, S.,
and Kautz, J. (2016). Online detection and classifica-
tion of dynamic hand gestures with recurrent 3d con-
volutional neural networks. 2016 IEEE Conference
on Computer Vision and Pattern Recognition (CVPR),
pages 4207–4215.
Ohn-Bar, E., Martin, S., Tawari, A., and Trivedi, M. M.
(2014). Head, eye, and hand patterns for driver activ-
ity recognition. In 2014 22nd International Confer-
ence on Pattern Recognition, pages 660–665.
Pickering, C. A. (2005). The search for a safer driver inter-
face: a review of gesture recognition human machine
interface. Computing Control Engineering Journal,
16(1):34–40.
Simonyan, K. and Zisserman, A. (2014). Two-stream con-
volutional networks for action recognition in videos.
In Advances in Neural Information Processing Sys-
tems 27. Curran Associates, Inc.
Wang, L., Xiong, Y., Wang, Z., Qiao, Y., Lin, D., Tang,
X., and Van Gool, L. (2019). Temporal segment net-
works for action recognition in videos. IEEE Trans-
actions on Pattern Analysis and Machine Intelligence,
41:2740–2755.
Werbos, P. (1990). Backpropagation through time: what
does it do and how to do it. In Proceedings of IEEE,
volume 78, pages 1550–1560.
Weyers, P., Barth, A., and Kummert, A. (2018). Driver state
monitoring with hierarchical classification. In 2018
21st International Conference on Intelligent Trans-
portation Systems (ITSC), pages 3239–3244.
Weyers, P., Schiebener, D., and Kummert, A. (2019). Ac-
tion and object interaction recognition for driver ac-
tivity classification. In 2019 IEEE Intelligent Trans-
portation Systems Conference (ITSC), pages 4336–
4341.
Yan, C., Coenen, F., and Zhang, B. (2016). Driving posture
recognition by convolutional neural networks. IET
Computer Vision, 10(2):103–114.
Zaremba, W., Sutskever, I., and Vinyals, O. (2014). Recur-
rent neural network regularization.
Zolfaghari, M., Singh, K., and Brox, T. (2018). Eco: Ef-
ficient convolutional network for online video under-
standing. In The European Conference on Computer
Vision (ECCV).
Continuous Driver Activity Recognition from Short Isolated Action Sequences
165