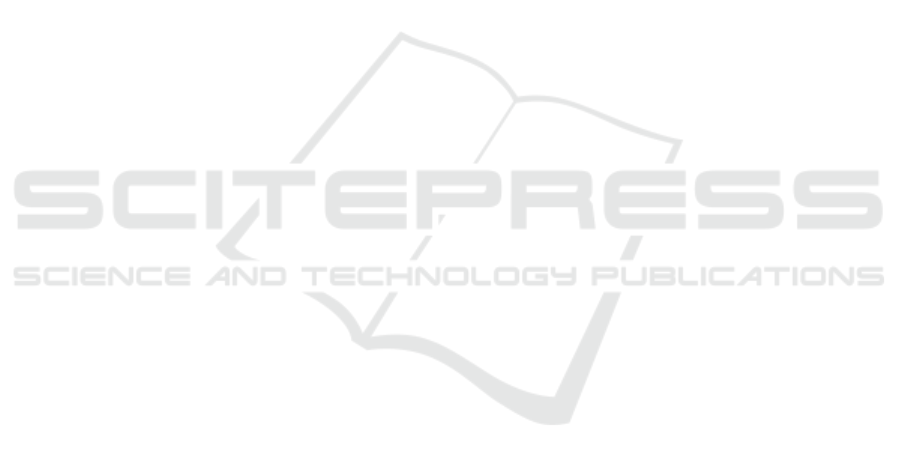
tween 75 and 100 km, and more than 100 km. Al-
most 60% from all selected postal codes were less
than 50 km from the nearest recruiting centre. The
number of postal codes in each bin per PRIZM seg-
ment is presented in Table 6. This allows an addi-
tional tool for the marketers to focus on the recruiting
strategy based on the distance.
7 CONCLUSIONS
In the absence of the individual data for the potential
applicants, we explored the problem of identifying
geographical areas with high potential for recruiting
women by using demographic, life style, consumer
behaviour, settlement patterns and social media char-
acteristics of the neighbourhood (postal code) of the
potential applicants. Using historical women recruit-
ing data and two Environics datasets (DemoStats and
PRIZM), a selection of 19,884 postal codes with high-
est recruiting potential was obtained from the most
promising 10% of all Canadian postal codes, obtained
using a logistic regression model. Finally, the selec-
tion was segmented to derive the optimum marketing
channels and messages by using two approaches: (i)
clustering based on the aggregated social media be-
haviour of the postal code and (ii) binning using the
distance to the nearest recruiting centre. The social
media behaviour was retrieved from a third Environ-
ics dataset, Optiks Social. The approach presented in
this paper can be applied frequently for a long term,
contributing to the commitment to increase women
representation in CAF.
REFERENCES
Ayzenshtat, L., Rajamani, K., Vishnevskiy, A., Georgiev,
N., and Preotescu, M. (2019). Methods and appara-
tus to identify affinity between segment attributes and
product characteristics. US PATENT 0073685 A1.
Boone, C., Cadenasso, M., Grove, J., Schwarz, K., and
Buckley, G. (2010). Landscape, vegetation charac-
teristics, and group identity in an urban and suburban
watershed: Why the 60s matter. Urban Ecosystems,
13(3):255–271.
Buttrey, S. E., Whitaker, L. R., and Alt, J. K. (2018). De-
velopments in the statistical modeling of military re-
cruiting. CHANCE, 31(2):38–44.
Clingan, M. (2007). U.s. army custom segmentation sys-
tem. Presentation at 75th Military Operations Re-
search Society Symposium, June 12-14, Annapolis,
MD.
Dempster, A. P., Laird, N. M., and Rubin, D. B. (1977).
Maximum likelihood from incomplete data via the em
algorithm. Journal of the Royal Statistical Society, Se-
ries B, 39(1):1–38.
Department of National Defence (2017). Strong, secure,
engaged: Canada’s defence policy.
Department of National Defence (2018). Annual Report on
Regular Force Personnel:2015-2016. Internal Refer-
ence Document: DRDC-RDDC-2018-D086.
Environics Analytics (2018). Demostats database.
https://www.environicsanalytics.com/en-ca/data/
demographic/demostats. Accessed Feb. 20, 2019.
Fulton, B. M. (2016). Determining Market Categorization
of United States Zip Codes for Purposes of Army Re-
cruiting. Master’s thesis, Naval Postgraduate School,
Monterey, CA.
Harnett, D. L. (1975). Introduction to statistical methods.
Addison-Wesley Pub. Co.
Heitgerd, J. L. (2001). Using gis and demographics to char-
acterize communities at risk: A model for atsdr. Jour-
nal of Environmental health, 64(5):21–30.
Monaghan, E. M. (2016). Estimating the depth of the navy
recruiting market. Master’s thesis, Naval Postgraduate
School, Monterey, CA.
Nielsen Company (2020). My best segments. https://
claritas360.claritas.com/mybestsegments/. Accessed
Jan. 31, 2020.
Pedregosa, F., Varoquaux, G., Gramfort, A., Michel, V.,
Thirion, B., Grisel, O., Blondel, M., Prettenhofer,
P., Weiss, R., Dubourg, V., Vanderplas, J., Passos,
A., Cournapeau, D., Brucher, M., Perrot, M., and
Duchesnay, E. (2011). Scikit-learn: Machine learning
in Python. Journal of Machine Learning Research,
12:2825–2830.
Ueno, R., Bryce, R., and Calitoiu, D. (2019). Ranking Clus-
ters of Postal Codes to Improve Recruitment in the
Canadian Armed Forces. In Proceedings of the 18th
IEEE International Conference on Machine Learning
and Application, volume 1, pages 1192 – 1197. Boca
Raton, FL, USA.
Ward, J. H. (1963). Hierarchical grouping to optimize an
objective function. Journal of the American Statistical
Association, 58(301):236–244.
ICORES 2021 - 10th International Conference on Operations Research and Enterprise Systems
314