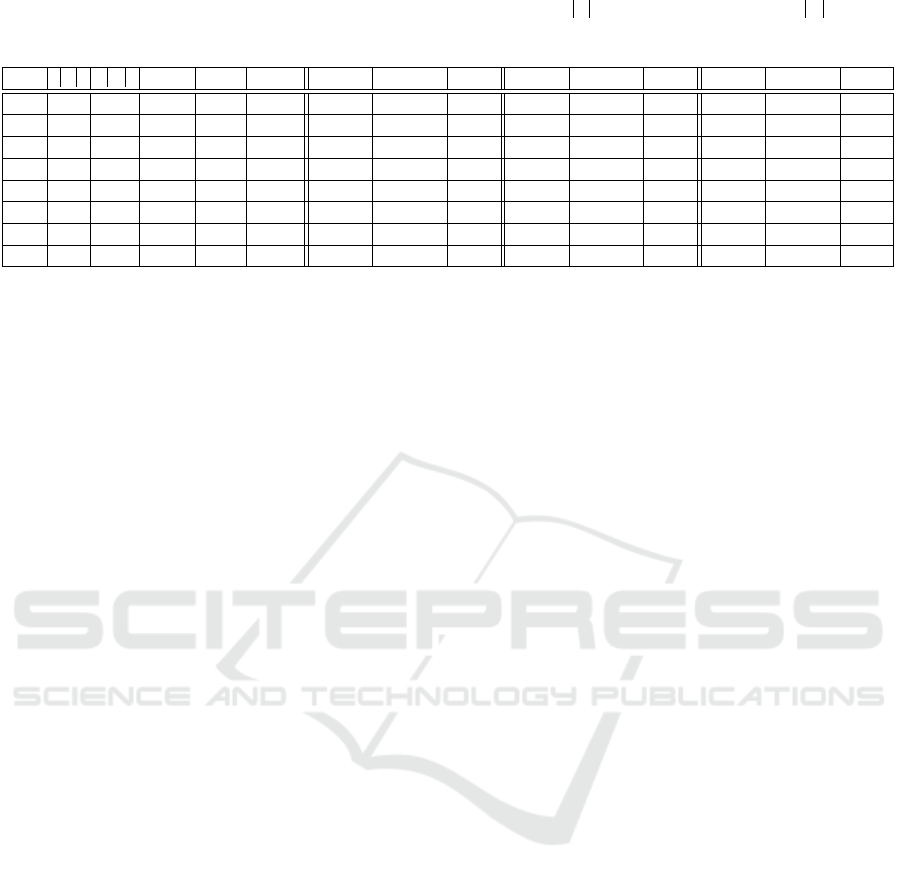
Table 2: Performance of the baseline algorithm on generated ground truth data. P – length of person index list, T – number
of generated short texts, Max – maximum number of mentioned persons per text, MN – number of persons with middle names
and Amb – degree of ambiguity. Precision (prec), recall and f-score (f ) are calculated based on the relations P, R and A.
Nr. P T Max MN Amb prec
P
recall
P
f
P
prec
R
recall
R
f
R
prec
A
recall
A
f
A
1 1 10 0 0 0 1.00 1.00 1.00 1.00 1.00 1.00 - - -
2 1 200 0 0 0 0.14 1.00 0.25 0.00 0.00 - - - -
3 20 200 0 0 0 0.63 0.85 0.72 0.82 0.72 0.77 - - -
4 20 200 10 0 0 0.38 0.90 0.54 0.61 0.09 0.16 - - -
5 20 200 10 4 0 0.31 0.80 0.45 0.63 0.09 0.16 - - -
6 20 200 10 4 2 0.39 0.75 0.52 0.47 0.41 0.44 0.03 1.00 0.06
7 20 200 10 4 3 0.45 0.85 0.59 0.59 0.54 0.56 0.05 0.95 0.09
8 40 300 10 4 3 0.39 0.75 0.52 0.53 0.49 0.51 0.03 0.87 0.06
evaluation, several measurements were defined to ex-
amine the performance of potential solutions. With
this, we analyzed our approach and suggested further
potentials for improvement for future approaches.
For future work, we plan to examine performance
in real use cases using data of our industrial scenar-
ios. In case of ambiguities, our goal is to efficiently
integrate human experts which are able to contribute
with their knowledge. The challenge itself can be
made more difficult by generating names with differ-
ent cases (i.e. lower case, upper case, mixed case,
camel case, etc). Regarding the domain, we aim to
generalize the problem statement to other entity types
which have multiple names or IDs in different forms.
ACKNOWLEDGEMENTS
This work was funded by the BMBF project SensAI
(grant no. 01IW20007).
REFERENCES
Cohen, A. (2005). Unsupervised gene/protein named en-
tity normalization using automatically extracted dic-
tionaries. In Proceedings of the ACL-ISMB Work-
shop on Linking Biological Literature, Ontologies
and Databases: Mining Biological Semantics@ISMB
2005, Detroit, MI, USA June 24, 2005, pages 17–24.
Association for Computational Linguistics.
Ek, T., Kirkegaard, C., Jonsson, H., and Nugues, P. (2011).
Named entity recognition for short text messages.
Procedia-Social and Behavioral Sciences, 27:178–
187.
Hua, W., Wang, Z., Wang, H., Zheng, K., and Zhou, X.
(2015). Short text understanding through lexical-
semantic analysis. In 2015 IEEE 31st Int’l Conf. on
Data Engineering, pages 495–506.
Jacob, F., Javed, F., Zhao, M., and McNair, M. (2014).
scool: A system for academic institution name nor-
malization. In 2014 Int. Conf. on Collaboration Tech-
nologies and Systems, CTS 2014, Minneapolis, MN,
USA, May 19-23, 2014, pages 86–93. IEEE.
Jijkoun, V., Khalid, M. A., Marx, M., and de Rijke, M.
(2008). Named entity normalization in user gener-
ated content. In Proceedings of the Second Workshop
on Analytics for Noisy Unstructured Text Data, AND
2008, Singapore, July 24, 2008, volume 303 of ACM
Int. Conf. Proceeding Series, pages 23–30. ACM.
Jilek, C., Schr
¨
oder, M., Novik, R., Schwarz, S., Maus, H.,
and Dengel, A. (2019). Inflection-tolerant ontology-
based named entity recognition for real-time appli-
cations. In 2nd Conference on Language, Data and
Knowledge, LDK 2019, May 20-23, 2019, Leipzig,
Germany, volume 70 of OASICS, pages 11:1–11:14.
Schloss Dagstuhl - Leibniz-Zentrum f
¨
ur Informatik.
Khalid, M. A., Jijkoun, V., and de Rijke, M. (2008). The
impact of named entity normalization on information
retrieval for question answering. In Advances in In-
formation Retrieval , 30th European Conference on IR
Research, ECIR 2008, Glasgow, UK, March 30-April
3, 2008. Proceedings, volume 4956 of Lecture Notes
in Computer Science, pages 705–710. Springer.
Liu, X., Zhou, M., Zhou, X., Fu, Z., and Wei, F. (2012).
Joint inference of named entity recognition and nor-
malization for tweets. In The 50th Annual Meeting of
the Association for Computational Linguistics, Pro-
ceedings of the Conference, July 8-14, 2012, Jeju Is-
land, Korea - Volume 1: Long Papers, pages 526–535.
The Association for Computer Linguistics.
Mart
´
ınez-Rodr
´
ıguez, J., Hogan, A., and L
´
opez-Ar
´
evalo, I.
(2020). Information extraction meets the semantic
web: A survey. Semantic Web, 11(2):255–335.
Nadeau, D. and Sekine, S. (2007). A survey of named entity
recognition and classification. Lingvisticae Investiga-
tiones, 30.
Rizzo, G., Pereira, B., Varga, A., van Erp, M., and Basave,
A. E. C. (2017). Lessons learnt from the named en-
tity recognition and linking (NEEL) challenge series.
Semantic Web, 8(5):667–700.
Song, Y., Wang, H., Wang, Z., Li, H., and Chen, W.
(2011). Short text conceptualization using a proba-
bilistic knowledgebase. In IJCAI 2011, Proceedings
of the 22nd Int. Joint Conference on Artificial Intelli-
gence, Barcelona, Catalonia, Spain, July 16-22, 2011,
pages 2330–2336. IJCAI/AAAI.
The Person Index Challenge: Extraction of Persons from Messy, Short Texts
537