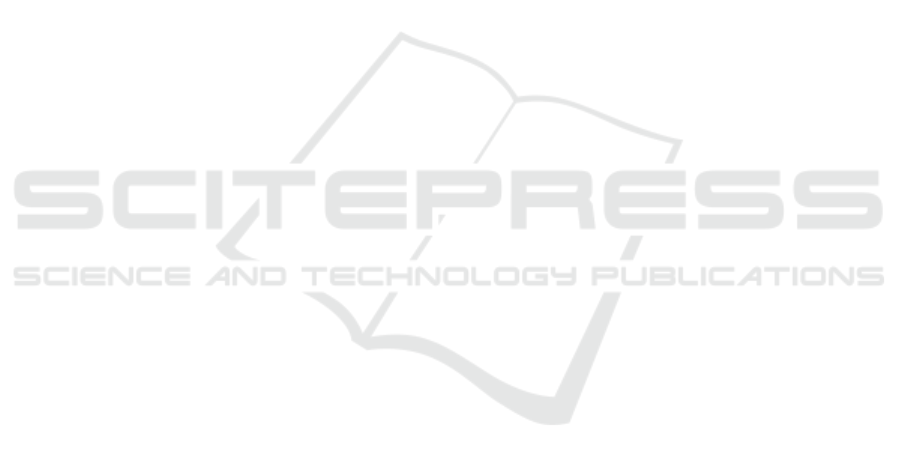
Devlin, S. and Kudenko, D. (2012). Dynamic potential-
based reward shaping. In Proceedings of the 11th
International Conference on Autonomous Agents and
Multiagent Systems - Volume 1, AAMAS ’12, pages
433–440. International Foundation for Autonomous
Agents and Multiagent Systems.
Devlin, S., Yliniemi, L., Kudenko, D., and Tumer, K.
(2014). Potential-based difference rewards for mul-
tiagent reinforcement learning. In Proceedings of
the 2014 International Conference on Autonomous
Agents and Multi-agent Systems, AAMAS ’14, pages
165–172. International Foundation for Autonomous
Agents and Multiagent Systems.
Foerster, J. N., Farquhar, G., Afouras, T., Nardelli, N., and
Whiteson, S. (2018). Counterfactual multi-agent pol-
icy gradients. In Thirty-Second AAAI Conference on
Artificial Intelligence.
Garc
´
ıa, J., Fern, and o Fern
´
andez (2015). A comprehen-
sive survey on safe reinforcement learning. Journal of
Machine Learning Research, 16(42):1437–1480.
Grze
´
s, M. (2017). Reward shaping in episodic reinforce-
ment learning. In Proceedings of the 16th Conference
on Autonomous Agents and MultiAgent Systems, AA-
MAS ’17, pages 565–573. International Foundation
for Autonomous Agents and Multiagent Systems.
Gupta, J. K., Egorov, M., and Kochenderfer, M. (2017).
Cooperative multi-agent control using deep reinforce-
ment learning. In International Conference on Au-
tonomous Agents and Multiagent Systems, pages 66–
83. Springer.
Jaderberg, M., Czarnecki, W. M., Dunning, I., Marris, L.,
Lever, G., Casta
˜
neda, A. G., Beattie, C., Rabinowitz,
N. C., Morcos, A. S., Ruderman, A., Sonnerat, N.,
Green, T., Deason, L., Leibo, J. Z., Silver, D., Has-
sabis, D., Kavukcuoglu, K., and Graepel, T. (2019).
Human-level performance in 3d multiplayer games
with population-based reinforcement learning. Sci-
ence, 364(6443):859–865.
Laurent, G. J., Matignon, L., Fort-Piat, L., et al. (2011).
The world of Independent Learners is not Marko-
vian. Journal of Knowledge-based and Intelligent En-
gineering Systems.
Leibo, J. Z., Zambaldi, V., Lanctot, M., Marecki, J.,
and Graepel, T. (2017). Multi-Agent Reinforcement
Learning in Sequential Social Dilemmas. In Proceed-
ings of the 16th Conference on Autonomous Agents
and Multiagent Systems, pages 464–473. IFAAMAS.
Leike, J., Martic, M., Krakovna, V., Ortega, P. A., Everitt,
T., Lefrancq, A., Orseau, L., and Legg, S. (2017). AI
safety gridworlds. CoRR, abs/1711.09883.
Liu, S., Lever, G., Merel, J., Tunyasuvunakool, S., Heess,
N., and Graepel, T. (2019). Emergent coordination
through competition. In 7th International Conference
on Learning Representations, ICLR 2019, New Or-
leans, LA, USA.
Lowd, D. and Meek, C. (2005). Adversarial learning. In
Proceedings of the eleventh ACM SIGKDD interna-
tional conference on Knowledge discovery in data
mining, pages 641–647. ACM.
Mnih, V., Kavukcuoglu, K., Silver, D., Rusu, A. A., Veness,
J., Bellemare, M. G., Graves, A., Riedmiller, M., Fid-
jeland, A. K., Ostrovski, G., et al. (2015). Human-
Level Control through Deep Reinforcement Learning.
Nature.
Ng, A. Y., Harada, D., and Russell, S. J. (1999). Pol-
icy invariance under reward transformations: Theory
and application to reward shaping. In Proceedings
of the Sixteenth International Conference on Machine
Learning, ICML ’99, pages 278–287. Morgan Kauf-
mann Publishers Inc.
Phan, T., Belzner, L., Gabor, T., and Schmid, K. (2018).
Leveraging statistical multi-agent online planning
with emergent value function approximation. In Pro-
ceedings of the 17th International Conference on Au-
tonomous Agents and MultiAgent Systems, AAMAS,
pages 730–738.
Rashid, T., Samvelyan, M., de Witt, C. S., Farquhar, G., Fo-
erster, J., and Whiteson, S. (2018). QMIX: Monotonic
Value Function Factorisation for Deep Multi-Agent
Reinforcement Learning. In International Conference
on Machine Learning, pages 4292–4301.
Seurin, M., Preux, P., and Pietquin, O. (2019). ”i’m sorry
dave, i’m afraid i can’t do that” deep q-learning from
forbidden action. CoRR, abs/1910.02078.
Silver, D., Schrittwieser, J., Simonyan, K., Antonoglou, I.,
Huang, A., Guez, A., Hubert, T., Baker, L., Lai, M.,
Bolton, A., et al. (2017). Mastering the Game of Go
without Human Knowledge. Nature, 550(7676):354.
Son, K., Kim, D., Kang, W. J., Hostallero, D. E., and Yi, Y.
(2019). QTRAN: Learning to Factorize with Transfor-
mation for Cooperative Multi-Agent Reinforcement
Learning. In International Conference on Machine
Learning, pages 5887–5896.
Sunehag, P., Lever, G., Gruslys, A., Czarnecki, W. M.,
Zambaldi, V., Jaderberg, M., Lanctot, M., Sonnerat,
N., Leibo, J. Z., Tuyls, K., et al. (2018). Value-
decomposition networks for cooperative multi-agent
learning based on team reward. In Proceedings of the
17th International Conference on Autonomous Agents
and Multiagent Systems (Extended Abstract), pages
2085–2087. IFAAMAS.
Sutton, R. S. and Barto, A. G. (2018). Reinforcement Learn-
ing: An Introduction. A Bradford Book, Cambridge,
MA, USA.
Tampuu, A., Matiisen, T., Kodelja, D., Kuzovkin, I., Korjus,
K., Aru, J., Aru, J., and Vicente, R. (2017). Multia-
gent Cooperation and Competition with Deep Rein-
forcement Learning. PloS one, 12(4):e0172395.
Wang, S., Wan, J., Zhang, D., Li, D., and Zhang, C.
(2016). Towards smart factory for industry 4.0: a
self-organized multi-agent system with big data based
feedback and coordination. Computer Networks,
101:158–168.
Wolpert, D. H. and Tumer, K. (2002). Optimal payoff func-
tions for members of collectives. In Modeling com-
plexity in economic and social systems, pages 355–
369. World Scientific.
Zahavy, T., Haroush, M., Merlis, N., Mankowitz, D. J.,
and Mannor, S. (2018). Learn what not to learn: Ac-
tion elimination with deep reinforcement learning. In
Bengio, S., Wallach, H., Larochelle, H., Grauman, K.,
Cesa-Bianchi, N., and Garnett, R., editors, Advances
in Neural Information Processing Systems 31, pages
3562–3573. Curran Associates, Inc.
SAT-MARL: Specification Aware Training in Multi-Agent Reinforcement Learning
37