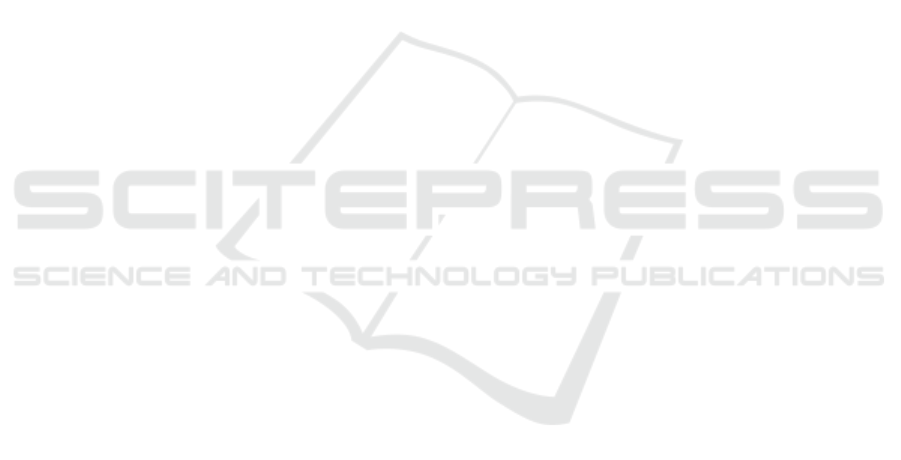
mance in instance segmentation. The experiments
show, that not only our accuracy in separating ob-
jects is higher than comparable fast approaches, but
we are able to match most ground truth instances
with a significant overlap of the ground truth. This
is particularly important in the context of driver as-
sistance systems, since a missed instance is a bigger
problem than an object that was not matched with all
Lidar points. We have also illustrated, that the pro-
posed MCs improve the results of our algorithm and
help to find otherwise missed objects. The further
use of segmented point clouds for classification and
to remove false positives, is outside the scope of this
work. However, this application has previously been
researched by (Hahn et al., 2020) and shows promis-
ing results.
REFERENCES
AbuBaker, A., Qahwaji, R., Ipson, S., and Saleh, M. (2007).
One scan connected component labeling technique.
In 2007 IEEE International Conference on Signal
Processing and Communications, pages 1283–1286.
IEEE.
Allegretti, S., Bolelli, F., Cancilla, M., and Grana, C.
(2019). A block-based union-find algorithm to la-
bel connected components on gpus. In International
Conference on Image Analysis and Processing, pages
271–281. Springer.
Ankerst, M., Breunig, M. M., Kriegel, H.-P., and Sander, J.
(1999). Optics: ordering points to identify the cluster-
ing structure. ACM Sigmod record, 28(2):49–60.
Behley, J., Garbade, M., Milioto, A., Quenzel, J., Behnke,
S., Stachniss, C., and Gall, J. (2019). Semantickitti:
A dataset for semantic scene understanding of lidar
sequences. In Proceedings of the IEEE International
Conference on Computer Vision, pages 9297–9307.
Bogoslavskyi, I. and Stachniss, C. (2016). Fast range
image-based segmentation of sparse 3d laser scans
for online operation. In 2016 IEEE/RSJ International
Conference on Intelligent Robots and Systems (IROS),
pages 163–169. IEEE.
Cheng, H., Li, Y., and Sester, M. (2019). Pedestrian group
detection in shared space. In 2019 IEEE Intelligent
Vehicles Symposium (IV), pages 1707–1714. IEEE.
Chu, P., Cho, S., Sim, S., Kwak, K., and Cho, K. (2017). A
fast ground segmentation method for 3d point cloud.
Journal of information processing systems, 13(3).
Comaniciu, D. and Meer, P. (2002). Mean shift: A robust
approach toward feature space analysis. IEEE Trans-
actions on pattern analysis and machine intelligence,
24(5):603–619.
Cortinhal, T., Tzelepis, G., and Aksoy, E. E. (2020). Sal-
sanext: Fast semantic segmentation of lidar point
clouds for autonomous driving. arXiv preprint
arXiv:2003.03653.
Ester, M., Kriegel, H.-P., Sander, J., Xu, X., et al. (1996).
A density-based algorithm for discovering clusters in
large spatial databases with noise. In Kdd, volume 96,
pages 226–231.
Fukunaga, K. and Hostetler, L. (1975). The estimation of
the gradient of a density function, with applications in
pattern recognition. IEEE Transactions on informa-
tion theory, 21(1):32–40.
Geiger, A., Lenz, P., and Urtasun, R. (2012). Are we ready
for autonomous driving? the kitti vision benchmark
suite. In 2012 IEEE Conference on Computer Vision
and Pattern Recognition, pages 3354–3361. IEEE.
Gerdzhev, M., Razani, R., Taghavi, E., and Liu, B. (2020).
Tornado-net: multiview total variation semantic seg-
mentation with diamond inception module. arXiv
preprint arXiv:2008.10544.
Hahn, L., Hasecke, F., and Kummert, A. (2020). Fast ob-
ject classification and meaningful data representation
of segmented lidar instances. 23rd IEEE Interna-
tional Conference on Intelligent Transportation Sys-
tems (ITSC).
Hennequin, A., Lacassagne, L., Cabaret, L., and Meunier,
Q. (2018). A new direct connected component label-
ing and analysis algorithms for gpus. In 2018 Con-
ference on Design and Architectures for Signal and
Image Processing (DASIP), pages 76–81. IEEE.
Himmelsbach, M., Hundelshausen, F. V., and Wuensche,
H.-J. (2010). Fast segmentation of 3d point clouds for
ground vehicles. In 2010 IEEE Intelligent Vehicles
Symposium, pages 560–565. IEEE.
Hoshen, J. and Kopelman, R. (1976). Percolation and clus-
ter distribution. i. cluster multiple labeling technique
and critical concentration algorithm. Physical Review
B, 14(8):3438.
Kochanov, D., Nejadasl, F. K., and Booij, O. (2020). Kpr-
net: Improving projection-based lidar semantic seg-
mentation. arXiv preprint arXiv:2007.12668.
Korchev, D., Cheng, S., Owechko, Y., et al. (2013). On
real-time lidar data segmentation and classification. In
Proceedings of the International Conference on Im-
age Processing, Computer Vision, and Pattern Recog-
nition (IPCV), page 1. The Steering Committee of The
World Congress in Computer Science, Computer . . . .
Lahoud, J., Ghanem, B., Pollefeys, M., and Oswald, M. R.
(2019). 3d instance segmentation via multi-task met-
ric learning. In Proceedings of the IEEE International
Conference on Computer Vision, pages 9256–9266.
Mao, J., Xu, G., Li, W., Fan, X., and Luo, J. (2020).
Pedestrian detection and recognition using lidar for
autonomous driving. In 2019 International Confer-
ence on Optical Instruments and Technology: Opti-
cal Sensors and Applications, volume 11436, page
114360R. International Society for Optics and Pho-
tonics.
Milioto, A., Behley, J., McCool, C., and Stachniss, C.
(2020). LiDAR Panoptic Segmentation for Au-
tonomous Driving.
Moosmann, F. (2013). Interlacing self-localization, mov-
ing object tracking and mapping for 3d range sensors,
volume 24. KIT Scientific Publishing.
ICPRAM 2021 - 10th International Conference on Pattern Recognition Applications and Methods
34