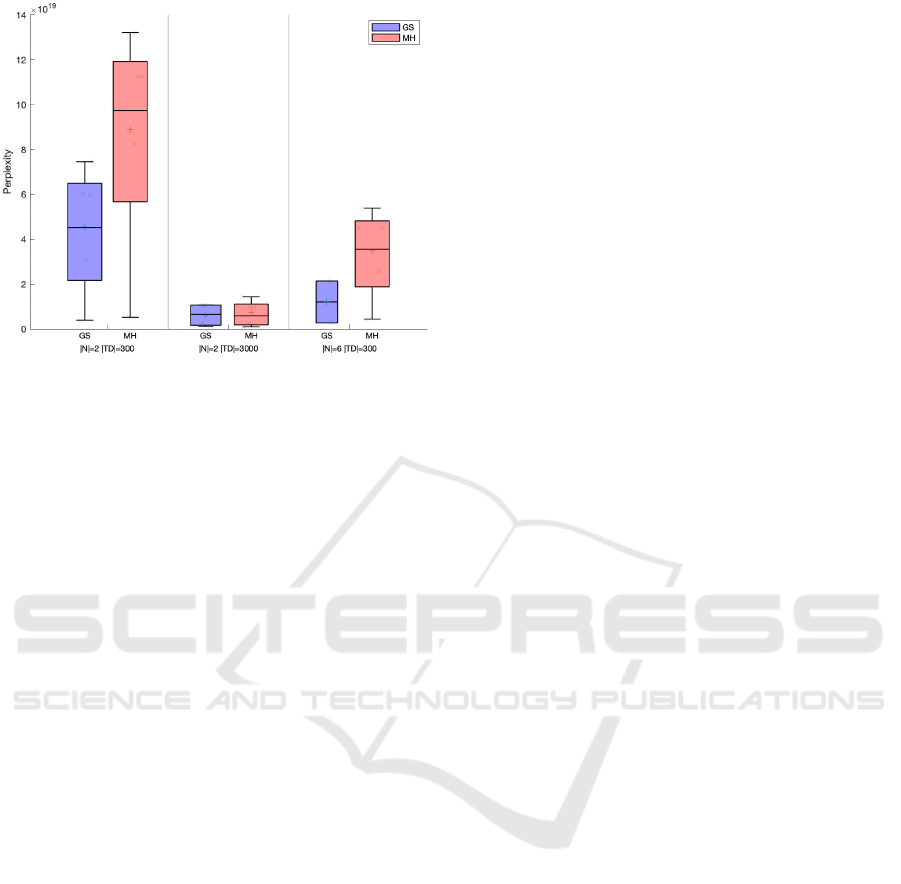
Figure 4: Perplexity Values for the GS and MH algorithms
inferring P(1, 0)CSGs.
models to perform machine learning tasks related to
chord sequences like style classification or the gener-
ation of musical phrases. The data suggest we could
improve our results by either investing in enough
computational power or developing cheaper algo-
rithms to infer grammars with k > 1 and l > 0.
ACKNOWLEDGMENTS
This work has been supported by the Brazilian Agen-
cies State of Minas Gerais Research Foundation –
FAPEMIG (APQ-00040-14); Coordination for the
Improvement of Higher Level Personnel – CAPES;
and National Council of Scientific and Technological
Development – CNPq (402956/2016-8).
REFERENCES
Bhattacharjee, A. and Srinivasan, N. (2011). Hindustani
raga representation and identification: A transition
probability based approach. International Journal of
Mind, Brain and Cognition, 2:71–99.
Blaß, M. (2013). Timbre-based drum pattern classification
using hidden markov models. In Machine Learning
and Knowledge Discovery in Databases.
Breuker, D., Matzner, M., Delfmann, P., and Becker, J.
(2016). Comprehensible predictive models for busi-
ness processes. MIS Q., 40(4):1009–1034.
Burgoyne, J., Wild, J., and Fujinaga, I. (2011). An expert
ground truth set for audio chord recognition and mu-
sic analysis. In Proceedings of the 12th International
Society for Music Information Retrieval Conference,
pages 633–638.
Forsyth, J. P. and Bello, J. (2013). Generating musical ac-
companiment using finite state transducers. In Con-
ference on Digital Audio Effects, National University
of Ireland.
Gagniuc, P. A. (2017). Markov Chains: From Theory to
Implementation and Experimentation. Wiley.
Geman, S. and Geman, D. (1984). Stochastic relaxation,
gibbs distributions, and the bayesian restoration of im-
ages. IEEE Transactions on Pattern Analysis and Ma-
chine Intelligence, PAMI-6(6):721–741.
Heinz, J. and Rogers, J. (2010). Estimating strictly piece-
wise distributions. In Proceedings of the 48th Annual
Meeting of the Association for Computational Lin-
guistics, ACL ’10, pages 886–896, Stroudsburg, PA,
USA. Association for Computational Linguistics.
Jarosz, G. (2013). Learning with hidden structure in opti-
mality theory and harmonic grammar: beyond robust
interpretive parsing. Phonology, 30(1):27–71.
Jarosz, G. (2015a). Expectation driven learning of phonol-
ogy*.
Jarosz, G. (2015b). Learning opaque and transparent inter-
actions in harmonic serialism.
J
¨
ager, G. and Rogers, J. (2012). Formal language theory:
Refining the chomsky hierarchy. Philosophical trans-
actions of the Royal Society of London. Series B, Bio-
logical sciences, 367:1956–70.
Johnson, M., Griffiths, T., and Goldwater, S. (2007).
Bayesian inference for pcfgs via markov chain monte
carlo. In Human Language Technologies 2007: The
Conference of the North American Chapter of the As-
sociation for Computational Linguistics; Proceedings
of the Main Conference, pages 139–146, Rochester,
New York. Association for Computational Linguis-
tics.
Kitani, K. and Koike, H. (2010). Improvgenerator: On-
line grammatical induction for on-the-fly improvisa-
tion accompaniment. In Proceedings of the 2010 Con-
ference on New Interfaces for Musical Expression.
Mao, H., Chen, Y., Jaeger, M., Nielsen, T., Larsen, K., and
Nielsen, B. (2012). Learning markov decision pro-
cesses for model checking. Electronic Proceedings in
Theoretical Computer Science, 103.
Nakamura, E., Nishikimi, R., Dixon, S., and Yoshii, K.
(2018). Probabilistic sequential patterns for singing
transcription. In 2018 Asia-Pacific Signal and Infor-
mation Processing Association Annual Summit and
Conference (APSIPA ASC), pages 1905–1912.
Nakano, M., Ohishi, Y., Kameoka, H., Mukai, R., and
Kashino, K. (2014). Mondrian hidden markov model
for music signal processing. In 2014 IEEE Interna-
tional Conference on Acoustics, Speech and Signal
Processing (ICASSP), pages 2405–2409.
Nouri, A., Raman, B., Bozga, M., Legay, A., and Bensalem,
S. (2014). Faster statistical model checking by means
of abstraction and learning. In Bonakdarpour, B. and
Smolka, S. A., editors, Runtime Verification, pages
340–355, Cham. Springer International Publishing.
Shibata, C. (2014). Inferring (k,l)-context-sensitive prob-
abilistic context-free grammars using hierarchical
pitman-yor processes. In Clark, A., Kanazawa, M.,
and Yoshinaka, R., editors, The 12th International
Conference on Grammatical Inference, volume 34 of
ICAART 2021 - 13th International Conference on Agents and Artificial Intelligence
578