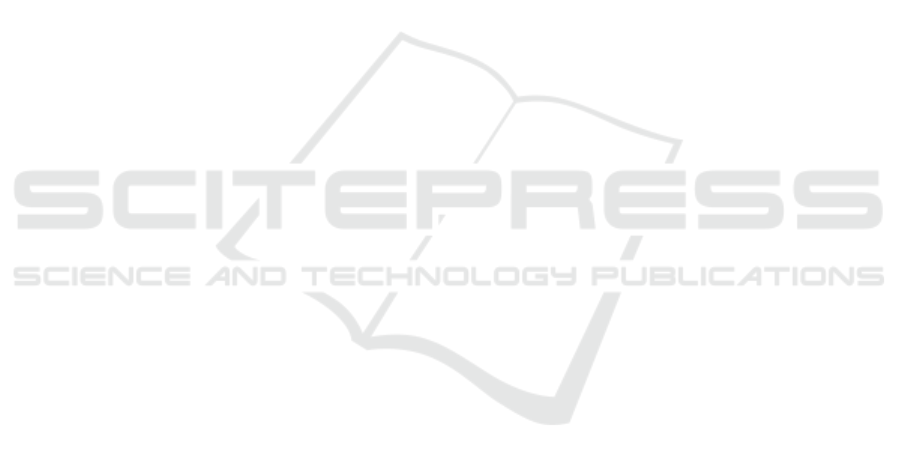
and object size. We directly prove this by visualizing
the offset field in the feature map viewpoint and the
kernel viewpoint separately. The position of the ob-
ject is global information which is in the feature map
viewpoint while the size of the object is local infor-
mation which is in the kernel viewpoint. The effect of
the offset field in the two viewpoints is investigated
separately and the results show the components in the
kernel viewpoint improves the deformable convolu-
tion more.
REFERENCES
Chen, Y., Zhang, Z., Cao, Y., Wang, L., Lin, S., and
Hu, H. (2020). Reppoints v2: Verification meets
regression for object detection. arXiv preprint
arXiv:2007.08508.
Dai, J., Qi, H., Xiong, Y., Li, Y., Zhang, G., Hu, H., and
Wei, Y. (2017). Deformable convolutional networks.
In Proceedings of the IEEE international conference
on computer vision, pages 764–773.
Gao, H., Zhu, X., Lin, S., and Dai, J. (2019). Deformable
kernels: Adapting effective receptive fields for object
deformation. arXiv preprint arXiv:1910.02940.
Kong, T., Sun, F., Liu, H., Jiang, Y., Li, L., and Shi,
J. (2020). Foveabox: Beyound anchor-based object
detection. IEEE Transactions on Image Processing,
29:7389–7398.
Lin, T.-Y., Maire, M., Belongie, S., Hays, J., Perona, P.,
Ramanan, D., Doll
´
ar, P., and Zitnick, C. L. (2014).
Microsoft coco: Common objects in context. In Euro-
pean conference on computer vision, pages 740–755.
Springer.
Ribeiro, M. T., Singh, S., and Guestrin, C. (2016). ” why
should i trust you?” explaining the predictions of any
classifier. In Proceedings of the 22nd ACM SIGKDD
international conference on knowledge discovery and
data mining, pages 1135–1144.
Simonyan, K. and Zisserman, A. (2014). Very deep con-
volutional networks for large-scale image recognition.
arXiv preprint arXiv:1409.1556.
Thomas, H., Qi, C. R., Deschaud, J.-E., Marcotegui, B.,
Goulette, F., and Guibas, L. J. (2019). Kpconv: Flex-
ible and deformable convolution for point clouds. In
Proceedings of the IEEE International Conference on
Computer Vision, pages 6411–6420.
Vu, T., Jang, H., Pham, T. X., and Yoo, C. (2019). Cas-
cade rpn: Delving into high-quality region proposal
network with adaptive convolution. In Advances in
Neural Information Processing Systems, pages 1432–
1442.
Wang, H., Wang, Z., Du, M., Yang, F., Zhang, Z., Ding, S.,
Mardziel, P., and Hu, X. (2020). Score-cam: Score-
weighted visual explanations for convolutional neu-
ral networks. In Proceedings of the IEEE/CVF Con-
ference on Computer Vision and Pattern Recognition
Workshops, pages 24–25.
Wang, J., Chen, K., Yang, S., Loy, C. C., and Lin, D. (2019).
Region proposal by guided anchoring. In Proceedings
of the IEEE Conference on Computer Vision and Pat-
tern Recognition, pages 2965–2974.
Yang, Z., Liu, S., Hu, H., Wang, L., and Lin, S. (2019a).
Reppoints: Point set representation for object detec-
tion. In Proceedings of the IEEE International Con-
ference on Computer Vision, pages 9657–9666.
Yang, Z., Xu, Y., Xue, H., Zhang, Z., Urtasun, R., Wang, L.,
Lin, S., and Hu, H. (2019b). Dense reppoints: Rep-
resenting visual objects with dense point sets. arXiv
preprint arXiv:1912.11473.
Zeiler, M. D. and Fergus, R. (2014). Visualizing and under-
standing convolutional networks. In European confer-
ence on computer vision, pages 818–833. Springer.
Zhou, B., Khosla, A., Lapedriza, A., Oliva, A., and Tor-
ralba, A. (2016). Learning deep features for discrim-
inative localization. In Proceedings of the IEEE con-
ference on computer vision and pattern recognition,
pages 2921–2929.
Zhu, X., Hu, H., Lin, S., and Dai, J. (2019). Deformable
convnets v2: More deformable, better results. In Pro-
ceedings of the IEEE Conference on Computer Vision
and Pattern Recognition, pages 9308–9316.
Revisiting the Deformable Convolution by Visualization
195