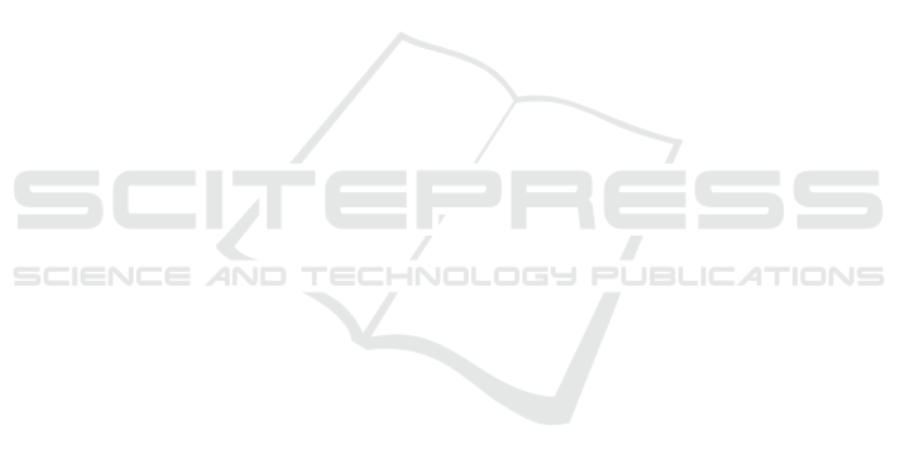
can be understood as a snapshot of the practice that
might differ in different industry sectors.
There are additional points that should be ad-
dressed in future work. Especially the definition of
AI and the measurement of its’ benefit has to be done
by a broader community in a joint effort. But not only
the definition is important; everyone can start right
now to use the term AI more carefully and question it
as needed. To ease the usage of AI, it can be supported
through projects such as ManuBrain or KOARCH.
Even if these projects follow a general idea, they fo-
cus on specific aspects of the (semi-)automatic appli-
cation of AI.
Properly used, AI is beneficial to companies and
can actively support problem solving in different ar-
eas of manufacturing.
ACKNOWLEDGEMENT
The work was supported by the German Federal Min-
istry of Education and Research (BMBF) under the
projects ”KOARCH” (funding code: 13FH007IA6).
REFERENCES
Abadi, M., Barham, P., and Chen et al., J. (2016). Tensor-
flow: A system for large-scale machine learning. In
12th USENIX Symposium on Operating Systems De-
sign and Implementation (OSDI 16), pages 265–283,
Savannah, GA. USENIX Association.
Ahmed, Z., Amizadeh, S., and Bilenko et al., M. (2019).
Machine learning at microsoft with ML .net. CoRR,
abs/1905.05715.
Bauernhansl, T., Kr
¨
uger, J., Reinhart, G., and Schuh, G.
(2016). WGP-Standpunkt Industrie 4.0. Technical re-
port, Wissenschaftliche Gesellschaft f
¨
ur Produktion-
stechnik WGP e. V., Darmstadt.
Bunte, A. (2020). Koarch website.
Burggr
¨
af, P. (2020). Manubrain website.
Feurer, M., Klein, A., Eggensperger, K., Springenberg, J.,
Blum, M., and Hutter, F. (2015). Efficient and Robust
Automated Machine Learning. In Advances in Neu-
ral Information Processing Systems 28, pages 2962–
2970. Curran Associates, Inc.
Fischbach, A., Strohschein, J., Bunte, A., Stork, J.,
Faeskorn-Woyke, H., Moriz, N., and Bartz-Beielstein,
T. (2020). CAAI – A Cognitive Architecture to Intro-
duce Artificial Intelligence in Cyber-Physical Produc-
tion Systems. The International Journal of Advanced
Manufacturing Technology.
Ghatak, A. (2017). Introduction to Machine Learning,
pages 57–78. Springer Singapore, Singapore.
Gottfredson, L. S. (1994). Mainstream science on intelli-
gence: An editorial with 52 signatories, history, and
bibliography. Wall Street Journal.
Hofst
¨
atter, P. (1966). Einf
¨
uhrung in die Sozialpsychologie,
volume 4. Kr
¨
oner Stuttgart.
Kagermann, H., Wahlster, W., and Helbig, J. (2013). Rec-
ommendations for Implementing the Strategic Ini-
tiative Industrie 4.0 – Securing the Future of Ger-
man Manufacturing Industry. Technical report, acat-
ech – National Academy of Science and Engineering,
M
¨
unchen.
Niggemann, O., Stein, B., Voden
ˇ
carevi
´
c, A., Maier, A., and
Kleine Buning, H. (2012). Learning Behavior Models
for Hybrid Timed Systems. In Twenty-Sixth Confer-
ence on Artificial Intelligence (AAAI-12).
Olson, R. S., Bartley, N., Urbanowicz, R. J., and Moore,
J. H. (2016). Evaluation of a tree-based pipeline op-
timization tool for automating data science. In Pro-
ceedings of the Genetic and Evolutionary Computa-
tion Conference 2016, GECCO ’16, New York, NY,
USA. ACM.
R
¨
ußmann, M., Lorenz, M., and et al. (2015). Industry 4.0:
The Future of Productivity and Growth in Manufac-
turing Industries. Technical report, The Boston Con-
sulting Group (BCG), M
¨
unchen.
Shrestha, G. M. and Niggemann, O. (2014). A bayesian
predictive assistance system for resource optimization
— a case study in industrial cleaning process. In Pro-
ceedings of the 2014 IEEE Emerging Technology and
Factory Automation (ETFA), pages 1–6.
Shrestha, G. M. and Niggemann, O. (2015). Hybrid ap-
proach combining bayesian network and rule-based
systems for resource optimization in industrial clean-
ing processes. In 2015 IEEE 20th Conference on
Emerging Technologies Factory Automation (ETFA),
pages 1–4.
Stern, W. (1912). Die psychologischen Methoden der Intel-
ligenzpr
¨
ufung und deren Anwendung an Schulkindern.
Kongress f
¨
ur experimentelle Psychologie. Bericht.
J.A. Barth.
von Birgelen, A., Buratti, D., Mager, J., and Niggemann,
O. (2018). Self-organizing maps for anomaly local-
ization and predictive maintenance in cyber-physical
production systems. Procedia CIRP, 72:480 – 485.
51st CIRP Conference on Manufacturing Systems.
Wahlster, W. (2017). Artificial Intelligence for Industrie 4.0.
In Proceedings of the KI 2017: Advances in Artificial
Intelligence, 40th Annual German Conference on AI,
Heidelberg, Germany. Springer.
ICAART 2021 - 13th International Conference on Agents and Artificial Intelligence
620