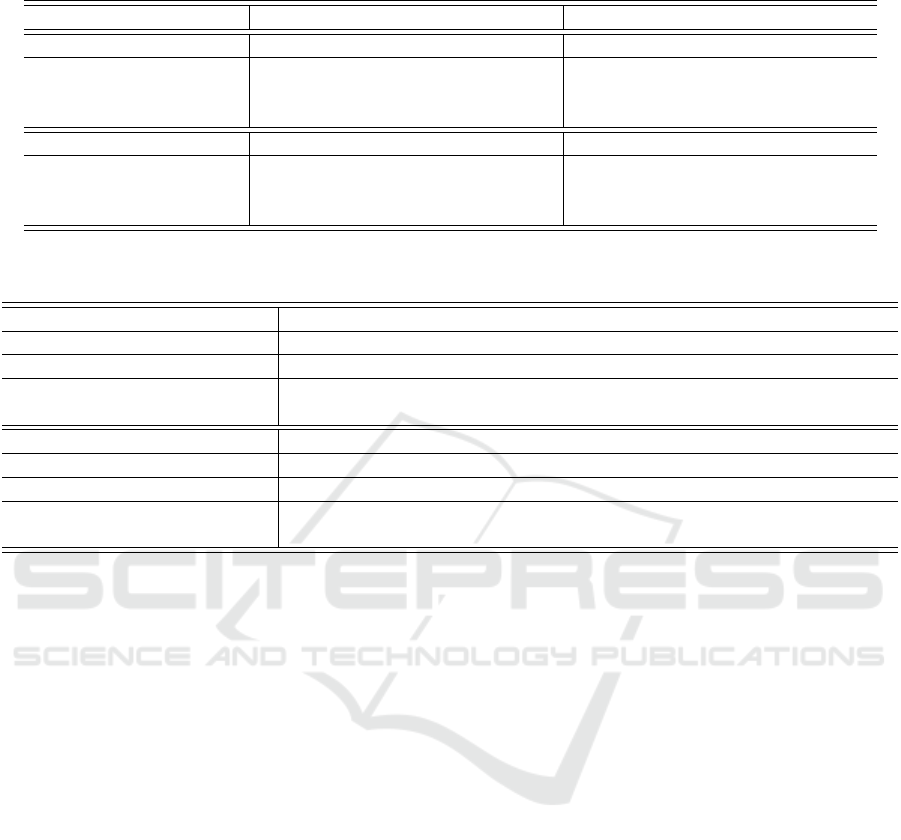
Table 6: Weight learning of formulas with unique occurrence of Hearst pairs vs multiple occurrence of Hearst pairs.
Unique occurrence Multiple occurrence
Initial weights w
1
= 1, w
2
= 1 w
1
= 10, w
2
= 1
Prob. using initial weights m(“ f ruit, f uel”) = 0.6600 m(“ f ruit, f uel”) = 0.9990
m(“orange, f uel”) = 0.7600 m(“orange, f uel”) = 0.7460
m(“company, f uel”) = 0.7600 m(“company, f uel”) = 0.8970
Learned weights w
1
= 4.3, w
2
= 13.1 w
1
= 2.2, w
2
= 8.1
Prob. using actual weights m(“ f ruit, f uel”) = 0.9400 m(“ f ruit, f uel”) = 0.7900
m(“orange, f uel”) = 0.9400 m(“orange, f uel”) = 0.8300
m(“company, f uel”) = 0.9900 m(“company, f uel”) = 0.8100
Table 7: Inference results using the data driven approach(Li et al., 2013) vs the proposed approach for a MLN method using
small example dataset.
Single occurrence Existing Method (Li et al., 2013) Proposed Method
Modern decision parameters δ = 0.5714 w
1
= 10, w
2
= 1
Marginal prob. of (x, y) m(“Orange, f uel”) = 0.1428 m(“Orange,fuel”) = 0.5800
Prob. of derived metaphor (h
x
, y) m(“ f ruit, f uel”) = 0.2857 m(“fruit,fuel”)= 0.6600
m(“company, f uel”) = 0.1428 m(“company,fuel”)= 0.7600
Multiple occurrence
Modern decision parameters δ = 0.5849 w
1
= 10, w
2
= 1
Marginal prob. of (x, y) m(“Orange, f uel”) = 0.2830 m(“Orange,fuel”) = 0.7460
Prob. of derived metaphor (h
x
, y) m(“ f ruit, f uel”) = 0.5660 m(“fruit,fuel”) = 0.9990
m(“company, f uel”) = 0.2325 m(“company,fuel”) = 0.8970
REFERENCES
Cheng, J., Wang, Z., Wen, J.-R., Yan, J., and Chen, Z.
(2015). Contextual text understanding in distribu-
tional semantic space. In ACM International Con-
ference on Information and Knowledge Management
(CIKM). ACM - Association for Computing Machin-
ery.
Doan, A., Niu, F., R
´
e, C., Shavlik, J., and Zhang, C. (2011).
User manual of tuffy 0.3. Technical report.
Domingos, P., Kok, S., Lowd, D., Poon, H., Richardson, M.,
and Singla, P. (2008). Markov Logic, pages 92–117.
Springer Berlin Heidelberg, Berlin, Heidelberg.
Fass, D. (1991). met*: A method for discriminating
metonymy and metaphor by computer. Computational
Linguistics, 17(1):49–90.
Lakoff, G. and Johnson, M. (1980). Metaphors we Live by.
University of Chicago Press, Chicago.
Li, H., Zhu, K. Q., and Wang, H. (2013). Data-driven
metaphor recognition and explanation. Transac-
tions of the Association for Computational Linguis-
tics, 1:379–390.
Niu, F., R
´
e, C., Doan, A., and Shavlik, J. W. (2011). Tuffy:
Scaling up statistical inference in markov logic net-
works using an RDBMS. CoRR, abs/1104.3216.
Richardson, M. and Domingos, P. (2006). Markov logic
networks. Machine learning, 62(1-2):107–136.
Schulder, M. and Hovy, E. (2014). Metaphor detection
through term relevance. In Proceedings of the Second
Workshop on Metaphor in NLP, pages 18–26, Balti-
more, MD. Association for Computational Linguis-
tics.
Shutova, E. (2010). Models of metaphor in NLP. In Pro-
ceedings of the 48th Annual Meeting of the Associ-
ation for Computational Linguistics, pages 688–697,
Uppsala, Sweden. Association for Computational Lin-
guistics.
Tsvetkov, Y., Boytsov, L., Gershman, A., Nyberg, E., and
Dyer, C. (2014). Metaphor detection with cross-
lingual model transfer. In Proceedings of the 52nd
Annual Meeting of the Association for Computational
Linguistics (Volume 1: Long Papers), pages 248–258,
Baltimore, Maryland. Association for Computational
Linguistics.
Wu, W., Li, H., Wang, H., and Zhu, K. Q. (2012). Probase:
A probabilistic taxonomy for text understanding. In
ACM International Conference on Management of
Data (SIGMOD).
ICAART 2021 - 13th International Conference on Agents and Artificial Intelligence
628