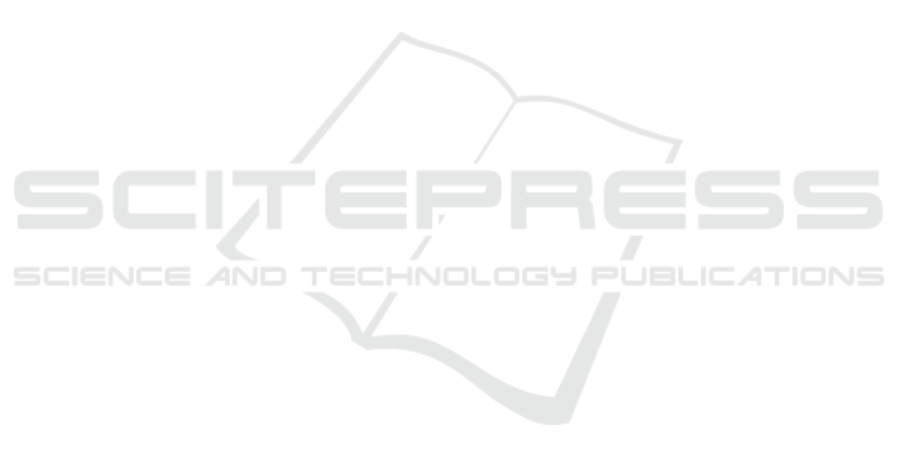
ment in performance was very evident but still the
concrete reason being unclear.
ACKNOWLEDGEMENTS
This work was partially supported by JSPS KAK-
ENHI Grant Number 18H03301.
REFERENCES
Abbeel, P. and Ng, A. Y. (2004). Apprenticeship learning
via inverse reinforcement learning. In Proceedings of
the twenty-first international conference on Machine
learning, page 1. ACM.
Alvarez, N. and Noda, I. (2018). Inverse reinforcement
learning for agents behavior in a crowd simulator. In
International Workshop on Massively Multiagent Sys-
tems, pages 81–95. Springer.
Choi, J. and Kim, K.-E. (2012). Nonparametric bayesian in-
verse reinforcement learning for multiple reward func-
tions. In Advances in Neural Information Processing
Systems, pages 305–313.
Crociani, L., Vizzari, G., Yanagisawa, D., Nishinari, K., and
Bandini, S. (2016). Route choice in pedestrian sim-
ulation: Design and evaluation of a model based on
empirical observations. Intelligenza Artificiale, 10(2).
Dvijotham, K. and Todorov, E. (2010). Inverse optimal con-
trol with linearly-solvable mdps. In Proceedings of the
27th International Conference on Machine Learning.
Faccin, J., Nunes, I., and Bazzan, A. (2017). Understand-
ing the Behaviour of Learning-Based BDI Agents in
the Braess’ Paradox, pages 187–204. Springer Inter-
national Publishing.
Herman, M., Gindele, T., Wagner, J., Schmitt, F., Quignon,
C., and Burgard, W. (2016). Learning high-level nav-
igation strategies via inverse reinforcement learning:
A comparative analysis. In Australasian Joint Confer-
ence on Artificial Intelligence. Springer.
Krishnan, S., Garg, A., Liaw, R., Miller, L., Pokorny, F. T.,
and Goldberg, K. (2016). Hirl: Hierarchical inverse
reinforcement learning for long-horizon tasks with de-
layed rewards. arXiv preprint arXiv:1604.06508.
L
¨
ammel, G., Grether, D., and Nagel, K. (2010). The rep-
resentation and implementation of time-dependent in-
undation in large-scale microscopic evacuation simu-
lations. Transportation Research Part C: Emerging
Technologies, 18(1):84–98.
Le Guillarme, N., Mouaddib, A.-i., Gatepaille, S., and Bel-
lenger, A. (2016). Adversarial intention recognition as
inverse game-theoretic planning for threat assessment.
Lelerre, M., Mouaddib, A.-i., and Jeanpierre, L. (2017). Ro-
bust inverse planning approaches for policy estimation
of semi-autonomous agents. pages 951–958.
Levine, S., Popovic, Z., and Koltun, V. (2011). Nonlin-
ear inverse reinforcement learning with gaussian pro-
cesses. In Advances in Neural Information Processing
Systems, pages 19–27.
Luo, L., Zhou, S., Cai, W., Low, M. Y. H., Tian, F., Wang,
Y., Xiao, X., and Chen, D. (2008). Agent-based hu-
man behavior modeling for crowd simulation. Com-
puter Animation and Virtual Worlds, 19(3-4).
Martinez-Gil, F., Lozano, M., and Fern
´
andez, F. (2017).
Emergent behaviors and scalability for multi-agent re-
inforcement learning-based pedestrian models. Simu-
lation Modelling Practice and Theory, 74:117–133.
Michini, B. and How, J. P. (2012). Bayesian nonparamet-
ric inverse reinforcement learning. In Joint European
Conference on Machine Learning and Knowledge
Discovery in Databases, pages 148–163. Springer.
Natarajan, S., Kunapuli, G., Judah, K., Tadepalli, P., Ker-
sting, K., and Shavlik, J. (2010). Multi-agent inverse
reinforcement learning. In Ninth International Con-
ference on Machine Learning and Applications. IEEE.
Neal, R. M. (2000). Markov chain sampling methods for
dirichlet process mixture models. Journal of compu-
tational and graphical statistics, 9(2):249–265.
Ng, A. Y., Russell, S. J., et al. (2000). Algorithms for in-
verse reinforcement learning. In Icml, pages 663–670.
Ram
´
ırez, M. and Geffner, H. (2009). Plan recognition as
planning. In Proceedings of the 21st International
Joint Conference on Artifical Intelligence, San Fran-
cisco, CA, USA. Morgan Kaufmann Publishers Inc.
Ram
´
ırez, M. and Geffner, H. (2011). Goal recognition over
pomdps: Inferring the intention of a pomdp agent. In
Proceedings of the 22nd International Joint Confer-
ence on Artificial Intelligence, IJCAI’11. AAAI Press.
Surana, A. and Srivastava, K. (2014). Bayesian nonpara-
metric inverse reinforcement learning for switched
markov decision processes. In Machine Learning and
Applications (ICMLA), 2014 13th International Con-
ference on, pages 47–54. IEEE.
Svetlik, M., Leonetti, M., Sinapov, J., Shah, R., Walker,
N., and Stone, P. (2016). Automatic curriculum graph
generation for reinforcement learning agents.
Torrens, P. M., Nara, A., Li, X., Zhu, H., Griffin, W. A., and
Brown, S. B. (2012). An extensible simulation en-
vironment and movement metrics for testing walking
behavior in agent-based models. Computers, Environ-
ment and Urban Systems, 36(1):1–17.
Yamashita, T., Soeda, S., and Noda, I. (2009). Evacua-
tion planning assist system with network model-based
pedestrian simulator. In PRIMA. Springer.
Zhong, J., Cai, W., Luo, L., and Zhao, M. (2016). Learning
behavior patterns from video for agent-based crowd
modeling and simulation. Autonomous Agents and
Multi-Agent Systems, 30(5):990–1019.
Ziebart, B. D., Maas, A. L., Bagnell, J. A., and Dey,
A. K. (2008). Maximum entropy inverse reinforce-
ment learning. In AAAI, volume 8, pages 1433–1438.
Chicago, IL, USA.
CAMP-IRL Agents: Extracting Multiple Behavior Profiles for Pedestrian Simulation
223