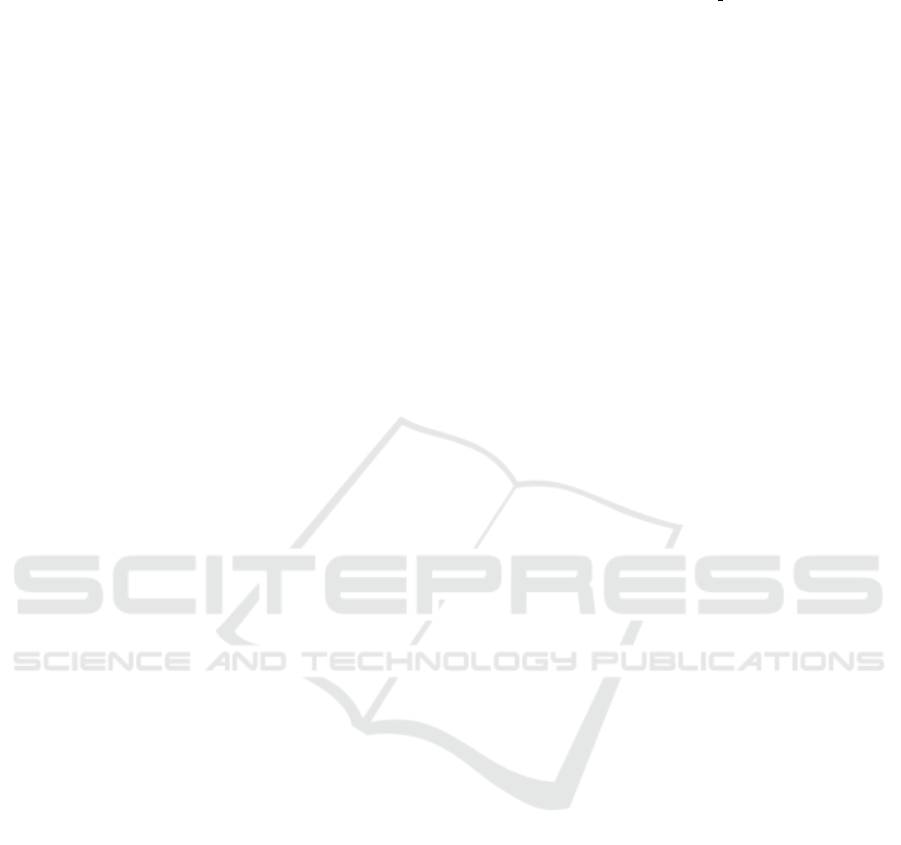
the computation time: this requires on average 50ms
to compute a solution, while the SAX method requires
15 seconds.
4 CONCLUSIONS
The contribution of this article is twofold: firstly, we
present a novel and open dataset of environmental
information acquired from indoor and outdoor sen-
sors deployed at SMART Infrastructure Facility at the
University of Wollongong, Australia; secondly, we
present a novel evolutionary approach to determine
in real-time the correlation between pairs of different
sensors.
This correlation is computed by using the infor-
mation coming from the sensors and two criteria: the
former based on the presence of both sensors inside
or outside a building, the latter on the spatial distance
among the sensors themselves. We experimentally
verify that this allows determining accurately corre-
lated pairs of sensors.
These techniques can be applied for different
smart building applications such as environmen-
tal monitoring to optimize energy consumption or
anomalies detection. In a real environment, sensors
can be subject to unpredictable anomalies that cause
missing information. A domain expert could be able
to understand if a given sensor is malfunctioning or
otherwise there is an emergency by using the pro-
posed system. For example, a high temperature could
indicate a malfunction, rather than the presence of
fire. In this case, the system should assist the domain
expert to determine whether a sensor is malfunction-
ing or there is an emergency.
We plan to extend our research by integrating
more information (e.g. luminosity or noise) and by
determining the state of devices (e.g. determining if a
door is open or closed).
REFERENCES
Ashby, W. R. (1991). Requisite variety and its implications
for the control of complex systems. In Facets of Sys-
tems Science, pages 405–417. Springer.
Barth
´
elemy, J., Arshad, B., Verstaevel, N., Guastella, D.,
and Perez, P. (2020a). Smart building additional data.
Barth
´
elemy, J., Arshad, B., Verstaevel, N., and Perez, P.
(2020b). Smart infrastructure facility building data.
Guastella, D. A., Camps, V., and Gleizes, M.-P. (2019). Es-
timating missing environmental information by con-
textual data cooperation. In Baldoni, M., Dastani,
M., Liao, B., Sakurai, Y., and Zalila Wenkstern, R.,
editors, PRIMA 2019: Principles and Practice of
Multi-Agent Systems, pages 523–531. Springer. doi:
10.1007/978-3-030-33792-6 37.
Guastella, D. A. and Valenti, C. (2018). Estimating miss-
ing information by cluster analysis and normalized
convolution. In 2018 IEEE 4th International Forum
on Research and Technology for Society and Industry
(RTSI), pages 1–6. doi: 10.1109/RTSI.2018.8548454.
Houssin, M., Combettes, S., Gleizes, M.-P., and Lartigue,
B. (2020). SANDMAN: a Self-Adapted System for
Anomaly Detection in Smart Buildings Data Streams.
In (to appear in) Proceedings of the 18th Adaptive
Computing (and Agents) for Enhanced Collaboration
(ACEC) at WETICE 2020.
Kenji Iwana, B. and Uchida, S. (2020). Time series
classification using local distance-based features in
multi-modal fusion networks. Pattern Recognition,
97:107024.
Nigon, J., Verstaevel, N., Boes, J., Migeon, F., and Gleizes,
M.-P. (2017). Smart is a matter of context. In Interna-
tional and Interdisciplinary Conference on Modeling
and Using Context, pages 189–202. Springer.
Otsu, N. (1979). A threshold selection method from gray-
level histograms. IEEE Transactions on Systems,
Man, and Cybernetics, 9(1):62–66.
Pociask, E., Malinowski, K. P.,
´
Sle¸zak, M., Jaworek-
Korjakowska, J., Wojakowski, W., and Roleder, T.
(2018). Fully automated lumen segmentation method
for intracoronary optical coherence tomography. Jour-
nal of Healthcare Engineering, 2018:1–13.
Sciortino, G., Tegolo, D., and Valenti, C. (2017). Auto-
matic detection and measurement of nuchal translu-
cency. Computers in Biology and Medicine, 82:12–
20.
Shyr-Shen, Y., Shao-Wei, C., Chuin-Mu, W., Yung-Kuan,
C., and Ting-Cheng, C. (2018). Two improved k-
means algorithms. Applied Soft Computing, 68:747
– 755.
Vonesch, C., Blu, T., and Unser, M. (2007). Generalized
Daubechies wavelet families. IEEE Transactions on
Signal Processing, 55(9):4415–4429.
Wang, L., Lu, F., Cui, M., and Bao, Y. (2019). Survey of
methods for time series symbolic aggregate approxi-
mation. In Cheng, X., Jing, W., Song, X., and Lu, Z.,
editors, Data Science, pages 645–657. Springer.
Zhou, H., Deng, Z., Xia, Y., and Fu, M. (2016). A new
sampling method in particle filter based on pearson
correlation coefficient. Neurocomputing, 216:208 –
215.
Evaluating Correlations in IoT Sensors for Smart Buildings
231