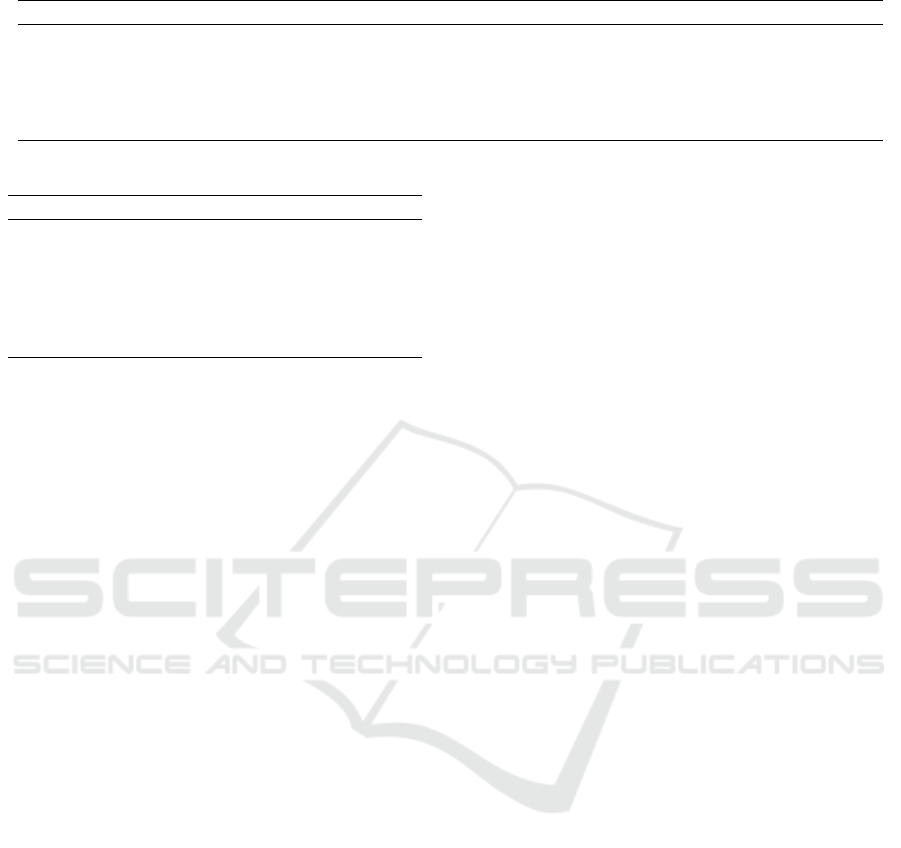
Table 2: Comparisons with other methods on HRSC2016.
Method Backbone mAP
R
2
CNN(Jiang et al., 2017) ResNet101 79.73
RRPN(Ma et al., 2018) ResNet101 85.64
RetinaNet-H(Yang et al., 2019a) ResNet101 89.27
DRN(Pan et al., 2020) Hourglass104 92.70
Ours ResNet50 93.16
Table 3: Ablation study on HRSC2016.
FPN PAM FSM L
min
AP
X 89.88
X X 90.92
X X 90.21
X X 90.87
X X X 91.43
X X X X 91.91
of region proposals. In addition, we adopt the eight-
parameter method to represent the oriented object and
propose a novel modulated loss function to address
the problem of the representation way when the tar-
get is nearly horizontal. We conduct extensive exper-
iments to illustrate the achievements of our method
across two datasets DOTA and HRSC2016 in compar-
ison with several qualitative approaches and the effect
of each module is shown by ablation study.
REFERENCES
Azimi, S. M., Vig, E., Bahmanyar, R., K
¨
orner, M., and
Reinartz, P. (2018). Towards multi-class object de-
tection in unconstrained remote sensing imagery. In
Asian Conference on Computer Vision, pages 150–
165. Springer.
Cai, Z. and Vasconcelos, N. (2018). Cascade r-cnn: Delving
into high quality object detection. In Proceedings of
the IEEE conference on computer vision and pattern
recognition, pages 6154–6162.
Dai, J., Li, Y., He, K., and Sun, J. (2016). R-fcn: Object de-
tection via region-based fully convolutional networks.
In Advances in neural information processing sys-
tems, pages 379–387.
Ding, J., Xue, N., Long, Y., Xia, G.-S., and Lu, Q. (2019).
Learning roi transformer for oriented object detection
in aerial images. In Proceedings of the IEEE Con-
ference on Computer Vision and Pattern Recognition,
pages 2849–2858.
Duan, K., Bai, S., Xie, L., Qi, H., Huang, Q., and Tian, Q.
(2019). Centernet: Keypoint triplets for object detec-
tion. In Proceedings of the IEEE International Con-
ference on Computer Vision, pages 6569–6578.
Ghiasi, G., Fowlkes, C. C., et al. (2016). Laplacian recon-
struction and refinement for semantic segmentation.
arXiv preprint arXiv:1605.02264, 4(4).
Ghiasi, G., Lin, T.-Y., and Le, Q. V. (2019). Nas-fpn: Learn-
ing scalable feature pyramid architecture for object
detection. In Proceedings of the IEEE conference on
computer vision and pattern recognition, pages 7036–
7045.
Girshick, R. (2015). Fast r-cnn. In Proceedings of the IEEE
international conference on computer vision, pages
1440–1448.
Girshick, R., Donahue, J., Darrell, T., and Malik, J. (2014).
Rich feature hierarchies for accurate object detec-
tion and semantic segmentation. In Proceedings of
the IEEE conference on computer vision and pattern
recognition, pages 580–587.
Jiang, Y., Zhu, X., Wang, X., Yang, S., Li, W., Wang, H.,
Fu, P., and Luo, Z. (2017). R2cnn: rotational region
cnn for orientation robust scene text detection. arXiv
preprint arXiv:1706.09579.
Li, Y., Huang, Q., Pei, X., Jiao, L., and Shang, R. (2020).
Radet: Refine feature pyramid network and multi-
layer attention network for arbitrary-oriented object
detection of remote sensing images. Remote Sensing,
12(3):389.
Liao, M., Shi, B., and Bai, X. (2018a). Textboxes++: A
single-shot oriented scene text detector. IEEE trans-
actions on image processing, 27(8):3676–3690.
Liao, M., Zhu, Z., Shi, B., Xia, G.-s., and Bai, X. (2018b).
Rotation-sensitive regression for oriented scene text
detection. In Proceedings of the IEEE conference on
computer vision and pattern recognition, pages 5909–
5918.
Lin, T.-Y., Doll
´
ar, P., Girshick, R., He, K., Hariharan, B.,
and Belongie, S. (2017a). Feature pyramid networks
for object detection. In Proceedings of the IEEE con-
ference on computer vision and pattern recognition,
pages 2117–2125.
Lin, T.-Y., Goyal, P., Girshick, R., He, K., and Doll
´
ar, P.
(2017b). Focal loss for dense object detection. In
Proceedings of the IEEE international conference on
computer vision, pages 2980–2988.
Liu, S., Huang, D., and Wang, Y. (2019). Learning spatial
fusion for single-shot object detection. arXiv preprint
arXiv:1911.09516.
Liu, S., Qi, L., Qin, H., Shi, J., and Jia, J. (2018). Path ag-
gregation network for instance segmentation. In Pro-
ceedings of the IEEE conference on computer vision
and pattern recognition, pages 8759–8768.
Liu, W., Anguelov, D., Erhan, D., Szegedy, C., Reed, S.,
Fu, C.-Y., and Berg, A. C. (2016). Ssd: Single shot
multibox detector. In European conference on com-
puter vision, pages 21–37. Springer.
ICPRAM 2021 - 10th International Conference on Pattern Recognition Applications and Methods
42