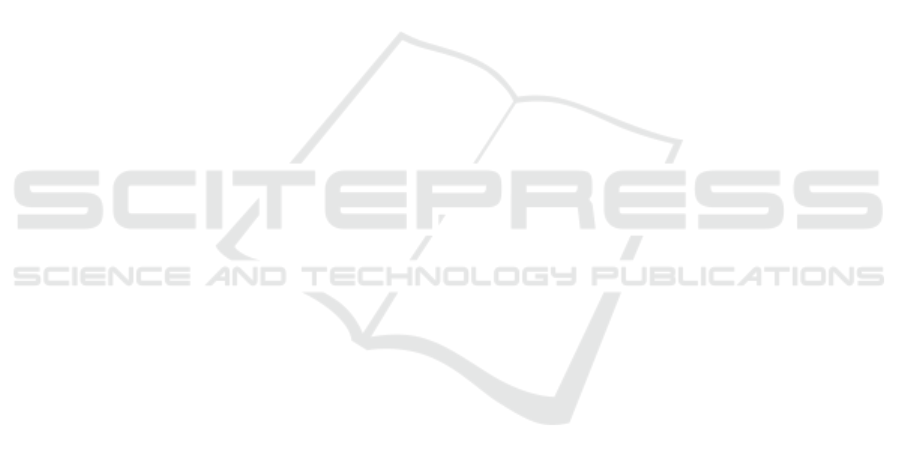
Liver segmentation: indications, techniques and fu-
ture directions. Insights into imaging, 8(4):377–392.
He, K., Zhang, X., Ren, S., and Sun, J. (2015). Delv-
ing deep into rectifiers: Surpassing human-level per-
formance on imagenet classification. In Proceedings
of the IEEE international conference on computer vi-
sion, pages 1026–1034.
Kavur, A. E., Gezer, N. S., Barıs¸, M., Conze, P.-H., Groza,
V., Pham, D. D., Chatterjee, S., Ernst, P.,
¨
Ozkan, S.,
Baydar, B., Lachinov, D., Han, S., Pauli, J., Isensee,
F., Perkonigg, M., Sathish, R., Rajan, R., Aslan, S.,
Sheet, D., Dovletov, G., Speck, O., N
¨
urnberger, A.,
Maier-Hein, K. H., Akar, G. B.,
¨
Unal, G., Dicle, O.,
and Selver, M. A. (2020). Chaos challenge – com-
bined (ct-mr) healthy abdominal organ segmentation.
Kavur, A. E., Selver, M. A., Dicle, O., Barıs¸, M., and Gezer,
N. S. (2019). CHAOS - Combined (CT-MR) Healthy
Abdominal Organ Segmentation Challenge Data.
Kr
¨
ahenb
¨
uhl, P. and Koltun, V. (2011). Efficient inference in
fully connected crfs with gaussian edge potentials. In
Advances in neural information processing systems,
pages 109–117.
Larkin, K. G. (2015). Structural similarity index ssimpli-
fied: Is there really a simpler concept at the heart
of image quality measurement? arXiv preprint
arXiv:1503.06680.
Lu, L., Shin, Y., Su, Y., and Karniadakis, G. E. (2019). Dy-
ing relu and initialization: Theory and numerical ex-
amples. arXiv preprint arXiv:1903.06733.
Nie, D., Wang, L., Gao, Y., and Shen, D. (2016). Fully con-
volutional networks for multi-modality isointense in-
fant brain image segmentation. In 2016 IEEE 13Th in-
ternational symposium on biomedical imaging (ISBI),
pages 1342–1345. IEEE.
Oktay, O., Schlemper, J., Folgoc, L. L., Lee, M., Heinrich,
M., Misawa, K., Mori, K., McDonagh, S., Hammerla,
N. Y., Kainz, B., et al. (2018). Attention u-net: Learn-
ing where to look for the pancreas. arXiv preprint
arXiv:1804.03999.
Paszke, A., Chaurasia, A., Kim, S., and Culurciello, E.
(2016). Enet: A deep neural network architecture
for real-time semantic segmentation. arXiv preprint
arXiv:1606.02147.
Shi, J. and Malik, J. (2000). Normalized cuts and image
segmentation. Departmental Papers (CIS), page 107.
Xia, X. and Kulis, B. (2017). W-net: A deep model for fully
unsupervised image segmentation. arXiv preprint
arXiv:1711.08506.
Zheng, S., Jayasumana, S., Romera-Paredes, B., Vineet, V.,
Su, Z., Du, D., Huang, C., and Torr, P. H. (2015). Con-
ditional random fields as recurrent neural networks. In
Proceedings of the IEEE international conference on
computer vision, pages 1529–1537.
ICPRAM 2021 - 10th International Conference on Pattern Recognition Applications and Methods
494