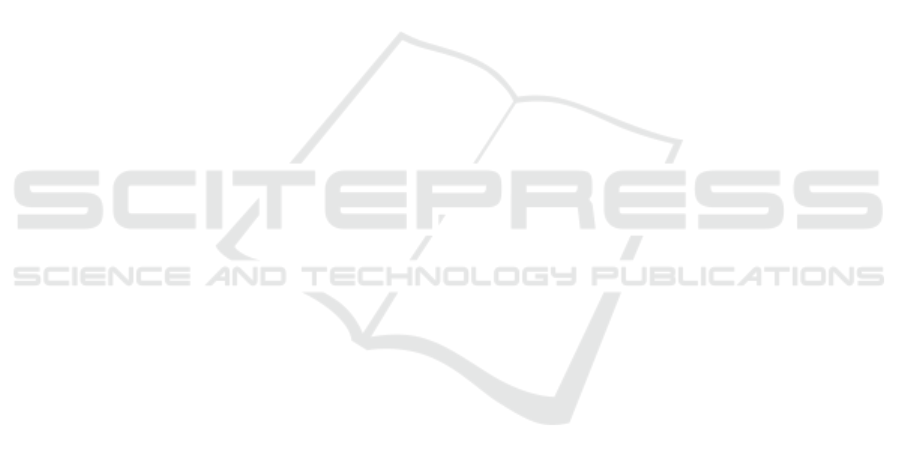
they fill out an account recovery form on Android
phones while sitting in a laboratory, we have per-
formed score-level fusion experiments, of two types,
namely, weighted score fusion and the likelihood ra-
tio based score fusion. In addition, we have also per-
formed both intra- and inter-session experiments.
By fusing both modalities, the likelihood ratio
based score fusion performs the best in both intra- and
inter-sessions, between the two score fusion strate-
gies, with EERs of 2.4% and 6.9%, respectively. An
average sliding window width of 80 for the best-
performing likelihood ratio approach is equivalent to
40 seconds of data per decision.
As shown in Table 1, in the score fusion of ac-
celeration experiment and score fusion of angular ve-
locity experiment, the average and the median EERs
are very close, which shows that the data is evenly
distributed. The standard deviation implies that the
EERs do not vary much among the users. Over-
all, the cross-modality fusion outperforms the within-
modality and the likelihood ratio based score fusion
performs the best in all experiments. Lastly, it is no-
ticed that in the score fusion experiments, the k and n
parameters of the sliding window are typically high.
Based on our sampling rate of 2 Hz, these would
amount to less than 2 minutes of data per authenti-
cation decision.
Our future work will include replicating this study
on other public data-sets to increase the reliability of
the reported performance. It will also be worthwhile
to investigate the fusion of motion events with other
modalities such as typing and swiping to identify the
optimal combination of multi-modalities while con-
sidering user experiences and usability.
ACKNOWLEDGEMENTS
This material is based upon work supported by
the Center for Identification Technology Research
(CITeR) and the National Science Foundation under
Grant No.1650503.
REFERENCES
Deb, D., Ross, A., Jain, A. K., Prakah-Asante, K., and
Prasad, K. V. (2019). Actions speak louder than (pass)
words: Passive authentication of smartphone* users
via deep temporal features. In 2019 International
Conference on Biometrics (ICB), pages 1–8. IEEE.
Derawi, M. O., Nickel, C., Bours, P., and Busch, C. (2010).
Unobtrusive user-authentication on mobile phones us-
ing biometric gait recognition. In 2010 Sixth Interna-
tional Conference on Intelligent Information Hiding
and Multimedia Signal Processing, pages 306–311.
IEEE.
Feng, T., Zhao, X., and Shi, W. (2013). Investigating mo-
bile device picking-up motion as a novel biometric
modality. In 2013 IEEE Sixth International Confer-
ence on Biometrics: Theory, Applications and Systems
(BTAS), pages 1–6. IEEE.
Giuffrida, C., Majdanik, K., Conti, M., and Bos, H. (2014).
I sensed it was you: authenticating mobile users with
sensor-enhanced keystroke dynamics. In Interna-
tional Conference on Detection of Intrusions and Mal-
ware, and Vulnerability Assessment, pages 92–111.
Springer.
Hong, F., Wei, M., You, S., Feng, Y., and Guo, Z. (2015).
Waving authentication: your smartphone authenticate
you on motion gesture. In Proceedings of the 33rd An-
nual ACM Conference Extended Abstracts on Human
Factors in Computing Systems, pages 263–266.
Jain, A. and Kanhangad, V. (2015). Exploring orientation
and accelerometer sensor data for personal authentica-
tion in smartphones using touchscreen gestures. Pat-
tern recognition letters, 68:351–360.
Kim, K.-E., Chang, W., Cho, S.-J., Shim, J., Lee, H., Park,
J., Lee, Y., Kim, S., et al. (2006). Hand grip pattern
recognition for mobile user interfaces. In Proceedings
of the National Conference on Artificial Intelligence,
volume 21, page 1789. Menlo Park, CA; Cambridge,
MA; London; AAAI Press; MIT Press; 1999.
Kumar, R., Phoha, V. V., and Serwadda, A. (2016). Con-
tinuous authentication of smartphone users by fusing
typing, swiping, and phone movement patterns. In
2016 IEEE 8th International Conference on Biomet-
rics Theory, Applications and Systems (BTAS), pages
1–8. IEEE.
Kwapisz, J. R., Weiss, G. M., and Moore, S. A. (2010).
Cell phone-based biometric identification. In 2010
Fourth IEEE International Conference on Biometrics:
Theory, Applications and Systems (BTAS), pages 1–7.
IEEE.
Lee, W.-H. and Lee, R. B. (2015). Multi-sensor authenti-
cation to improve smartphone security. In 2015 Inter-
national Conference on Information Systems Security
and Privacy (ICISSP), pages 1–11. IEEE.
Lin, C.-C., Chang, C.-C., Liang, D., and Yang, C.-H.
(2012). A new non-intrusive authentication method
based on the orientation sensor for smartphone users.
In 2012 IEEE Sixth International Conference on Soft-
ware Security and Reliability, pages 245–252. IEEE.
Nandakumar, K., Chen, Y., Dass, S. C., and Jain, A. (2007).
Likelihood ratio-based biometric score fusion. IEEE
transactions on pattern analysis and machine intelli-
gence, 30(2):342–347.
Ross, A., Nandakumar, K., and Jain, A. K. (2008). Intro-
duction to multibiometrics. In Handbook of biomet-
rics, pages 271–292. Springer.
Sitov
´
a, Z.,
ˇ
Sed
ˇ
enka, J., Yang, Q., Peng, G., Zhou, G., Gasti,
P., and Balagani, K. S. (2015). Hmog: New behav-
ioral biometric features for continuous authentication
of smartphone users. IEEE Transactions on Informa-
tion Forensics and Security, 11(5):877–892.
Continuous Authentication based on Hand Micro-movement during Smartphone Form Filling by Seated Human Subjects
431