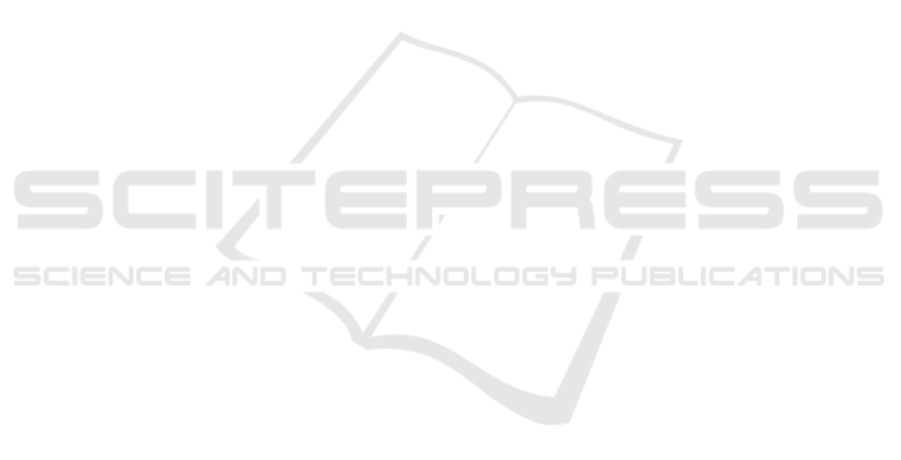
B. W. (2017). Snore sound classification using image-
based deep spectrum features. In INTERSPEECH,
volume 434, pages 3512–3516.
Bayram, B., Duman, T. B., and Ince, G. (2020). Real time
detection of acoustic anomalies in industrial processes
using sequential autoencoders. Expert Systems, page
e12564.
Beckmann, P., Kegler, M., Saltini, H., and Cernak, M.
(2019). Speech-vgg: A deep feature extractor for
speech processing. arXiv preprint arXiv:1910.09909.
Chi, P.-H., Chung, P.-H., Wu, T.-H., Hsieh, C.-C., Li, S.-
W., and Lee, H.-y. (2020). Audio albert: A lite bert
for self-supervised learning of audio representation.
arXiv preprint arXiv:2005.08575.
Cramer, J., Wu, H.-H., Salamon, J., and Bello, J. P. (2019).
Look, listen, and learn more: Design choices for
deep audio embeddings. In IEEE International Con-
ference on Acoustics, Speech and Signal Processing
(ICASSP), pages 3852–3856. IEEE.
Devlin, J., Chang, M.-W., Lee, K., and Toutanova, K.
(2019). Bert: Pre-training of deep bidirectional trans-
formers for language understanding. In Proceedings
of the 2019 Conference of the North American Chap-
ter of the Association for Computational Linguistics,
pages 4171–4186.
Donahue, J., Jia, Y., Vinyals, O., Hoffman, J., Zhang, N.,
Tzeng, E., and Darrell, T. (2014). Decaf: A deep con-
volutional activation feature for generic visual recog-
nition. In International conference on machine learn-
ing, pages 647–655.
Duman, T. B., Bayram, B., and
˙
Ince, G. (2019). Acoustic
anomaly detection using convolutional autoencoders
in industrial processes. In International Workshop on
Soft Computing Models in Industrial and Environmen-
tal Applications, pages 432–442. Springer.
Hasan, M. A., Abu-Bakar, M.-H., Razuwan, R., and Nazri,
Z. (2018). Deep neural network tool chatter model
for aluminum surface milling using acoustic emmi-
sion sensor. In MATEC Web of Conferences.
Hayashi, T., Komatsu, T., Kondo, R., Toda, T., and Takeda,
K. (2018). Anomalous sound event detection based on
wavenet. In 2018 26th European Signal Processing
Conference (EUSIPCO), pages 2494–2498. IEEE.
He, K., Zhang, X., Ren, S., and Sun, J. (2016). Deep resid-
ual learning for image recognition. In Proceedings of
the IEEE conference on computer vision and pattern
recognition, pages 770–778.
Hershey, S., Chaudhuri, S., Ellis, D. P., Gemmeke, J. F.,
Jansen, A., Moore, R. C., Plakal, M., Platt, D.,
Saurous, R. A., Seybold, B., et al. (2017). Cnn archi-
tectures for large-scale audio classification. In IEEE
International Conference on Acoustics, Speech and
Signal Processing (ICASSP), pages 131–135. IEEE.
Huang, G., Liu, Z., Van Der Maaten, L., and Weinberger,
K. Q. (2017). Densely connected convolutional net-
works. In Proceedings of the IEEE conference on
computer vision and pattern recognition, pages 4700–
4708.
Kawaguchi, Y., Tanabe, R., Endo, T., Ichige, K., and
Hamada, K. (2019). Anomaly detection based on an
ensemble of dereverberation and anomalous sound ex-
traction. In IEEE International Conference on Acous-
tics, Speech and Signal Processing (ICASSP), pages
865–869.
Koizumi, Y., Murata, S., Harada, N., Saito, S., and Ue-
matsu, H. (2019). Sniper: Few-shot learning for
anomaly detection to minimize false-negative rate
with ensured true-positive rate. In IEEE International
Conference on Acoustics, Speech and Signal Process-
ing (ICASSP), pages 915–919. IEEE.
Koizumi, Y., Saito, S., Uematsu, H., and Harada, N. (2017).
Optimizing acoustic feature extractor for anomalous
sound detection based on neyman-pearson lemma. In
2017 25th European Signal Processing Conference
(EUSIPCO), pages 698–702. IEEE.
Marchi, E., Vesperini, F., Eyben, F., Squartini, S., and
Schuller, B. (2015). A novel approach for automatic
acoustic novelty detection using a denoising autoen-
coder with bidirectional lstm neural networks. In
IEEE international conference on acoustics, speech
and signal processing (ICASSP), pages 1996–2000.
IEEE.
M
¨
uller, R., Illium, S., , Ritz, F., Schr
¨
oder, T., Platschek, C.,
Ochs, J., and Linnhoff-Popien, C. (2020a). Acoustic
leak detection in water networks. Technical report.
M
¨
uller, R., Ritz, F., Illium, S., and Linnhoff-Popien, C.
(2020b). Acoustic anomaly detection for machine
sounds based on image transfer learning. arXiv
preprint arXiv:2006.03429.
Oord, A. v. d., Dieleman, S., Zen, H., Simonyan, K.,
Vinyals, O., Graves, A., Kalchbrenner, N., Senior,
A., and Kavukcuoglu, K. (2016). Wavenet: A
generative model for raw audio. arXiv preprint
arXiv:1609.03499.
Peters, M. E., Neumann, M., Iyyer, M., Gardner, M., Clark,
C., Lee, K., and Zettlemoyer, L. (2018). Deep con-
textualized word representations. In Proceedings of
NAACL-HLT, pages 2227–2237.
Pons, J. and Serra, X. (2019). musicnn: Pre-trained con-
volutional neural networks for music audio tagging.
arXiv preprint arXiv:1909.06654.
Purohit, H., Tanabe, R., Ichige, K., Endo, T., Nikaido,
Y., Suefusa, K., and Kawaguchi, Y. (2019). Mimii
dataset: Sound dataset for malfunctioning industrial
machine investigation and inspection. arXiv preprint
arXiv:1909.09347.
Ruder, S. (2018). NLP’s ImageNet moment has arrived.
Rushe, E. and Namee, B. M. (2019). Anomaly detection
in raw audio using deep autoregressive networks. In
IEEE International Conference on Acoustics, Speech
and Signal Processing (ICASSP), pages 3597–3601.
Suefusa, K., Nishida, T., Purohit, H., Tanabe, R., Endo, T.,
and Kawaguchi, Y. (2020). Anomalous sound detec-
tion based on interpolation deep neural network. In
IEEE International Conference on Acoustics, Speech
and Signal Processing (ICASSP), pages 271–275.
ICAART 2021 - 13th International Conference on Agents and Artificial Intelligence
106