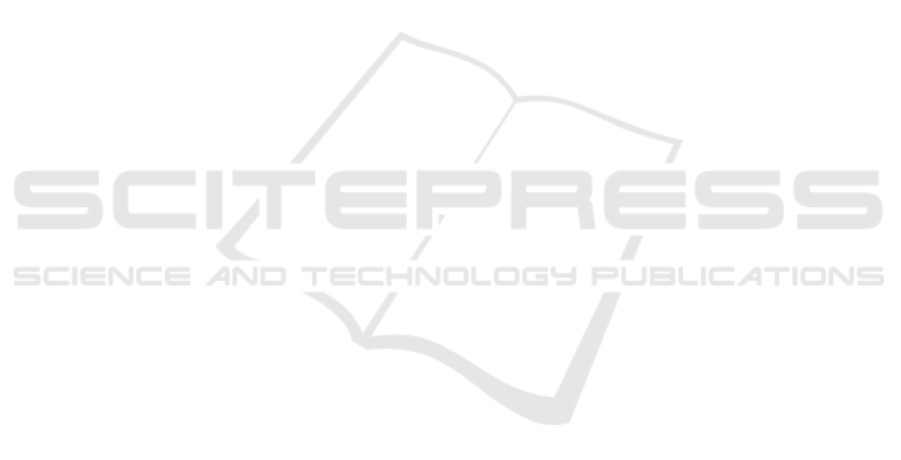
Jain, S. D. and Grauman, K. (2016). Active image segmen-
tation propagation. In 2016 IEEE Conference on Com-
puter Vision and Pattern Recognition (CVPR), pages
2864–2873.
Kasarla, T., Nagendar, G., Hegde, G. M., Balasubramanian,
V., and Jawahar, C. V. (2019). Region-based active
learning for efficient labeling in semantic segmenta-
tion. In 2019 IEEE Winter Conference on Applications
of Computer Vision (WACV), pages 1109–1117.
Konyushkova, K., Sznitman, R., and Fua, P. (2015). In-
troducing geometry in active learning for image seg-
mentation. In Proceedings of the IEEE International
Conference on Computer Vision, pages 2974–2982.
Long, J., Shelhamer, E., and Darrell, T. (2015). Fully con-
volutional networks for semantic segmentation. In
The IEEE Conference on Computer Vision and Pat-
tern Recognition (CVPR).
Maag, K., Rottmann, M., and Gottschalk, H. (2020). Time-
dynamic estimates of the reliability of deep semantic
segmentation networks. In 2020 IEEE International
Conference on Tools with Artificial Intelligence (IC-
TAI).
Mackowiak, R., Lenz, P., Ghori, O., Diego, F., Lange, O.,
and Rother, C. (2018). CEREALS - cost-effective
region-based active learning for semantic segmenta-
tion. CoRR, abs/1810.09726.
Mahapatra, D., Bozorgtabar, B., Thiran, J., and Reyes, M.
(2018). Efficient active learning for image classifica-
tion and segmentation using a sample selection and
conditional generative adversarial network. CoRR,
abs/1806.05473.
Mosinska, A., Tarnawski, J., and Fua, P. (2017). Active
learning and proofreading for delineation of curvilin-
ear structures. In International Conference on Med-
ical Image Computing and Computer-Assisted Inter-
vention, pages 165–173. Springer.
Neuhold, G., Ollmann, T., Rota Bulo, S., and Kontschieder,
P. (2017). The mapillary vistas dataset for semantic
understanding of street scenes. In Proceedings of the
IEEE International Conference on Computer Vision,
pages 4990–4999.
Özdemir, F., Peng, Z., Tanner, C., Fürnstahl, P., and Gok-
sel, O. (2018). Active learning for segmentation by
optimizing content information for maximal entropy.
CoRR, abs/1807.06962.
Ramer, U. (1972). An iterative procedure for the polygonal
approximation of plane curves. Computer graphics
and image processing, 1(3):244–256.
Ronneberger, O., Fischer, P., and Brox, T. (2015). U-net:
Convolutional networks for biomedical image seg-
mentation. In International Conference on Medical
image computing and computer-assisted intervention,
pages 234–241. Springer.
Rottmann, M., Colling, P., Hack, T. P., Chan, R., Hüger, F.,
Schlicht, P., and Gottschalk, H. (2020). Prediction er-
ror meta classification in semantic segmentation: De-
tection via aggregated dispersion measures of softmax
probabilities. In 2020 International Joint Conference
on Neural Networks (IJCNN), pages 1–9. IEEE.
Rottmann, M., Kahl, K., and Gottschalk, H. (2018).
Deep bayesian active semi-supervised learning. In
2018 17th IEEE International Conference on Machine
Learning and Applications (ICMLA), pages 158–164.
IEEE.
Rottmann, M. and Schubert, M. (2019). Uncertainty mea-
sures and prediction quality rating for the semantic
segmentation of nested multi resolution street scene
images. In Proceedings of the IEEE Conference on
Computer Vision and Pattern Recognition Workshops,
pages 0–0.
Sandler, M., Howard, A. G., Zhu, M., Zhmoginov, A., and
Chen, L.-C. (2018). Inverted residuals and linear bot-
tlenecks: Mobile networks for classification, detection
and segmentation. CoRR, abs/1801.04381.
Settles, B. (2009). Active learning literature survey. Com-
puter Sciences Technical Report 1648, University of
Wisconsin–Madison.
Shannon, C. E. (2001). A mathematical theory of communi-
cation, volume 5, pages 3–55. ACM New York, NY,
USA.
Siddiqui, Y., Valentin, J., and Nießner, M. (2019). Viewal:
Active learning with viewpoint entropy for semantic
segmentation. arXiv preprint arXiv:1911.11789.
Sun, C., Shrivastava, A., Singh, S., and Gupta, A. (2017).
Revisiting unreasonable effectiveness of data in deep
learning era. In Proceedings of the IEEE international
conference on computer vision, pages 843–852.
Vezhnevets, A., Buhmann, J. M., and Ferrari, V. (2012).
Active learning for semantic segmentation with ex-
pected change. In 2012 IEEE Conference on Com-
puter Vision and Pattern Recognition, pages 3162–
3169. IEEE.
Wang, J., Sun, K., Cheng, T., Jiang, B., Deng, C., Zhao,
Y., Liu, D., Mu, Y., Tan, M., Wang, X., Liu, W., and
Xiao, B. (2019). Deep high-resolution representation
learning for visual recognition. TPAMI.
Wang, K., Zhang, D., Li, Y., Zhang, R., and Lin, L. (2016).
Cost-effective active learning for deep image classi-
fication. IEEE Transactions on Circuits and Systems
for Video Technology, 27(12):2591–2600.
Yang, L., Zhang, Y., Chen, J., Zhang, S., and Chen, D. Z.
(2017). Suggestive annotation: A deep active learning
framework for biomedical image segmentation. pages
399–407.
Zhao, H., Shi, J., Qi, X., Wang, X., and Jia, J. (2017).
Pyramid scene parsing network. In Proceedings of
the IEEE conference on computer vision and pattern
recognition, pages 2881–2890.
ICPRAM 2021 - 10th International Conference on Pattern Recognition Applications and Methods
62