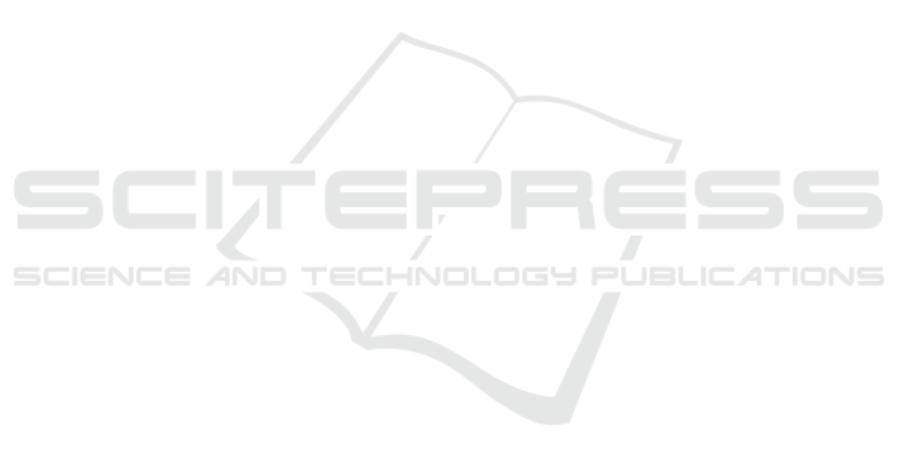
The efficiency of SCP does not reach the level of
ICP trained on pooled data, but efficiency is lower
(lower median prediction interval width) compared to
the predictions made on the best individual partition,
indicating attractive properties in distributed and fed-
erated settings as a valid confidence predictor. Future
directions when working on partitioned data include
(i) studying the effect of the number and size of data
partitions as well as overlapping partitions (ii) eval-
uating the effect of different nonconformity scores
and different underlying machine learning algorithms
with individual partitions.
REFERENCES
Beganovic, D. and Smirnov, E. (2018). Ensemble
cross-conformal prediction. In Proceedings of the
2018 IEEE International Conference on Data Min-
ing Workshops, ICDM Workshops, Singapore, Singa-
pore, November 17-20, 2018, pages 870–877, United
States. IEEE.
Carlsson, L., Eklund, M., and Norinder, U. (2014a). Ag-
gregated conformal prediction. In IFIP International
Conference on Artificial Intelligence Applications and
Innovations, pages 231–240. Springer.
Carlsson, L., Eklund, M., and Norinder, U. (2014b). Aggre-
gated conformal prediction. In Iliadis, L., Maglogian-
nis, I., Papadopoulos, H., Sioutas, S., and Makris, C.,
editors, Artificial Intelligence Applications and Inno-
vations, pages 231–240. Springer Berlin Heidelberg.
Elish, M. C. and danah boyd (2018). Situating methods in
the magic of big data and ai. Communication Mono-
graphs, 85(1):57–80.
Gandomi, A. and Haider, M. (2015). Beyond the hype: Big
data concepts, methods, and analytics. International
Journal of Information Management, 35(2):137–144.
Gauraha, N. and Spjuth, O. (2018). Synergy conformal pre-
diction. UU.
Hashem, I. A. T., Yaqoob, I., Anuar, N. B., Mokhtar, S.,
Gani, A., and Khan, S. U. (2015). The rise of “big
data” on cloud computing: Review and open research
issues. Information systems, 47:98–115.
Lichman, M. et al. (2013). Uci machine learning repository.
Linusson, H., Norinder, U., Bostr
¨
om, H., Johansson, U.,
and L
¨
ofstr
¨
om, T. (2017). On the calibration of ag-
gregated conformal predictors. In Gammerman, A.,
Vovk, V., Luo, Z., and Papadopoulos, H., editors, Pro-
ceedings of the Sixth Workshop on Conformal and
Probabilistic Prediction and Applications, volume 60
of Proceedings of Machine Learning Research, pages
154–173, Stockholm, Sweden. PMLR.
Meng, X., Bradley, J., Yavuz, B., Sparks, E., Venkataraman,
S., Liu, D., Freeman, J., Tsai, D., Amde, M., Owen,
S., et al. (2016). Mllib: Machine learning in apache
spark. The Journal of Machine Learning Research,
17(1):1235–1241.
Papadopoulos, H. (2008). Inductive conformal prediction:
Theory and application to neural networks. In Tools
in artificial intelligence, pages 315–329. InTech.
Papadopoulos, H. (2015). Cross-conformal prediction with
ridge regression. In International Symposium on Sta-
tistical Learning and Data Sciences, pages 260–270.
Springer.
Papadopoulos, H., Proedrou, K., Vovk, V., and Gammer-
man, A. (2002). Inductive confidence machines for re-
gression. In European Conference on Machine Learn-
ing, pages 345–356. Springer.
Park, S. and Budescu, D. V. (2015). Aggregating multiple
probability intervals to improve calibration. Judgment
and Decision Making, 10(2):130.
Peteiro-Barral, D. and Guijarro-Berdi
˜
nas, B. (2013). A
survey of methods for distributed machine learning.
Progress in Artificial Intelligence, 2(1):1–11.
Stephens, Z. D., Lee, S. Y., Faghri, F., Campbell, R. H.,
Zhai, C., Efron, M. J., Iyer, R., Schatz, M. C., Sinha,
S., and Robinson, G. E. (2015). Big data: astronomi-
cal or genomical? PLoS biology, 13(7):e1002195.
Svensson, F., Aniceto, N., Norinder, U., Cortes-Ciriano, I.,
Spjuth, O., Carlsson, L., and Bender, A. (2018). Con-
formal regression for quantitative structure–activity
relationship modeling—quantifying prediction uncer-
tainty. Journal of Chemical Information and Model-
ing, 58(5):1132–1140.
Vapnik, V. and Izmailov, R. (2016). Synergy of monotonic
rules. The Journal of Machine Learning Research,
17(1):4722–4754.
Vovk, V. (2013). Transductive conformal predictors. In
IFIP International Conference on Artificial Intelli-
gence Applications and Innovations, pages 348–360.
Springer.
Vovk, V. (2015). Cross-conformal predictors. Annals of
Mathematics and Artificial Intelligence, 74(1-2):9–28.
Vovk, V., Fedorova, V., Nouretdinov, I., and Gammerman,
A. (2016). Criteria of efficiency for conformal predic-
tion. In Symposium on Conformal and Probabilistic
Prediction with Applications, pages 23–39. Springer.
Vovk, V., Gammerman, A., and Shafer, G. (2005). Algo-
rithmic learning in a random world. Springer Science
& Business Media.
Zhou, L., Pan, S., Wang, J., and Vasilakos, A. V. (2017).
Machine learning on big data: Opportunities and chal-
lenges. Neurocomputing, 237:350 – 361.
Synergy Conformal Prediction for Regression
219