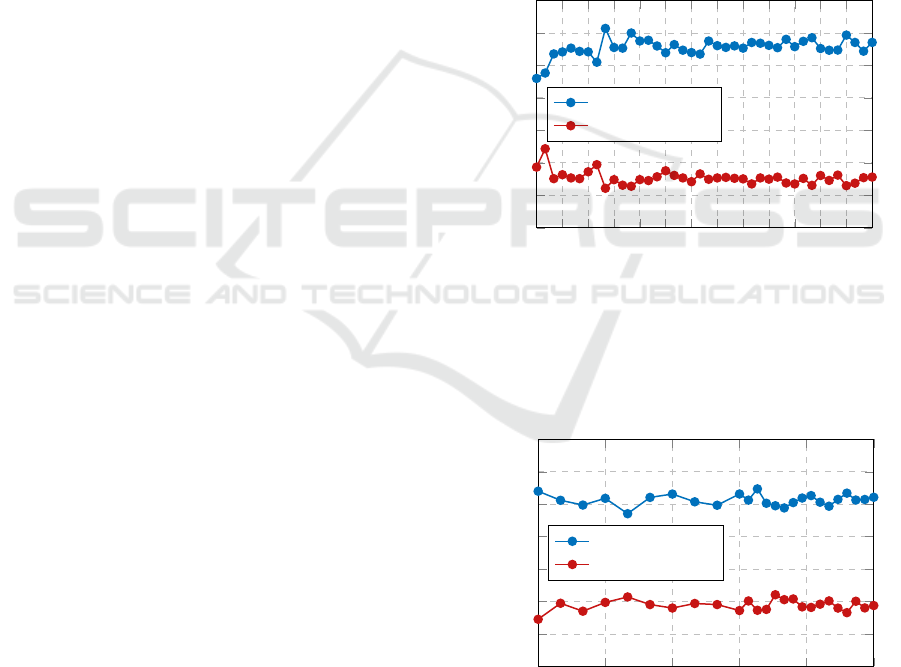
REFERENCES
Bidram, A., Lewis, F. L., and Davoudi, A. (2014). Dis-
tributed control systems for small-scale power net-
works: Using multiagent cooperative control theory.
IEEE Control Systems Magazine, 34(6):56–77.
de Roo, F. V., Tejada, A., van Waarde, H., and Trentelman,
H. L. (2015). Towards observer-based fault detection
and isolation for branched water distribution networks
without cycles. In 2015 European Control Conference
(ECC), pages 3280–3285. IEEE.
Gonc¸alves, J. and Warnick, S. (2008). Necessary and suf-
ficient conditions for dynamical structure reconstruc-
tion of lti networks. IEEE Transactions on Automatic
Control, 53(7):1670–1674.
Julius, A., Zavlanos, M., Boyd, S., and Pappas, G. J. (2009).
Genetic network identification using convex program-
ming. IET systems biology, 3(3):155–166.
Lewis, F. L., Zhang, H., Hengster-Movric, K., and Das, A.
(2014). Cooperative control of multi-agent systems:
Optimal and adaptive design approaches. Springer-
Verlag, London, England.
McKay, B. D. and Piperno, A. (2013). Nauty and traces
user’s guide (version 2.5). Computer Science Depart-
ment, Australian National University, Canberra, Aus-
tralia.
Nabi-Abdolyousefi, M. and Mesbahi, M. (2012). Network
identification via node knockout. IEEE Transactions
on Automatic Control, 57(12):3214–3219.
Olfati-Saber, R. and Murray, R. M. (2004). Consensus
problems in networks of agents with switching topol-
ogy and time-delays. IEEE Transactions on automatic
control, 49(9):1520–1533.
Ota, J. (2006). Multi-agent robot systems as distributed au-
tonomous systems. Advanced engineering informat-
ics, 20(1):59–70.
Rahimian, M. A., Ajorlou, A., Tutunov, R., and Aghdam,
A. G. (2013). Identifiability of links and nodes in
multi-agent systems under the agreement protocol.
In 2013 American Control Conference, pages 6853–
6858. IEEE.
Scharre, P. (2018). How swarming will change warfare.
Bulletin of the atomic scientists, 74(6):385–389.
Stanhope, S., Rubin, J. E., and Swigon, D. (2014). Identi-
fiability of linear and linear-in-parameters dynamical
systems from a single trajectory. SIAM Journal on
Applied Dynamical Systems, 13(4):1792–1815.
Sun, C. and Dai, R. (2015). Identification of network topol-
ogy via quadratic optimization. In 2015 American
Control Conference (ACC), pages 5752–5757. IEEE.
Tan, Y. and Zheng, Z. (2013). Research advance in swarm
robotics. Defence Technology, 9(1):18–39.
Teodorovic, D. (2003). Transport modeling by multi-agent
systems: a swarm intelligence approach. Transporta-
tion planning and Technology, 26(4):289–312.
The MathWorks, Inc. (2019). Deep Learning Toolbox,
MATLAB 2019a. Natick, Massachusetts, U.S.
Vald
´
es-Sosa, P. A., S
´
anchez-Bornot, J. M., Lage-
Castellanos, A., Vega-Hern
´
andez, M., Bosch-Bayard,
J., Melie-Garc
´
ıa, L., and Canales-Rodr
´
ıguez, E.
(2005). Estimating brain functional connectivity with
sparse multivariate autoregression. Philosophical
Transactions of the Royal Society B: Biological Sci-
ences, 360(1457):969–981.
van Waarde, H. J., Tesi, P., and Camlibel, M. K. (2019).
Topology reconstruction of dynamical networks via
constrained lyapunov equations. IEEE Transactions
on Automatic Control, 64(10):4300–4306.
Wu, X., Zhao, X., L
¨
u, J., Tang, L., and an Lu, J. (2015).
Identifying topologies of complex dynamical net-
works with stochastic perturbations. IEEE Transac-
tions on Control of Network Systems, 3(4):379–389.
APPENDIX
10
40
70
100
130
160
190
220
250
280
310
340
370
400
10
20
30
40
50
60
70
80
Number of Graphs Sampled
Mean Success (%)
Mean Success of 4-Node Trained Network for
Prediction of P.-S. Nodes of 8-Node Graph
Primary Node
Secondary Node
Figure 6: Results of testing a pattern-recognition network
trained on data from a small-scale (4-node) system on large-
scale (8-node) data.
25
100
175 250 325
400
10
20
30
40
50
60
70
80
Number of Graphs Sampled
Mean Success (%)
Mean Success of 4-Node Trained Network for
Prediction of P.-S. Nodes of 9-Node Graph
Primary Node
Secondary Node
Figure 7: Results of testing a pattern-recognition network
trained on data from a small-scale (4-node) system on large-
scale (9-node) data.
ICAART 2021 - 13th International Conference on Agents and Artificial Intelligence
264