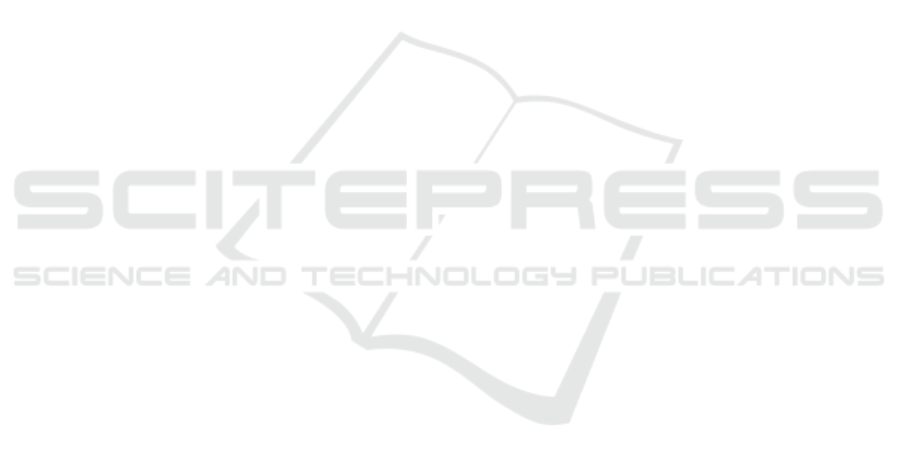
chical structure (shown in Fig.7), which may require a
long training period. To address this, we will look into
proposing new training methods that may improve the
efficiency in training the network.
REFERENCES
Agarwal, A., Hazan, E., Kale, S., and Schapire, R. E.
(2006). Algorithms for portfolio management based
on the newton method. In Proceedings of the 23rd
international conference on Machine learning, pages
9–16.
Borodin, A., El-Yaniv, R., and Gogan, V. (2004). Can we
learn to beat the best stock. In Advances in Neural
Information Processing Systems, pages 345–352.
Cover, T. M. (2011). Universal portfolios. In The Kelly Cap-
ital Growth Investment Criterion: Theory and Prac-
tice, pages 181–209. World Scientific.
Dempster, M. A. and Leemans, V. (2006). An automated fx
trading system using adaptive reinforcement learning.
Expert Systems with Applications, 30(3):543–552.
Faragher, R. (2012). Understanding the basis of the kalman
filter via a simple and intuitive derivation [lecture
notes]. IEEE Signal processing magazine, 29(5):128–
132.
Gao, Z., Gao, Y., Hu, Y., Jiang, Z., and Su, J. (2020). Appli-
cation of deep q-network in portfolio management. In
2020 5th IEEE International Conference on Big Data
Analytics (ICBDA), pages 268–275. IEEE.
Heaton, J., Polson, N. G., and Witte, J. H. (2016). Deep
learning in finance. arXiv preprint arXiv:1602.06561.
Helmbold, D. P., Schapire, R. E., Singer, Y., and Warmuth,
M. K. (1998). On-line portfolio selection using mul-
tiplicative updates. Mathematical Finance, 8(4):325–
347.
Huang, D., Zhou, J., Li, B., HOI, S., and Zhou, S. (2012).
Robust median reversion strategy for on-line portfo-
lio selection.(2013). In Proceedings of the Twenty-
Third International Joint Conference on Artificial In-
telligence: IJCAI 2013: Beijing, 3-9 August 2013.
Jiang, Z., Xu, D., and Liang, J. (2017). A deep re-
inforcement learning framework for the financial
portfolio management problem. arXiv preprint
arXiv:1706.10059.
Klambauer, G., Unterthiner, T., Mayr, A., and Hochre-
iter, S. (2017). Self-normalizing neural networks. In
Advances in neural information processing systems,
pages 971–980.
Kulkarni, T. D., Narasimhan, K., Saeedi, A., and Tenen-
baum, J. (2016). Hierarchical deep reinforcement
learning: Integrating temporal abstraction and intrin-
sic motivation. In Advances in neural information pro-
cessing systems, pages 3675–3683.
Li, B. and Hoi, S. C. (2014). Online portfolio selection: A
survey. ACM Computing Surveys (CSUR), 46(3):1–
36.
Li, B., Hoi, S. C., Sahoo, D., and Liu, Z.-Y. (2015). Moving
average reversion strategy for on-line portfolio selec-
tion. Artificial Intelligence, 222:104–123.
Li, B., Hoi, S. C., Zhao, P., and Gopalkrishnan, V. (2013).
Confidence weighted mean reversion strategy for on-
line portfolio selection. ACM Transactions on Knowl-
edge Discovery from Data (TKDD), 7(1):1–38.
Li, B., Zhao, P., Hoi, S. C., and Gopalkrishnan, V. (2012).
Pamr: Passive aggressive mean reversion strategy for
portfolio selection. Machine learning, 87(2):221–258.
Magdon-Ismail, M. and Atiya, A. F. (2004). Maximum
drawdown. Risk Magazine, 17(10):99–102.
Neuneier, R. (1998). Enhancing q-learning for optimal as-
set allocation. In Advances in neural information pro-
cessing systems, pages 936–942.
Park, S., Song, H., and Lee, S. (2019). Linear programing
models for portfolio optimization using a benchmark.
The European Journal of Finance, 25(5):435–457.
Rua, A. and Nunes, L. C. (2009). International comovement
of stock market returns: A wavelet analysis. Journal
of Empirical Finance, 16(4):632–639.
Sharpe, W. F. (1994). The sharpe ratio. Journal of portfolio
management, 21(1):49–58.
Van Hasselt, H., Guez, A., and Silver, D. (2015). Deep
reinforcement learning with double q-learning. arXiv
preprint arXiv:1509.06461.
Wang, Z., Schaul, T., Hessel, M., Hasselt, H., Lanctot, M.,
and Freitas, N. (2016). Dueling network architectures
for deep reinforcement learning. In International con-
ference on machine learning, pages 1995–2003.
ICAART 2021 - 13th International Conference on Agents and Artificial Intelligence
140