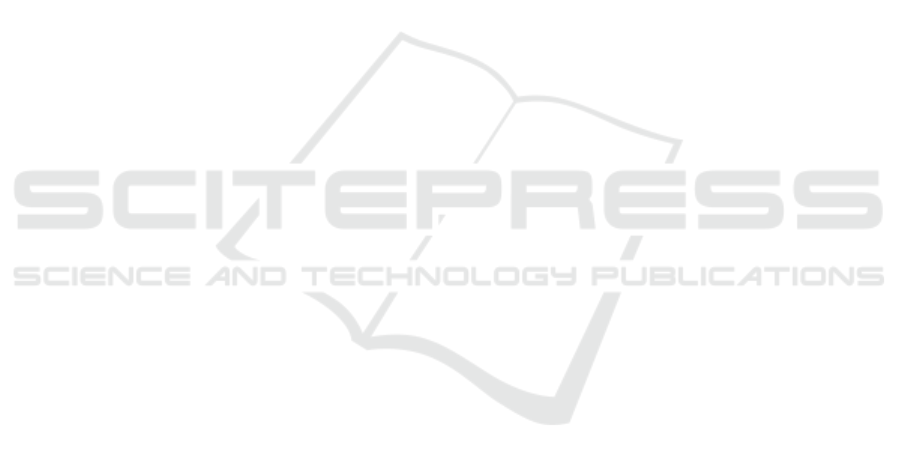
gain obtained by our approach will allow for
the usage of more complex network monitoring
features.
ACKNOWLEDGEMENTS
This work was funded by the Austrian Federal Min-
istry of Climate Action, Environment, Energy, Mobil-
ity, Innovation and Technology (BMK).
REFERENCES
Alshamrani, A., Myneni, S., Chowdhary, A., and Huang,
D. (2019). A Survey on Advanced Persistent Threats:
Techniques, Solutions, Challenges, and Research Op-
portunities. IEEE Communications Surveys Tutorials.
An, J. and Cho, S. (2015). Variational autoencoder based
anomaly detection using reconstruction probability.
Special Lecture on IE, 2:1–18.
Baddar, S. W. A.-H., Merlo, A., and Migliardi, M. (2014).
Anomaly detection in computer networks: A state-of-
the-art review. JoWUA, 5(4):29–64.
Chandola, V., Banerjee, A., and Kumar, V. (2009).
Anomaly Detection: A Survey. ACM computing sur-
veys (CSUR), 41(3):15.
Chen, J., Sathe, S., Aggarwal, C., and Turaga, D. (2017).
Outlier detection with autoencoder ensembles. In Pro-
ceedings of the 2017 SIAM International Conference
on Data Mining, pages 90–98. SIAM.
Duessel, P., Gehl, C., Flegel, U., Dietrich, S., and
Meier, M. (2017). Detecting zero-day attacks using
context-aware anomaly detection at the application-
layer. International Journal of Information Security,
16(5):475–490.
Fraley, J. B. and Cannady, J. (2017). The promise of
machine learning in cybersecurity. In SoutheastCon,
2017, pages 1–6. IEEE.
Ghafir, I., Hammoudeh, M., Prenosil, V., Han, L., Hegarty,
R., Rabie, K., and Aparicio-Navarro, F. J. (2018). De-
tection of advanced persistent threat using machine-
learning correlation analysis. Future Generation
Computer Systems, 89:349–359.
Hindy, H., Brosset, D., Bayne, E., Seeam, A., Tachtatzis,
C., Atkinson, R., and XavierBellekens (2018). A tax-
onomy and survey of intrusion detection systemdesign
techniques, network threats and datasets. Association
for Computing Machinery.
Hodo, E., Bellekens, X., Hamilton, A., Tachtatzis, C., and
Atkinson, R. (2017). Shallow and deep networks in-
trusion detection system: A taxonomy and survey.
arXiv preprint arXiv:1701.02145.
Javaid, A., Niyaz, Q., Sun, W., and Alam, M. (2016).
A deep learning approach for network intrusion de-
tection system. In Proceedings of the 9th EAI In-
ternational Conference on Bio-inspired Information
and Communications Technologies (formerly BIO-
NETICS), pages 21–26. ICST.
Kwon, D., Kim, H., Kim, J., Suh, S.-c., Kim, I., and Kim,
K. (2017). A survey of deep learning-based network
anomaly detection. Cluster Computing, pages 1–13.
Pawlicki, M., Chora
´
s, M., Kozik, R., and Hołubowicz, W.
(2020). On the impact of network data balancing in
cybersecurity applications. In International Confer-
ence on Computational Science, pages 196–210.
Ravi Kiran, M. T. and Parakkal, R. (2018). An overview
of deep learning based methods for unsupervised and
semi-supervised anomaly detection in videos. arXiv
preprint arXiv:1801.03149.
Ring, M., Wunderlich, S., Scheuring, D., Landes, D., and
Hotho, A. (2019). A survey of network-based in-
trusion detection data sets. Computers & Security,
86:147–167.
Schneider, P. and B
¨
ottinger, K. (2018). High-performance
unsupervised anomaly detection for cyber-physical
system networks. In CPS-SPC@CCS.
Sharafaldin, I., Lashkari, A. H., and Ghorbani, A. A.
(2018). Toward generating a new intrusion detection
dataset and intrusion traffic characterization. In 4th
International Conference on Information Systems Se-
curity and Privacy (ICISSP).
Stojanovi
´
c, B., Hofer-Schmitz, K., and Kleb, U. (2020).
Apt datasets and attack modeling for automated de-
tection methods: A review. Computers & Security,
92:101734.
Tavallaee, M., Bagheri, E., Lu, W., and Ghorbani, A. A.
(2009). A Detailed Analysis of the KDD CUP 99 Data
Set. In Proceedings of the 2009 IEEE Symposium on
Computational Intelligence.
Tuor, A., Kaplan, S., Hutchinson, B., Nichols, N., and
Robinson, S. (2017). Deep learning for unsuper-
vised insider threat detection in structured cybersecu-
rity data streams. arXiv preprint arXiv:1710.00811.
Xin, Y., Kong, L., Liu, Z., Chen, Y., Li, Y., Zhu, H., Gao,
M., Hou, H., and Wang, C. (2018). Machine learning
and deep learning methods for cybersecurity. IEEE
Access, 6:35365–35381.
Y
¨
uksel,
¨
O., den Hartog, J., and Etalle, S. (2016). Reading
between the fields: practical, effective intrusion detec-
tion for industrial control systems. In Proceedings of
the 31st Annual ACM Symposium on Applied Comput-
ing, pages 2063–2070. ACM.
Zavrak, S. and Iskefiyeli, M. (2020). Anomaly-Based In-
trusion Detection From Network Flow Features Us-
ing Variational Autoencoder. IEEE Access, 8:108346–
108358.
Two Stage Anomaly Detection for Network Intrusion Detection
457