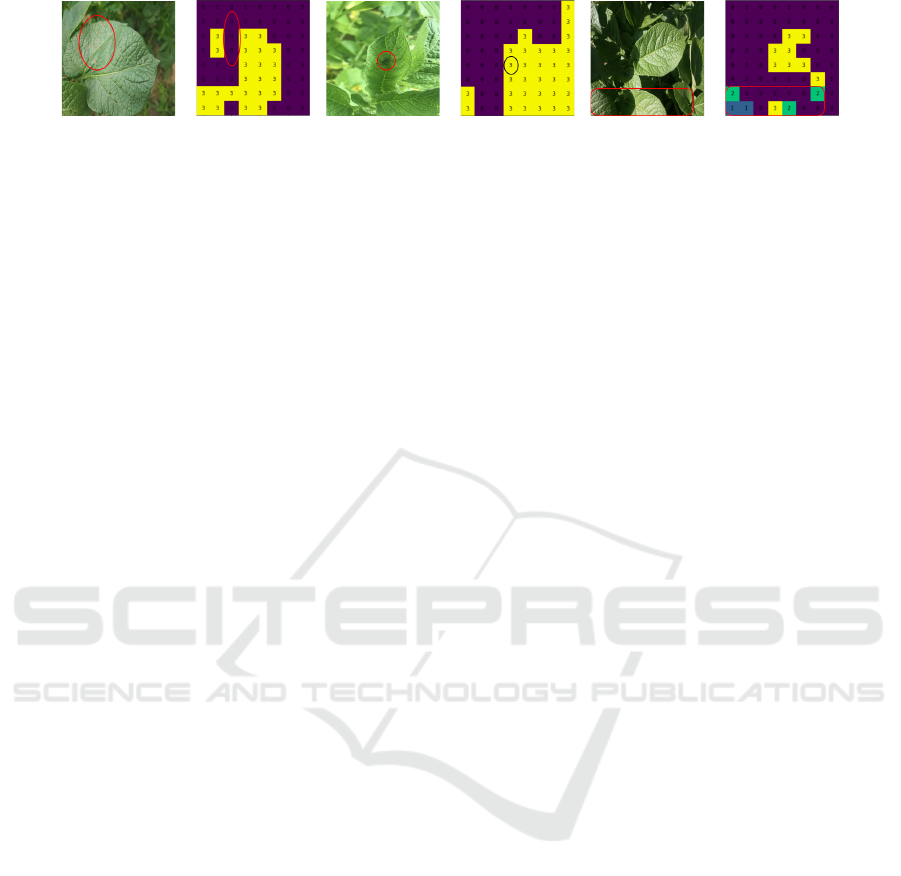
(a) Early Blight (b) Late Blight (c) Healthy Leaves
Figure 5: Misinterpretation of patches due to defocus and/or uneven illumination and/or unnoticeable disease spots. First,
third and fifth are original images ; second, fourth and sixth images are corresponding patch label maps. (Patch labels - 0:
background, 1: early blight, 2: late blight and 3: healthy.)
spots are intelligible in image, 4) in case of multiple
spots of varying sizes on a cluster of leaves, multi-
ple images must be captured and 5) samples of a par-
ticular disease must be labelled as per their maturity
phase, as appearance of disease spots differs widely
with time. These properties are expected to provide
clarity to region of interest and to ensure sufficient
class separability of the sample images. A robust seg-
mentation algorithm is also required to be devised to
extract a single leaf with disease spots or a healthy
leaf only from the images captured in field.
Our observations suggest that, classification accu-
racy alone is not a good performance metric for this
type of systems. We deduce that, quantitative and
qualitative measure of features learnt by the models
can only establish the trustworthiness of such models
with some guarantee. We are carrying out an exhaus-
tive analysis of features learnt by the models to prove
our hypothesis that features represent the overall or-
ganisation of an image rather than leaf and disease
region alone.
ACKNOWLEDGEMENTS
This research work is a part of the project Farmer-
Zone, sponsored by Department of Biotechnology,
Govt. of India. We thank Central Potato Research In-
stitute (CPRI), Shimla, India for providing the dataset
from field.
REFERENCES
Deng, J., Dong, W., Socher, R., Li, L., Kai Li, and Li Fei-
Fei (2009). Imagenet: A large-scale hierarchical im-
age database. In 2009 IEEE Conference on Computer
Vision and Pattern Recognition, pages 248–255.
Kaur, S., Pandey, S., and Goel, S. (2018). Plants disease
identification and classification through leaf images:
A survey. Archives of Computational Methods in En-
gineering, 26:507–530.
Krizhevsky, A., Sutskever, I., and Hinton, G. E. (2012). Im-
agenet classification with deep convolutional neural
networks. In Advances in Neural Information Pro-
cessing Systems 25, pages 1097–1105.
Liu, W., Anguelov, D., Erhan, D., Szegedy, C., Reed, S., Fu,
C.-Y., and Berg, A. (2016). Ssd: Single shot multibox
detector. volume 9905, pages 21–37.
Martinelli, F., Scalenghe, R., Davino, S., Panno, S., Scud-
eri, G., Ruisi, P., Villa, P., Stroppiana, D., Boschetti,
M., Goulart, L. R., Davis, C. E., and Dandekar, A. M.
(2015). Advanced methods of plant disease detection.
a review. Agron. Sustain. Dev., 35:1–25.
Mohanty, S. P., Hughes, D. P., and Salath
´
e, M. (2016). Us-
ing deep learning for image-based plant disease detec-
tion. Front. Plant Sci., 7:1419.
Ngugi, L. C., Abelwahab, M., and Abo-Zahhad, M. (2020).
Recent advances in image processing techniques for
automated leaf pest and disease recognition – a review.
Information Processing in Agriculture.
Ren, S., He, K., Girshick, R., and Sun, J. (2015). Faster
r-cnn: Towards real-time object detection with region
proposal networks. In Cortes, C., Lawrence, N. D.,
Lee, D. D., Sugiyama, M., and Garnett, R., editors,
Advances in Neural Information Processing Systems
28, pages 91–99. Curran Associates, Inc.
Saleem, M. H., Potgieter, J., and Arif, K. M. (2019). Plant
disease detection and classification by deep learning.
Plants, 8.
Simonyan, K. and Zisserman, A. (2015). Very deep con-
volutional networks for large-scale image recognition.
CoRR, abs/1409.1556.
Szegedy, C., Wei Liu, Yangqing Jia, Sermanet, P., Reed, S.,
Anguelov, D., Erhan, D., Vanhoucke, V., and Rabi-
novich, A. (2015). Going deeper with convolutions. In
2015 IEEE Conference on Computer Vision and Pat-
tern Recognition (CVPR), pages 1–9.
Thurston, H. and Schultz, O. (1981). Late blight. Com-
pendium of Potato Diseases, pages 41–42.
Weingartner, D. (1981). Early blight. Compendium of
Potato Diseases, pages 43–44.
ICPRAM 2021 - 10th International Conference on Pattern Recognition Applications and Methods
522