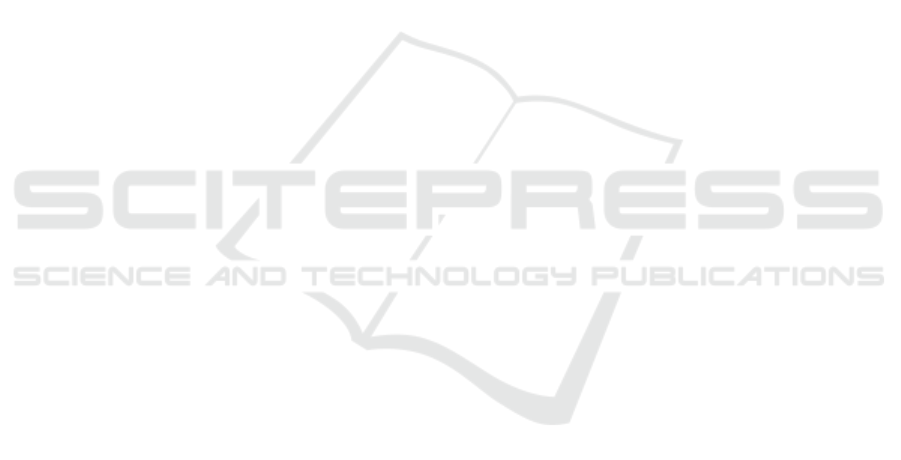
The DT purpose may also change due to the current
life-cycle stage of the system or the maturity of the DT
itself.
Similar to our work, (Uhlenkamp et al., 2019)
present seven characteristics of DTs. Goals refer to
the abilities of the DT and include information ac-
quisition, information analysis, decision and action
selection and action implementation. Users focus on
one user or multiple stakeholders. Life-cycle focus
can likewise be a single stage or multiple. A system
focus can be component, subsystem, system, or system
of systems. Data sources for a DT include measure-
ments, virtual data (including simulation data), and
(expert) knowledge. Data integration levels replace
the classification of (Kritzinger et al., 2018) with man-
ual, semi-automated, and automated. Finally, their
characteristics include authenticity which we refer to
in our conceptual structure as fidelity. While relevant,
we demonstrate in Section 2 that further characteris-
tics and detail may be required in current experience
reports to apply their structure.
According to (Madni et al., 2019), DTs are an en-
abling technology in the next steps of model-based
system engineering (MBSE). A comprehensive exami-
nation of DTs within the context of MBSE is presented,
along with a classification of DT maturity through-
out the life-cycle of the system of pre-DT, DT, adap-
tive DT, and intelligent DT. Relevant to our work is
a list of characteristics which separates DT from tra-
ditional computer-assisted design (CAD) models, in-
cluding specificity, understanding assumptions and
performance, and traceability among others.
(van der Valk et al., 2020) propose a DT taxonomy
with characteristics and relationships, while (Jones
et al., 2020) details a literature survey. These works
propose further characteristics of DTs, but do not cre-
ate a conceptual structuring.
6 CONCLUSION
This paper presents a conceptual structure for describ-
ing digital twins (DTs) such that practitioners can struc-
ture their reporting and ensure they describe essential
characteristics and capabilities in their experience re-
ports. In turn, this provides a firmer foundation for
the DT research community to gain further insights
into challenges and solutions, and offer a more precise
classification of the DT types in practice. It is our
ambition that this structure serves as a common refer-
ence to enable clear communication of DT solutions
between academic and industrial stakeholders. The
applicability of the structuring and its use in structur-
ing a report and indicating missing characteristics has
been demonstrated by expressing multiple experience
reports from the literature. This has revealed six cases
where the suggested classification differs from that of
others (Oakes et al., 2020).
ACKNOWLEDGEMENTS
This work was partly funded by Flanders Make vzw,
the strategic research centre for the Flemish manufac-
turing industry.
REFERENCES
Boschert, S. and Rosen, R. (2016). Digital twin—the sim-
ulation aspect. In Mechatronic Futures, pages 59–74.
Springer.
Bottani, E., Cammardella, A., et al. (2017). From the cyber-
physical system to the digital twin: the process de-
velopment for behaviour modelling of a cyber guided
vehicle in m2m logic. XXII Summer School Francesco
Turco – Industrial Systems Engineering, pages 1–7.
Chhetri, S. R., Faezi, S., et al. (2019). QUILT: quality
inference from living digital twins in iot-enabled man-
ufacturing systems. In Proceedings of International
Conference on Internet of Things Design and Imple-
mentation, pages 237–248. ACM.
Fuller, A., Fan, Z., et al. (2020). Digital twin: Enabling
technologies, challenges and open research. IEEE
Access, 8:108952–108971.
Grieves, M. and Vickers, J. (2017). Digital twin: Mitigat-
ing unpredictable, undesirable emergent behavior in
complex systems. In Transdisciplinary perspectives on
complex systems, pages 85–113. Springer.
Jones, D., Snider, C., et al. (2020). Characterising the digital
twin: A systematic literature review. CIRP Journal of
Manufacturing Science and Technology, 29:36–52.
Kritzinger, W., Karner, M., et al. (2018). Digital twin in man-
ufacturing: A categorical literature review and classifi-
cation. IFAC-PapersOnLine, 51(11):1016–1022.
Leinen, R. (2017). Driving the digital enterprise in prod-
uct development and manufacturing. Presented at 6th
CSIR Conference.
Liu, Y., Zhang, L., et al. (2019). A novel cloud-based frame-
work for the elderly healthcare services using digital
twin. IEEE Access, 7:49088–49101.
Madni, A. M., Madni, C. C., and Lucero, S. D. (2019).
Leveraging digital twin technology in model-based
systems engineering. Systems, 7(1):7.
Malik, A. A. and Bilberg, A. (2018). Digital twins of human
robot collaboration in a production setting. Procedia
manufacturing, 17:278–285.
Mayani, M. G., Svendsen, M., and Oedegaard, S. I. (2018).
Drilling digital twin success stories the last 10 years. In
SPE Norway One Day Seminar, pages 1–13. Society of
Petroleum Engineers, Society of Petroleum Engineers.
Improving Digital Twin Experience Reports
189