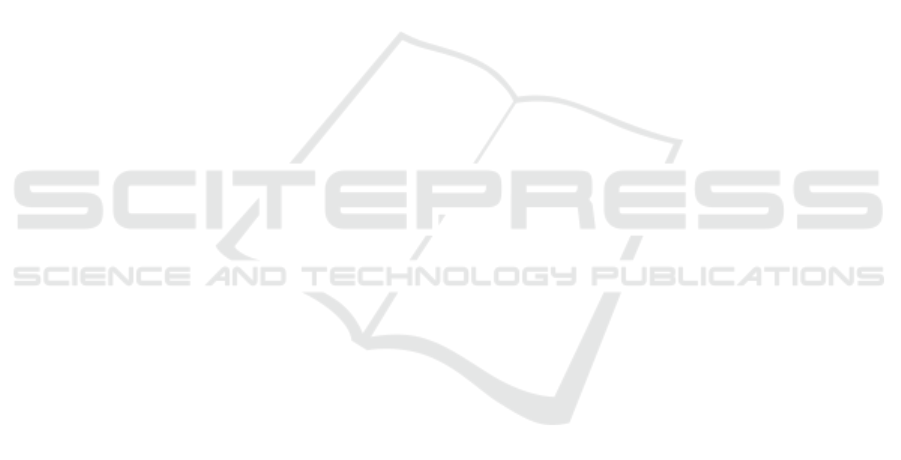
get stuck without entering the roundabout, thus caus-
ing unrealistic congestions. Taking GTFS data into
account, MATSim multimodal network offers a more
realistic public transport supply. Integration of GTFS
data into SUMO are still in progress.
MATSim, thanks to its activity and agent-based ap-
proach, allows better modeling intermodal mobility
behaviors by taking into account, for example, the trip
purpose, the chains of daily activities, and some at-
tributes of the user such as car availability. The modal
choice is also based on a utility function that can con-
sider several criteria such as travel time and cost. This
choice can be performed upstream of the simulation
through a pairing with a discrete choice model (H
¨
orl
et al., 2019). However, due to a high abstraction level,
MATSim does not simulate microscopic interaction as
the pedestrian crossing. SUMO takes advantage if the
studies focus on a specific multimodal area such as
a town square with a train station, buses, bikes, car
parking plot. In addition, through the MATSim loop,
it is possible to better assess the travel behavior of
agents who, thanks to replanning, can modify their
habits. This is quite interesting because one can imag-
ine new users learning to define the routes as they
discover the road network and the state of the traf-
fic. SUMO also has an iteration module (dua-iterate),
but it only relies on travel times to make the choice of
transportation mode.
5 CONCLUSION
In this paper we evaluated different simulation plat-
forms with the aim of choosing the most suitable to
simulate intermodality policies. The paper first pre-
sented a state of the art of traffic simulators compar-
ison. These works can be divided into two groups
depending on the evaluation method used: qualitative
or quantitative. Based on these works, we proposed a
new comparison approach that is both qualitative and
quantitative and which takes into account criteria spe-
cific to the modeler. It consists first in evaluating the
simulators on their ability to meet the evaluation crite-
ria. From this step, a shortlist is retained for a deeper
study. Finally, the paper presented a case of study
where we evaluated five simulators: four specific to
traffic and one generic. We retained SUMO and MAT-
Sim and compared their capabilities to simulate the
intermodal mobility behaviors of a given population.
MATSim was selected as the most appropriate simula-
tion tool to reproduce intermodal mobility behaviors
on large scale.
As future works, we plan to focus our studies on
MATSim, working on embedding an intermodal mode
choice module. The aim will be to estimate the pa-
rameters of this model from actual mobility data such
as HTS and then to implement it inside MATSim.
REFERENCES
Ejercito, P. M., Nebrija, K. G. E., Feria, R. P., and Lara-
Figueroa, L. L. (2017). Traffic simulation software
review. In 8th Int. Conf. on Information, Intelligence,
Systems & Applications (IISA), pages 1–4.
Ghariani, N., Elkosantini, S., Darmoul, S., and Said, L. B.
(2014). A survey of simulation platforms for the
assessment of public transport control systems. In
14th Int. Conf. on Advanced Logistics and Transport
(ICALT), pages 85–90.
Grignard, A., Taillandier, P., Gaudou, B., Vo, D. A., Huynh,
N. Q., and Drogoul, A. (2013). Gama 1.6: Advancing
the art of complex agent-based modeling and simu-
lation. In PRIMA: Principles and Practice of Multi-
Agent Systems, pages 117–131.
H
¨
orl, S., Bala
´
c, M., and Axhausen, K. W. (2019). Pairing
discrete mode choice models and agent-based trans-
port simulation with matsim. In 2019 TRB Annual
Meeting Online.
Horni, A., Nagel, K., and Axhausen, K., editors (2016).
Multi-Agent Transport Simulation MATSim. Ubiquity
Press, London.
Li, J., Doniec, A., Boonaert, J., and Lozenguez, G. (2018).
Which traffic simulator is suitable for customized be-
haviors in multi-modal scenarios. In 21st Int. Conf.
on Intelligent Transportation Systems (ITSC), pages
3791–3796.
Lopez, P. A., Behrisch, M., Bieker-Walz, L., Erdmann, J.,
Fl
¨
otter
¨
od, Y.-P., Hilbrich, R., L
¨
ucken, L., Rummel, J.,
Wagner, P., and Wießner, E. (2018). Microscopic traf-
fic simulation using sumo. In 21st IEEE Int. Conf. on
Intelligent Transportation Systems (ITSC).
Mandiau, R., Champion, A., Auberlet, J., Espi
´
e, S., and
Kolski, C. (2008). Behaviour based on decision matri-
ces for a coordination between agents in a urban traffic
simulation. Appl. Intell., 28(2):121–138.
Mathieu, P., Morvan, G., and Picault, S. (2018). Multi-level
agent-based simulations: Four design patterns. Simul.
Model. Pract. Theory, 83:51–64.
Neumann, A. and Zilske, M. (2018). MATSim JOSMNet-
work Editor. Technische Universit
¨
at Berlin.
Poletti, F., B
¨
osch, P. M., Ciari, F., and Axhausen, K. W.
(2017). Public transit route mapping for large-scale
multimodal networks. ISPRS International Journal of
Geo-Information, 6(9):268.
Pursula, M. (1999). Simulation of traffic systems-an
overview. Journal of geographic information and de-
cision analysis, 3(1):1–8.
Saidallah, M., El Fergougui, A., and Elalaoui, A. E. (2016).
A comparative study of urban road traffic simulators.
In MATEC Web of Conferences, Vol. 81. EDP Sci-
ences.
Xiao, H., Ambadipudi, R., Hourdakis, J., and Michalopou-
los, P. (2005). Methodology for selecting microscopic
simulators: Comparative evaluation of aimsun and
vissim. Technical report, Intelligent Transportation
Systems Institute, University of Minnesota.
ICAART 2021 - 13th International Conference on Agents and Artificial Intelligence
272