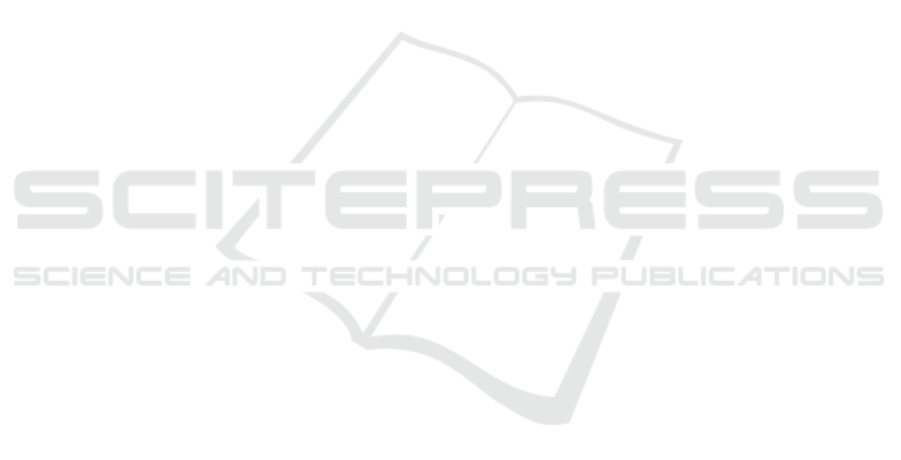
proper edges to base the segmentation on.
5 CONCLUSIONS
This paper proposed a monocular vision based driv-
able area segmentation pipeline that was able to de-
tect the drivable area with and without the presence
of shadows in the scene in different situations without
any additional sensors. The CV-IM pipeline proved
to be robust and surpassed a DL network in the pro-
cess. In addition, the use of the output image from
the pipeline as an input to the DL model significantly
improved the prediction compared to the normal gray
scale image. Moreover, the system is independent
from the use of any maps and is a plug-and-play one.
Future work can include making the pipeline more ro-
bust to work in more challenging situations as well as
adding more modules such as object detection.
REFERENCES
Bai, M., Mattyus, G., Homayounfar, N., Wang, S., Lak-
shmikanth, S. K., and Urtasun, R. (2018). Deep
multi-sensor lane detection. In IEEE/RSJ Interna-
tional Conference on Intelligent Robots and Systems
(IROS), pages 3102–3109.
Fan, H., Xie, F., Li, Y., Jiang, Z., and Liu, J. (2017).
Automatic segmentation of dermoscopy images using
saliency combined with otsu threshold. Computers in
biology and medicine, 85:75–85.
Gevers, T. and Smeulders, A. W. (1999). Color-based object
recognition. Pattern recognition, 32(3):453–464.
Gurghian, A., Koduri, T., Bailur, S. V., Carey, K. J., and
Murali, V. N. (2016). Deeplanes: End-to-end lane po-
sition estimation using deep neural networksa. In Pro-
ceedings of the IEEE Conference on Computer Vision
and Pattern Recognition Workshops, pages 38–45.
Haque, M. R., Islam, M. M., Alam, K. S., Iqbal, H., and
Shaik, M. E. (2019). A computer vision based lane
detection approach. International Journal of Image,
Graphics and Signal Processing, 11(3):27.
Hata, A. and Wolf, D. (2014). Road marking detection us-
ing lidar reflective intensity data and its application to
vehicle localization. In 17th International IEEE Con-
ference on Intelligent Transportation Systems (ITSC),
pages 584–589.
Hou, Y. (2019). Agnostic lane detection. arXiv preprint
arXiv:1905.03704.
Katramados, I., Crumpler, S., and Breckon, T. P. (2009).
Real-time traversable surface detection by colour
space fusion and temporal analysis. In International
Conference on Computer Vision Systems, pages 265–
274. Springer.
Kim, Z. (2008). Robust lane detection and tracking in chal-
lenging scenarios. IEEE Transactions on Intelligent
Transportation Systems, 9(1):16–26.
Levi, D., Garnett, N., Fetaya, E., and Herzlyia, I. (2015).
Stixelnet: A deep convolutional network for obsta-
cle detection and road segmentation. In BMVC, pages
109–1.
Miksik, O., Petyovsky, P., Zalud, L., and Jura, P. (2011).
Robust detection of shady and highlighted roads for
monocular camera based navigation of ugv. In 2011
IEEE International Conference on Robotics and Au-
tomation, pages 64–71. IEEE.
Mishra, A. and Chourasia, B. (2017). Modified hue over
intensity ratio based method for shadow detection and
removal in arial images. INTERNATIONAL JOUR-
NAL OF ADVANCED ENGINEERING AND MAN-
AGEMENT, 2:101.
Montabone, S. and Soto, A. (2010). Human detection using
a mobile platform and novel features derived from a
visual saliency mechanism. Image and Vision Com-
puting, 28(3):391–402.
Neto, A. M., Victorino, A. C., Fantoni, I., and Ferreira, J. V.
(2013). Real-time estimation of drivable image area
based on monocular vision. In IEEE Intelligent Ve-
hicles Symposium Workshops (IV Workshops), pages
63–68.
Otsu, N. (1979). A threshold selection method from gray-
level histograms. IEEE Transactions on Systems,
Man, and Cybernetics, 9(1):62–66.
Qiang, Z., Tu, S., and Xu, L. (2019). A k-dense-unet
for biomedical image segmentation. In International
Conference on Intelligent Science and Big Data Engi-
neering, pages 552–562. Springer.
Suzuki, S. et al. (1985). Topological structural analy-
sis of digitized binary images by border following.
Computer vision, graphics, and image processing,
30(1):32–46.
Drivable Area Extraction based on Shadow Corrected Images
767