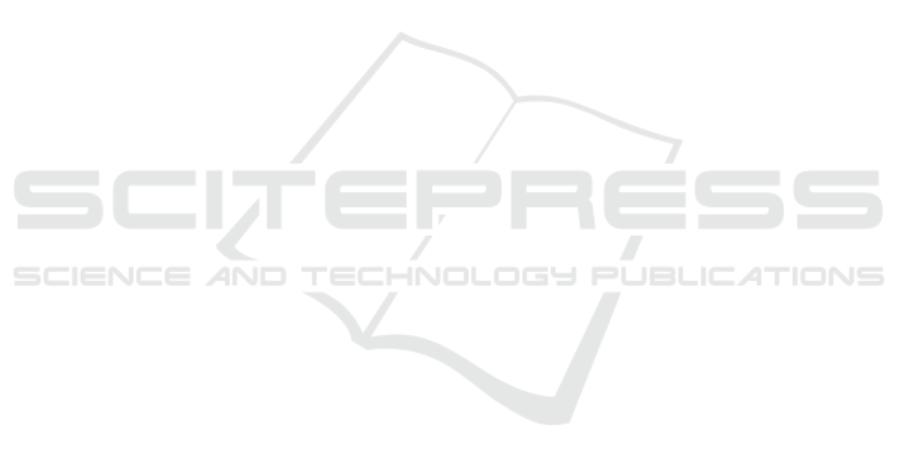
Our method supports only numerical input.
Therefore to improve the range of application, it must
be extended to the mixed data input: numerical and
categorical data. Although our method shows high fi-
delity in the experiment, coverage is still lower than
those of earlier methods so that improving coverage
is an important task.It remains a global explanation of
a black-box model.
ACKNOWLEDGEMENTS
This work was partially supported by JSPS Kakenhi
20H04143 and 17K00002.
REFERENCES
Aivodji, U., Arai, H., Fortineau, O., Gambs, S., Hara, S.,
and Tapp, A. (2019). Fairwashing: the risk of ra-
tionalization. volume 97 of Proceedings of Machine
Learning Research, pages 161–170, Long Beach, Cal-
ifornia, USA. PMLR.
Angelino, E., Larus-Stone, N., Alabi, D., Seltzer, M., and
Rudin, C. (2017). Learning certifiably optimal rule
lists. In Proceedings of the 23rd ACM SIGKDD In-
ternational Conference on Knowledge Discovery and
Data Mining, pages 35–44. ACM.
Caruana, R., Lou, Y., Gehrke, J., Koch, P., Sturm, M., and
Elhadad, N. (2015). Intelligible models for healthcare:
Predicting pneumonia risk and hospital 30-day read-
mission. In Proceedings of the 21th ACM SIGKDD In-
ternational Conference on Knowledge Discovery and
Data Mining, pages 1721–1730. ACM.
Dash, S., Gunluk, O., and Wei, D. (2018). Boolean decision
rules via column generation. In Advances in Neural
Information Processing Systems, pages 4655–4665.
Guidotti, R., Monreale, A., Ruggieri, S., Pedreschi, D.,
Turini, F., and Giannotti, F. (2018a). Local rule-based
explanations of black box decision systems. arXiv
preprint arXiv:1805.10820.
Guidotti, R., Monreale, A., Ruggieri, S., Turini, F., Gian-
notti, F., and Pedreschi, D. (2018b). A survey of meth-
ods for explaining black box models. ACM Computing
Surveys (CSUR), 51(5):93.
Kaufmann, E. and Kalyanakrishnan, S. (2013). Information
complexity in bandit subset selection. In Conference
on Learning Theory, pages 228–251.
Lakkaraju, H., Aguiar, E., Shan, C., Miller, D., Bhanpuri,
N., Ghani, R., and Addison, K. L. (2015). A ma-
chine learning framework to identify students at risk
of adverse academic outcomes. In Proceedings of
the 21th ACM SIGKDD international conference on
knowledge discovery and data mining, pages 1909–
1918. ACM.
Lakkaraju, H., Bach, S. H., and Leskovec, J. (2016). Inter-
pretable decision sets: A joint framework for descrip-
tion and prediction. In Proceedings of the 22nd ACM
SIGKDD international conference on knowledge dis-
covery and data mining, pages 1675–1684. ACM.
Laugel, T., Lesot, M.-J., Marsala, C., Renard, X., and De-
tyniecki, M. (2019). The dangers of post-hoc inter-
pretability: Unjustified counterfactual explanations.
In Proceedings of the Twenty-Eighth International
Joint Conference on Artificial Intelligence, IJCAI-19,
pages 2801–2807. International Joint Conferences on
Artificial Intelligence Organization.
Lundberg, S. M. and Lee, S.-I. (2017). A unified approach
to interpreting model predictions. In Advances in neu-
ral information processing systems, pages 4765–4774.
Ribeiro, M. T., Singh, S., and Guestrin, C. (2016). Why
should i trust you?: Explaining the predictions of any
classifier. In Proceedings of the 22nd ACM SIGKDD
international conference on knowledge discovery and
data mining, pages 1135–1144. ACM.
Ribeiro, M. T., Singh, S., and Guestrin, C. (2018). An-
chors: High-precision model-agnostic explanations.
In AAAI, pages 1527–1535.
Rudin, C. (2019). Stop explaining black box machine learn-
ing models for high stakes decisions and use inter-
pretable models instead. Nature Machine Intelligence,
1(5):206–215.
Rudin, C. and Shaposhnik, Y. (2019). Globally-consistent
rule-based summary-explanations for machine learn-
ing models: Application to credit-risk evaluation.
SSRN Electronic Journal.
Tsai, C.-F., Eberle, W., and Chu, C.-Y. (2013). Ge-
netic algorithms in feature and instance selection.
Knowledge-Based Systems, 39:240–247.
Wang, F. and Rudin, C. (2015). Falling rule lists. In Artifi-
cial Intelligence and Statistics, pages 1013–1022.
Wang, T. (2018). Multi-value rule sets for interpretable
classification with feature-efficient representations. In
Bengio, S., Wallach, H., Larochelle, H., Grauman, K.,
Cesa-Bianchi, N., and Garnett, R., editors, Advances
in Neural Information Processing Systems 31, pages
10835–10845. Curran Associates, Inc.
ICAART 2021 - 13th International Conference on Agents and Artificial Intelligence
774