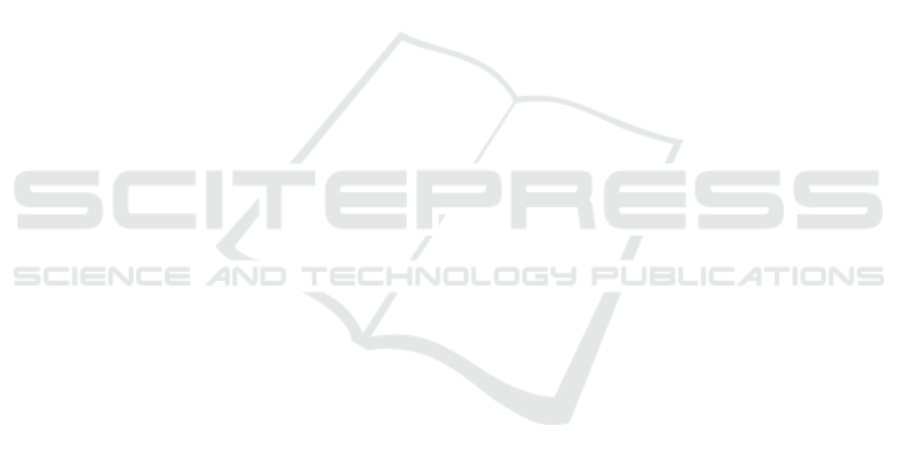
International Conference on Robotics and Automation
(ICRA), pages 3832–3838.
Hansen, M. K. and Underwood, J. P. (2017). Multi-modal
obstacle detection in unstructured environments with
conditional random fields. CoRR, abs/1706.02908.
John, V. and Mita, S. (2019). RVNet: Deep Sensor Fusion
of Monocular Camera and Radar for Image-Based Ob-
stacle Detection in Challenging Environments. In Lec-
ture Notes in Computer Science (including subseries
Lecture Notes in Artificial Intelligence and Lecture
Notes in Bioinformatics), volume 11854 LNCS, pages
351–364.
Li, G., Yang, Y., and Qu, X. (2020). Deep Learn-
ing Approaches on Pedestrian Detection in Hazy
Weather. IEEE Transactions on Industrial Electron-
ics, 67(10):8889–8899.
Lin, T., Maire, M., Belongie, S. J., et al. (2014). Mi-
crosoft COCO: common objects in context. In Com-
puter Vision - ECCV 2014 - 13th European Confer-
ence, Zurich, Switzerland, September 6-12, 2014, Pro-
ceedings, Part V, volume 8693 of Lecture Notes in
Computer Science, pages 740–755. Springer.
Liu, Z., Yu, S., Wang, X., and Zheng, N. (2017). Detecting
drivable area for self-driving cars: An unsupervised
approach. CoRR, abs/1705.00451.
Long, J., Shelhamer, E., and Darrell, T. (2015). Fully Con-
volutional Networks for Semantic Segmentation. In
Proceedings of the IEEE Conference on Computer Vi-
sion and Pattern Recognition (CVPR).
Maag, K., Rottmann, M., and Gottschalk, H. (2020). Time-
Dynamic Estimates of the Reliability of Deep Seman-
tic Segmentation Networks. In IEEE International
Conference on Tools with Artificial Intelligence (IC-
TAI).
Nobis, F., Geisslinger, M., Weber, M., et al. (2019). A
deep learning-based radar and camera sensor fusion
architecture for object detection. In 2019 Sensor Data
Fusion: Trends, Solutions, Applications, SDF 2019,
Bonn, Germany, October 15-17, 2019, pages 1–7.
IEEE.
Phillips, J. (2020). Achieving Safe Autonomous
Driving. Available: https://www.ni.com/de-
de/perspectives/imminent-trade-offs-for-achieving-
safe-autonomous-driving.html.
Pidurkar, A., Sadakale, R., and Prakash, A. K. (2019).
Monocular camera based computer vision system for
cost effective autonomous vehicle. In 2019 10th Inter-
national Conference on Computing, Communication
and Networking Technologies (ICCCNT), pages 1–5.
P
´
erez, R., Schubert, F., Rasshofer, R., and Biebl, E. (2019).
A machine learning joint lidar and radar classification
system in urban automotive scenarios. Advances in
Radio Science, 17:129–136.
Redmon, J. and Farhadi, A. (2018). Yolov3: An incremental
improvement. CoRR, abs/1804.02767.
Rong, G., Shin, B. H., Tabatabaee, H., et al. (2020). LGSVL
simulator: A high fidelity simulator for autonomous
driving. CoRR, abs/2005.03778.
Rottmann, M., Colling, P., Hack, T. P., Chan, R., H
¨
uger,
F., Schlicht, P., and Gottschalk, H. (2020). Predic-
tion Error Meta Classification in Semantic Segmenta-
tion: Detection via Aggregated Dispersion Measures
of Softmax Probabilities. In 2020 IEEE International
Joint Conference on Neural Networks (IJCNN).
Rottmann, M. and Schubert, M. (2019). Uncertainty Mea-
sures and Prediction Quality Rating for the Seman-
tic Segmentation of Nested Multi Resolution Street
Scene Images. In Proceedings of the IEEE/CVF Con-
ference on Computer Vision and Pattern Recognition
(CVPR) Workshops.
Schneider, M. (2005). Automotive radar: Status and trends.
In In Proceedings of the German Microwave Confer-
ence GeMIC 2005, pages 144–147.
Schubert, M., Kahl, K., and Rottmann, M. (2020).
Metadetect: Uncertainty quantification and predic-
tion quality estimates for object detection. CoRR,
abs/2010.01695v2.
Silva, V. D., Roche, J., and Kondoz, A. M. (2018). Ro-
bust fusion of lidar and wide-angle camera data for
autonomous mobile robots. Sensors, 18(8):2730.
Tumas, P., Nowosielski, A., and Serackis, A. (2020). Pedes-
trian Detection in Severe Weather Conditions. IEEE
Access, 8:62775–62784.
Wang, Z., Wu, Y., and Niu, Q. (2020). Multi-sensor fu-
sion in automated driving: A survey. IEEE Access,
8:2847–2868.
Wu, T.-E., Tsai, C.-C., and Guo, J.-I. (2017). Li-
DAR/camera sensor fusion technology for pedestrian
detection. In 2017 Asia-Pacific Signal and Informa-
tion Processing Association Annual Summit and Con-
ference (APSIPA ASC), pages 1675–1678.
Xiao, Y., Jiang, A., Ye, J., and Wang, M.-W. (2020). Mak-
ing of Night Vision: Object Detection Under Low-
Illumination. IEEE Access, 8:123075–123086.
ICAART 2021 - 13th International Conference on Agents and Artificial Intelligence
186