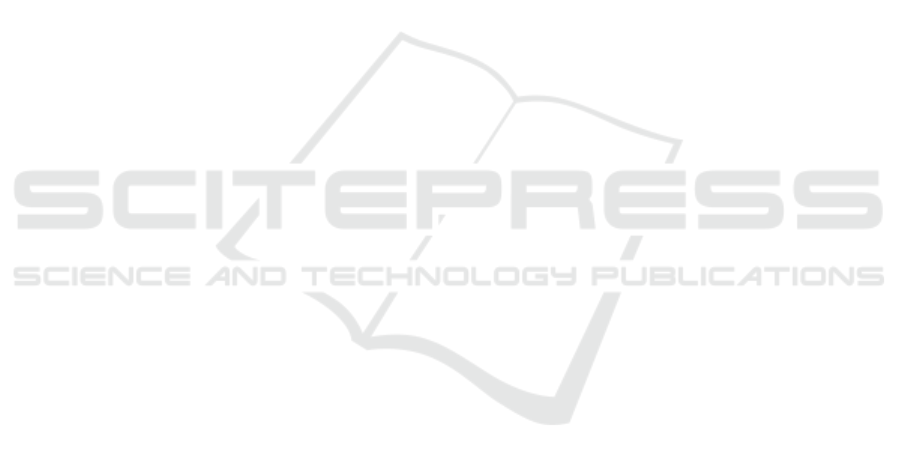
IEEE International Conference on Industrial Engi-
neering and Engineering Management (IEEM), pages
1602–1606. IEEE.
Group, E. (2016). Expert group 21: Obsolescence manage-
ment final report.
Gutlein, M., Frank, E., Hall, M., and Karwath, A. (2009).
Large-scale attribute selection using wrappers. In
2009 IEEE symposium on computational intelligence
and data mining, pages 332–339. IEEE.
Jennings, C., Wu, D., and Terpenny, J. (2016). Forecasting
obsolescence risk and product life cycle with machine
learning. IEEE Transactions on Components, Packag-
ing and Manufacturing Technology, 6(9):1428–1439.
Khalid, S., Khalil, T., and Nasreen, S. (2014). A survey of
feature selection and feature extraction techniques in
machine learning. In 2014 Science and Information
Conference, pages 372–378. IEEE.
Kohavi, R., John, G. H., et al. (1997). Wrappers for feature
subset selection. Artificial intelligence, 97(1-2):273–
324.
Kostrzewa, D. and Brzeski, R. (2017). The data di-
mensionality reduction in the classification process
through greedy backward feature elimination. In
International Conference on Man–Machine Interac-
tions, pages 397–407. Springer.
Lee, M.-C. (2009). Using support vector machine with a hy-
brid feature selection method to the stock trend predic-
tion. Expert Systems with Applications, 36(8):10896–
10904.
Li, B., Wang, Q., and Hu, J. (2011). Feature subset selec-
tion: a correlation-based svm filter approach. IEEJ
Transactions on Electrical and Electronic Engineer-
ing, 6(2):173–179.
Ma, J. and Kim, N. (2017). Electronic part obsolescence
forecasting based on time series modeling. Interna-
tional Journal of Precision Engineering and Manu-
facturing, 18(5):771–777.
Mellal, M. A. (2020). Obsolescence–a review of the litera-
ture. Technology in Society, page 101347.
Meng, X., Th
¨
ornberg, B., and Olsson, L. (2014). Strate-
gic proactive obsolescence management model. IEEE
Transactions on Components, Packaging and Manu-
facturing Technology, 4(6):1099–1108.
Muthukrishnan, R. and Rohini, R. (2016). Lasso: A fea-
ture selection technique in predictive modeling for
machine learning. In 2016 IEEE international confer-
ence on advances in computer applications (ICACA),
pages 18–20. IEEE.
Omri, N., Al Masry, Z., Mairot, N., Giampiccolo, S., and
Zerhouni, N. (2020). Industrial data management
strategy towards an sme-oriented phm. Journal of
Manufacturing Systems, 56:23–36.
Pingle, P. (2015). Selection of obsolescence resolution strat-
egy based on a multi criteria decision model.
Rish, I. et al. (2001). An empirical study of the naive bayes
classifier. In IJCAI 2001 workshop on empirical meth-
ods in artificial intelligence, volume 3, pages 41–46.
Rojo, F. J. R., Roy, R., and Shehab, E. (2010). Obsoles-
cence management for long-life contracts: state of the
art and future trends. The International Journal of Ad-
vanced Manufacturing Technology, 49(9-12):1235–
1250.
Samb, M. L., Camara, F., Ndiaye, S., Slimani, Y., and
Esseghir, M. A. (2012). Approche de s
´
election
d’attributs pour la classification bas
´
ee sur l’algorithme
rfe-svm. In 11
`
eme Colloque Africain sur la Recherche
en Informatique et Math
´
ematiques.
Sandborn, P. (2007). Software obsolescence-complicating
the part and technology obsolescence management
problem. IEEE Transactions on Components and
Packaging Technologies, 30(4):886–888.
Sandborn, P. (2013). Design for obsolescence risk manage-
ment. Procedia CIRP, 11:15–22.
Sandborn, P. (2017). Forecasting technology and part obso-
lescence. Proceedings of the Institution of Mechanical
Engineers, Part B: Journal of Engineering Manufac-
ture, 231(13):2251–2260.
Sandborn, P., Prabhakar, V., and Ahmad, O. (2011). Fore-
casting electronic part procurement lifetimes to enable
the management of dmsms obsolescence. Microelec-
tronics Reliability, 51(2):392–399.
Solomon, R., Sandborn, P. A., and Pecht, M. G. (2000).
Electronic part life cycle concepts and obsolescence
forecasting. IEEE Transactions on Components and
Packaging Technologies, 23(4):707–717.
Trabelsi, I., Zolghadri, M., Zeddini, B., Barkallah, M., and
Haddar, M. (2020). Fmeca-based risk assessment ap-
proach for proactive obsolescence management. In
IFIP International Conference on Product Lifecycle
Management, pages 215–226. Springer.
Wang, J., Liu, S., Gao, R. X., and Yan, R. (2012). Current
envelope analysis for defect identification and diag-
nosis in induction motors. Journal of Manufacturing
Systems, 31(4):380–387.
Zemouri, R., Omri, N., Devalland, C., Arnould, L., Morello,
B., Zerhouni, N., and Fnaiech, F. (2018). Breast can-
cer diagnosis based on joint variable selection and
constructive deep neural network. In 2018 IEEE 4th
Middle East Conference on Biomedical Engineering
(MECBME), pages 159–164. IEEE.
Zemouri, R., Omri, N., Fnaiech, F., Zerhouni, N., and
Fnaiech, N. (2019). A new growing pruning deep
learning neural network algorithm (gp-dlnn). Neural
Computing and Applications, pages 1–17.
Zhao, Z., Zhang, R., Cox, J., Duling, D., and Sarle, W.
(2013). Massively parallel feature selection: an ap-
proach based on variance preservation. Machine
learning, 92(1):195–220.
ICAART 2021 - 13th International Conference on Agents and Artificial Intelligence
794