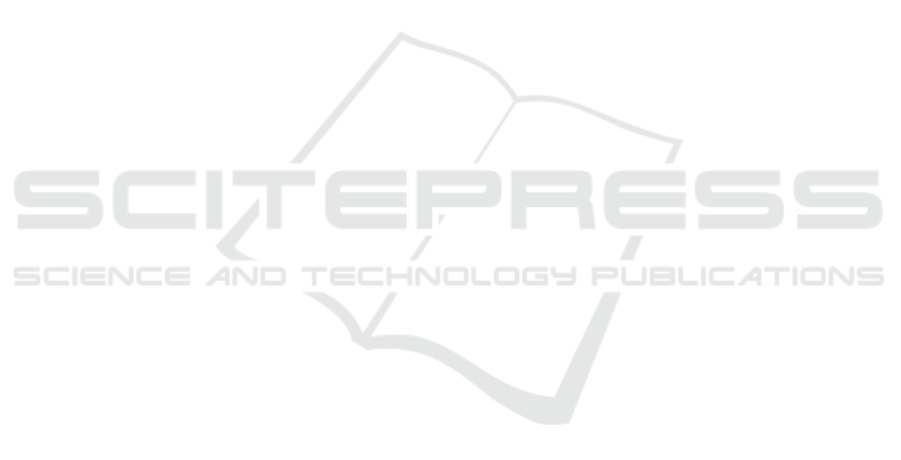
sification of manipulation building blocks using 1-
NN (Gutzeit et al., 2019b). While the LSTM network
performs better on data with higher inter-subject vari-
ations, this approach as well as HMM based classi-
fication cannot express their superior capabilities on
sequenced data in the classification of building blocks
of human arm movements.
For future work, a more detailed analysis of the
influence of the segmentation into building blocks to
reduce the complexity of the data, as well as the in-
sights of human movement generation that can be in-
ferred from this, would be of interest. These insights
could help, e.g., to improve the generation of robotic
behavior based on human examples to generate more
flexible robotic systems.
ACKNOWLEDGEMENTS
This work was supported through two grants of the
German Federal Ministry for Economic Affairs and
Energy (BMWi, FKZ 50 RA 1703 and 50 RA 2023).
REFERENCES
Aarno, D. and Kragic, D. (2008). Motion intention recog-
nition in robot assisted applications. Robotics and Au-
tonomous Systems, 56:692–705.
Argall, B. D., Chernova, S., Veloso, M., and Browning, B.
(2009). A survey of robot learning from demonstra-
tion. Robotics and Autonomous Systems, 57(5):469–
483.
Bishop, C. M. (2006). Pattern Recognition and Machine
Learning. Springer-Verlag New York, Inc.
Borghi, G., Vezzani, R., and Cucchiara, R. (2016). Fast
gesture recognition with Multiple Stream Discrete
HMMs on 3D skeletons. Proceedings - International
Conference on Pattern Recognition, pages 997–1002.
Gutzeit, L., Fabisch, A., Otto, M., Metzen, J. H., Hansen,
J., Kirchner, F., and Kirchner, E. A. (2018). The Be-
sMan Learning Platform for Automated Robot Skill
Learning. Frontiers in Robotics and AI, 5.
Gutzeit, L., Fabisch, A., Petzoldt, C., Wiese, H., and Kirch-
ner, F. (2019a). Automated Robot Skill Learning
from Demonstration for Various Robot Systems. In
Benzm
¨
uller, C. and Stuckenschmidt, H., editors, KI
2019: Advances in Artificial Intelligence, Conference
Proc., volume LNAI 11793, pages 168–181. Springer.
Gutzeit, L., Otto, M., and Kirchner, E. A. (2019b). Simple
and robust automatic detection and recognition of hu-
man movement patterns in tasks of different complex-
ity. In Physiological Computing Systems. Springer.
Hochreiter, S. and Schmidhuber, J. (1997). Long short-term
memory. Neural Computation, 9(8):1735–1780.
Liu, J., Shahroudy, A., Xu, D., Kot Chichung, A., and
Wang, G. (2017). Skeleton-Based Action Recognition
Using Spatio-Temporal LSTM Network with Trust
Gates. IEEE Transactions on Pattern Analysis and
Machine Intelligence, 40(12):3007–3021.
Patsadu, O., Nukoolkit, C., and Watanapa, B. (2012). Hu-
man gesture recognition using Kinect camera. Com-
puter Science and Software Engineering (JCSSE),
2012 International Joint Conference on, pages 28–32.
Poppe, R. (2010). A survey on vision-based human action
recognition. Image and Vision Computing, 28(6):976–
990.
Senger, L., Schr
¨
oer, M., Metzen, J. H., and Kirchner,
E. A. (2014). Velocity-based Multiple Change-
point Inference for Unsupervised Segmentation of
Human Movement Behavior. In Proccedings of the
22th International Conference on Pattern Recognition
(ICPR2014), pages 4564–4569.
Shi, Y., Tian, Y., Wang, Y., and Huang, T. (2017). Sequen-
tial Deep Trajectory Descriptor for Action Recogni-
tion with Three-Stream CNN. IEEE Transactions on
Multimedia, 19(7):1510–1520.
Stefanov, N., Peer, A., and Buss, M. (2010). Online inten-
tion recognition for computer-assisted teleoperation.
In Proceedings - IEEE International Conference on
Robotics and Automation, pages 5334–5339.
van der Maaten, L. and Hinton, G. (2008). Visualizing Data
using t-SNE. Journal of Machine Learning Research,
9:2579–2605.
Wang, Y., Yao, Q., Kwok, J. T., and Ni, L. M. (2020). Gen-
eralizing from a Few Examples: A Survey on Few-
shot Learning. ACM Computing Surveys, 53(3).
ICPRAM 2021 - 10th International Conference on Pattern Recognition Applications and Methods
250