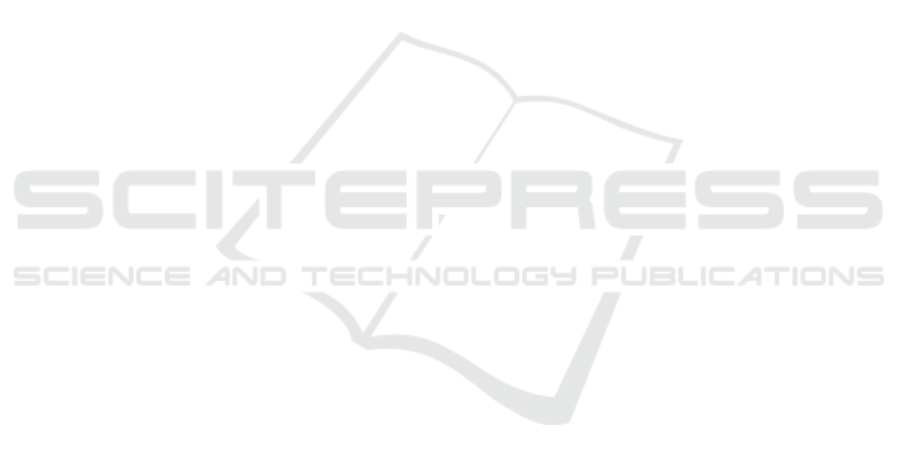
ration of different scales for rating. Furthermore, the
research introduces the need for interdisciplinary col-
laboration (e.g. by actively involving artists), serving
as a bridge between feature design and art.
REFERENCES
Amirshahi, S. A., Hayn-Leichsenring, G. U., Denzler, J.,
and Redies, C. (2014). Evaluating the rule of thirds
in photographs and paintings. Art & Perception, 2(1-
2):163–182.
Bay, H., Tuytelaars, T., and Van Gool, L. (2006). SURF:
Speeded up robust features. In European conference
on computer vision, pages 404–417. Springer.
Bharati, M. H., Liu, J. J., and MacGregor, J. F. (2004).
Image texture analysis: methods and comparisons.
Chemometrics and intelligent laboratory systems,
72(1):57–71.
Bishop, C. M. (2006). Pattern recognition and machine
learning. springer.
Bolthausen, E. and W
¨
uthrich, M. V. (2013). Bernoulli’s law
of large numbers. ASTIN Bulletin, 43(2):73–79.
Breiman, L. (2001). Random forests. Machine learning,
45(1):5–32.
Chen, T. and Guestrin, C. (2016). Xgboost: A scalable
tree boosting system. In Proceedings of the 22nd acm
sigkdd international conference on knowledge discov-
ery and data mining, pages 785–794. ACM.
Cortes, C. and Vapnik, V. (1995). Support-vector networks.
Machine learning, 20(3):273–297.
Davis, R. C. (1936). An evaluation and test of birkhoff’s
aesthetic measure formula. The Journal of General
Psychology, 15(2):231–240.
Deepa, S. and Devi, B. A. (2011). A survey on artifi-
cial intelligence approaches for medical image clas-
sification. Indian Journal of Science and Technology,
4(11):1583–1595.
Di Dio, C., Macaluso, E., and Rizzolatti, G. (2007). The
golden beauty: brain response to classical and renais-
sance sculptures. PloS one, 2(11):e1201.
Dietterich, T. G. (2000). Ensemble methods in machine
learning. In International workshop on multiple clas-
sifier systems, pages 1–15. Springer.
Friedman, J. H. (2001). Greedy function approximation: a
gradient boosting machine. Annals of statistics, pages
1189–1232.
Golub, G. H. and Reinsch, C. (1971). Singular value de-
composition and least squares solutions. In Linear Al-
gebra, pages 134–151. Springer.
Hinton, G. E. (1990). Connectionist learning procedures. In
Machine learning, pages 555–610. Elsevier.
Hoerl, A. E. and Kennard, R. W. (1970). Ridge regression:
Biased estimation for nonorthogonal problems. Tech-
nometrics, 12(1):55–67.
Jolliffe, I. (2011). Principal component analysis. Springer.
Jordanous, A. (2012). A standardised procedure for evalu-
ating creative systems: Computational creativity eval-
uation based on what it is to be creative. Cognitive
Computation, 4(3):246–279.
LeCun, Y., Boser, B. E., Denker, J. S., Henderson, D.,
Howard, R. E., Hubbard, W. E., and Jackel, L. D.
(1990). Handwritten digit recognition with a back-
propagation network. In Advances in neural informa-
tion processing systems, pages 396–404.
Leder, H., Belke, B., Oeberst, A., and Augustin, D. (2004).
A model of aesthetic appreciation and aesthetic judg-
ments. British journal of psychology, 95(4):489–508.
Lowe, D. G. (1999). Object recognition from local scale-
invariant features. In Computer vision, 1999. The pro-
ceedings of the seventh IEEE international conference
on, volume 2, pages 1150–1157. Ieee.
Masci, J., Meier, U., Cires¸an, D., and Schmidhuber, J.
(2011). Stacked convolutional auto-encoders for hi-
erarchical feature extraction. In International Con-
ference on Artificial Neural Networks, pages 52–59.
Springer.
Newton, I. (1987). Philosophiæ naturalis principia math-
ematica (mathematical principles of natural philoso-
phy). London (1687), 1687.
Nixon, M. and Aguado, A. S. (2012). Feature extraction
and image processing for computer vision. Academic
Press.
Pappas, N. (2008). Plato’s aesthetics.
ping Tian, D. et al. (2013). A review on image feature ex-
traction and representation techniques. International
Journal of Multimedia and Ubiquitous Engineering,
8(4):385–396.
Salleh, N. D. H. M. and Phon-Amnuaisuk, S. (2015). Quan-
tifying aesthetic beauty through its dimensions: a case
study on trochoids. International Journal of Knowl-
edge Engineering and Soft Data Paradigms, 5(1):51–
64.
Wolpert, D. H. (1992). Stacked generalization. Neural net-
works, 5(2):241–259.
Wu, S. G., Bao, F. S., Xu, E. Y., Wang, Y.-X., Chang, Y.-F.,
and Xiang, Q.-L. (2007). A leaf recognition algorithm
for plant classification using probabilistic neural net-
work. In Signal Processing and Information Technol-
ogy, 2007 IEEE International Symposium on, pages
11–16. IEEE.
Zhao, W., Krishnaswamy, A., Chellappa, R., Swets, D. L.,
and Weng, J. (1998). Discriminant analysis of princi-
pal components for face recognition. In Face Recog-
nition, pages 73–85. Springer.
Can We Detect Harmony in Artistic Compositions? A Machine Learning Approach
195