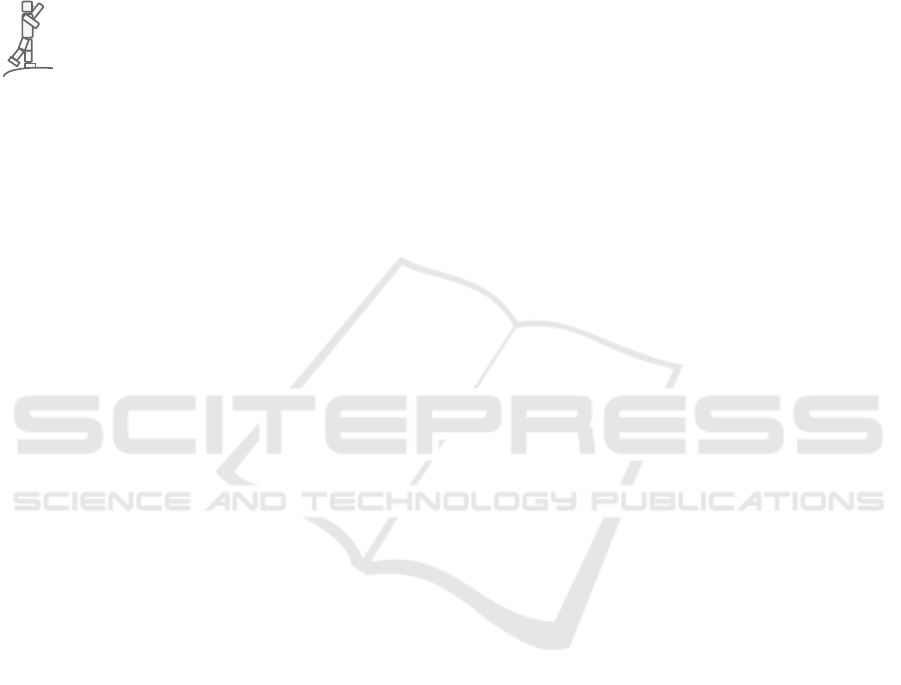
et al., 2019a; Lippi et al., 2020) that aims to make
a posture control testbed available for the humanoid
robotics community, and to define performance met-
rics.
ACKNOWLEDGEMENTS
This work is supported by the project
COMTEST, a sub-project of EUROBENCH
(European Robotic Framework for
Bipedal Locomotion Benchmarking,
www.eurobench2020.eu) funded by H2020
Topic ICT 27-2017 under grant agreement number
779963.
REFERENCES
Abdu-Aguye, M. G. and Gomaa., W. (2019a). On the fea-
sibility of on-body roaming models in human activity
recognition. In Proceedings of the 16th International
Conference on Informatics in Control, Automation and
Robotics - Volume 1: ICINCO,, pages 507–516. IN-
STICC, SciTePress.
Abdu-Aguye, M. G. and Gomaa., W. (2019b). Versatl: Ver-
satile transfer learning for imu-based activity recogni-
tion using convolutional neural networks. In Proceed-
ings of the 16th International Conference on Informat-
ics in Control, Automation and Robotics - Volume 1:
ICINCO,, pages 507–516. INSTICC, SciTePress.
Abedi, P. and Shoushtari, A. L. (2012). Modelling and
simulation of human-like movements for humanoid
robots. In Proceedings of the 9th International Con-
ference on Informatics in Control, Automation and
Robotics - Volume 1: ICINCO,, pages 342–346. IN-
STICC, SciTePress.
Andersson, C., Ribeiro, A. H., Tiels, K., Wahlstr
¨
om, N., and
Sch
¨
on, T. B. (2019). Deep convolutional networks in
system identification. In 2019 IEEE 58th Conference
on Decision and Control (CDC), pages 3670–3676.
IEEE.
Antritter, F., Scholz, F., Hettich, G., and Mergner, T. (2014).
Stability analysis of human stance control from the
system theoretic point of view. In Control Conference
(ECC), 2014 European, pages 1849–1855. IEEE.
Assl
¨
ander, L., Hettich, G., and Mergner, T. (2015). Visual
contribution to human standing balance during support
surface tilts. Human movement science, 41:147–164.
Batchuluun, G., Naqvi, R. A., Kim, W., and Park, K. R.
(2018). Body-movement-based human identification
using convolutional neural network. Expert Systems
with Applications, 101:56–77.
Boonstra, T. A., van Vugt, J. P., van der Kooij, H., and Bloem,
B. R. (2014). Balance asymmetry in parkinson’s dis-
ease and its contribution to freezing of gait. PLoS One,
9(7):e102493.
Choi, Y. and Kim, D. (2007). On the balancing control of
humanoid robot. In Proceedings of the Fourth Inter-
national Conference on Informatics in Control, Au-
tomation and Robotics - Volume 2: ICINCO,, pages
248–252. INSTICC, SciTePress.
Chugo, D., Koyama, M., Yokota, M., Kawazoe, S., Mu-
ramatsu, S., Yokota, S., Hashimoto, H., Katayama,
T., Mizuta, Y., and Koujina, A. (2019). Sitting assis-
tance that considers user posture tolerance. In Proceed-
ings of the 16th International Conference on Informat-
ics in Control, Automation and Robotics - Volume 2:
ICINCO,, pages 489–496. INSTICC, SciTePress.
De la Rosa, E. and Yu, W. (2016). Randomized algorithms
for nonlinear system identification with deep learning
modification. Information Sciences, 364:197–212.
De la Rosa, E., Yu, W., and Li, X. (2015). Nonlinear system
identification using deep learning and randomized al-
gorithms. In 2015 IEEE International Conference on
Information and Automation, pages 274–279. IEEE.
Engelhart, D., Boonstra, T. A., Aarts, R. G., Schouten, A. C.,
and van der Kooij, H. (2016). Comparison of closed-
loop system identification techniques to quantify multi-
joint human balance control. Annual reviews in control,
41:58–70.
Engelhart, D., Pasma, J. H., Schouten, A. C., Meskers, C. G.,
Maier, A. B., Mergner, T., and van der Kooij, H. (2014).
Impaired standing balance in elderly: a new engineer-
ing method helps to unravel causes and effects. Jour-
nal of the American Medical Directors Association,
15(3):227–e1.
Goodworth, A. D. and Peterka, R. J. (2018). Identifying
mechanisms of stance control: a single stimulus multi-
ple output model-fit approach. Journal of neuroscience
methods, 296:44–56.
Hettich, G., Assl
¨
ander, L., Gollhofer, A., and Mergner, T.
(2014). Human hip—ankle coordination emerging
from multisensory feedback control. Human Movement
Science, 37:123–146.
Hettich, G., Lippi, V., and Mergner, T. (2013). Human-like
sensor fusion mechanisms in a postural control robot.
In Londral, A. E., Encarnacao, P., and Pons, J. L., ed-
itors, Proceedings of the International Congress on
Neurotechnology, Electronics and Informatics. Vilam-
oura, Portugal, pages 152–160.
Hettich, G., Lippi, V., and Mergner, T. (2015). Human-like
sensor fusion implemented in the posture control of a
bipedal robot. In Neurotechnology, Electronics, and
Informatics, pages 29–45. Springer.
Jeka, J. J., Allison, L. K., and Kiemel, T. (2010). The dynam-
ics of visual reweighting in healthy and fall-prone older
adults. Journal of motor behavior, 42(4):197–208.
Karatzoglou, A., Schnell, N., and Beigl, M. (2018). A convo-
lutional neural network approach for modeling seman-
tic trajectories and predicting future locations. In In-
ternational Conference on Artificial Neural Networks,
pages 61–72. Springer.
Lippi, V. (2018). Prediction in the context of a human-
inspired posture control model. Robotics and Au-
tonomous Systems.
ICPRAM 2021 - 10th International Conference on Pattern Recognition Applications and Methods
546