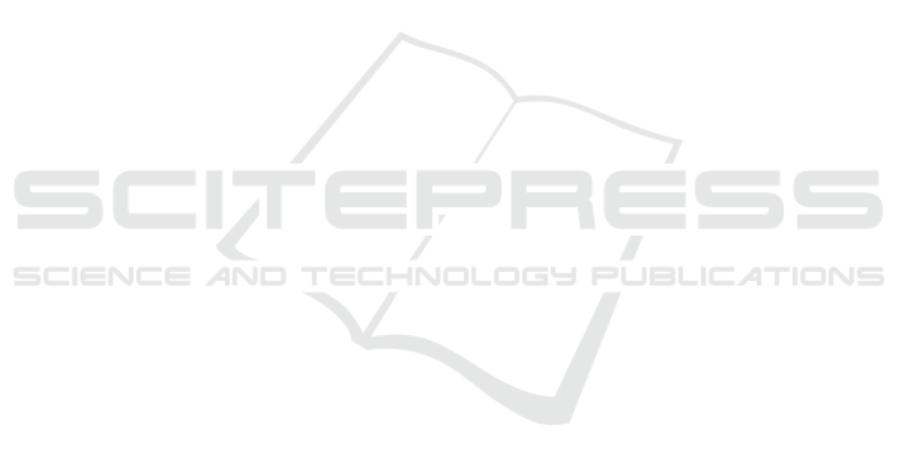
Azzopardi., G. (2020). Device-based image matching
with similarity learning by convolutional neural net-
works that exploit the underlying camera sensor pat-
tern noise. In Proceedings of the 9th International
Conference on Pattern Recognition Applications and
Methods - Volume 1: ICPRAM,, pages 578–584. IN-
STICC, SciTePress.
Bondi, L., Baroffio, L., G
¨
uera, D., Bestagini, P., Delp,
E. J., and Tubaro, S. (2016). First steps toward cam-
era model identification with convolutional neural net-
works. IEEE Signal Processing Letters, 24(3):259–
263.
Caldell, R., Amerini, I., Picchioni, F., De Rosa, A., and
Uccheddu, F. (2010). Multimedia forensic techniques
for acquisition device identification and digital image
authentication. In Handbook of Research on Compu-
tational Forensics, Digital Crime, and Investigation:
Methods and Solutions, pages 130–154. IGI Global.
Chen, C. and Stamm, M. C. (2015). Camera model identi-
fication framework using an ensemble of demosaicing
features. In 2015 IEEE International Workshop on In-
formation Forensics and Security (WIFS), pages 1–6.
IEEE.
Chen, J., Kang, X., Liu, Y., and Wang, Z. J. (2015). Median
filtering forensics based on convolutional neural net-
works. IEEE Signal Processing Letters, 22(11):1849–
1853.
Dirik, A. E., Sencar, H. T., and Memon, N. (2008). Digital
single lens reflex camera identification from traces of
sensor dust. IEEE Transactions on Information Foren-
sics and Security, 3(3):539–552.
Geradts, Z. J., Bijhold, J., Kieft, M., Kurosawa, K., Kuroki,
K., and Saitoh, N. (2001). Methods for identification
of images acquired with digital cameras. In Enabling
technologies for law enforcement and security, vol-
ume 4232, pages 505–512. International Society for
Optics and Photonics.
Hosler, B., Mayer, O., Bayar, B., Zhao, X., Chen, C., Shack-
leford, J. A., and Stamm, M. C. (2019). A video cam-
era model identification system using deep learning
and fusion. In ICASSP 2019-2019 IEEE International
Conference on Acoustics, Speech and Signal Process-
ing (ICASSP), pages 8271–8275. IEEE.
Li, C.-T. (2010). Source camera identification using en-
hanced sensor pattern noise. IEEE Transactions on
Information Forensics and Security, 5(2):280–287.
Luk
´
a
ˇ
s, J., Fridrich, J., and Goljan, M. (2006). Detecting
digital image forgeries using sensor pattern noise. In
Security, Steganography, and Watermarking of Multi-
media Contents VIII, volume 6072, page 60720Y. In-
ternational Society for Optics and Photonics.
Lukas, J., Fridrich, J., and Goljan, M. (2006). Digital cam-
era identification from sensor pattern noise. IEEE
Transactions on Information Forensics and Security,
1(2):205–214.
Milani, S., Bestagini, P., Tagliasacchi, M., and Tubaro,
S. (2014). Demosaicing strategy identification via
eigenalgorithms. In 2014 IEEE International Con-
ference on Acoustics, Speech and Signal Processing
(ICASSP), pages 2659–2663. IEEE.
Milani, S., Fontani, M., Bestagini, P., Barni, M., Piva, A.,
Tagliasacchi, M., and Tubaro, S. (2012). An overview
on video forensics. APSIPA Transactions on Signal
and Information Processing, 1.
Pibre, L., Pasquet, J., Ienco, D., and Chaumont, M. (2016).
Deep learning is a good steganalysis tool when em-
bedding key is reused for different images, even if
there is a cover sourcemismatch. Electronic Imaging,
2016(8):1–11.
Qiu, X., Li, H., Luo, W., and Huang, J. (2014). A universal
image forensic strategy based on steganalytic model.
In Proceedings of the 2nd ACM workshop on Informa-
tion hiding and multimedia security, pages 165–170.
Shullani, D., Fontani, M., Iuliani, M., Al Shaya, O., and
Piva, A. (2017). Vision: a video and image dataset for
source identification. EURASIP Journal on Informa-
tion Security, 2017(1):15.
Video Camera Identification from Sensor Pattern Noise with a Constrained ConvNet
425