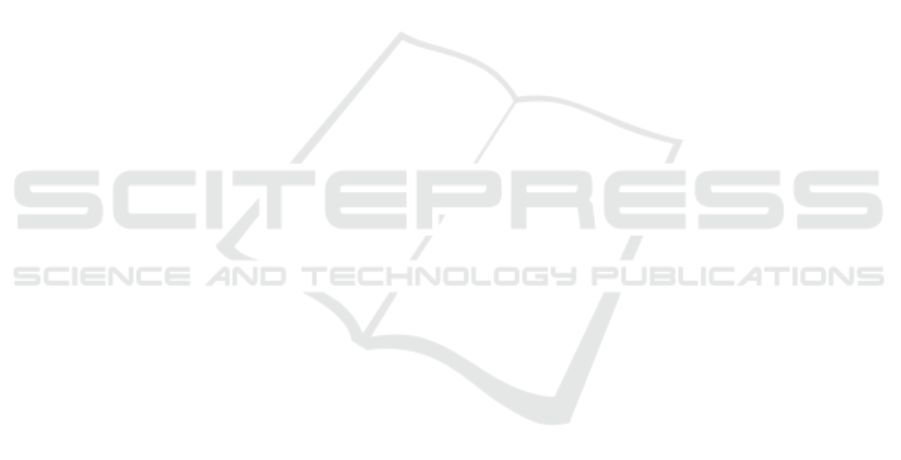
Amruthnath, N. and Gupta, T. (2018). A research study on
unsupervised machine learning algorithms for early
fault detection in predictive maintenance. In 2018
5th International Conference on Industrial Engineer-
ing and Applications, ICIEA 2018, pages 355–361. In-
stitute of Electrical and Electronics Engineers Inc.
Aremu, O. O., Hyland-Wood, D., and McAree, P. R. (2020).
A machine learning approach to circumventing the
curse of dimensionality in discontinuous time series
machine data. Reliability Engineering and System
Safety, 195(October 2019):106706.
Bangalore, P. and Patriksson, M. (2018). Analysis of
SCADA data for early fault detection, with applica-
tion to the maintenance management of wind turbines.
Renewable Energy.
Cai, J., Luo, J., Wang, S., and Yang, S. (2018). Feature se-
lection in machine learning: A new perspective. Neu-
rocomputing.
Canizo, M., Onieva, E., Conde, A., Charramendieta, S.,
and Trujillo, S. (2017). Real-time predictive main-
tenance for wind turbines using Big Data frameworks.
2017 IEEE International Conference on Prognostics
and Health Management, ICPHM 2017, pages 70–77.
Carvalho, T. P., Soares, F. A. A. M. N., Vita, R., and Fran-
cisco, P. (2019). A systematic literature review of ma-
chine learning methods applied to predictive mainte-
nance. Computers & Industrial Engineering, 137(Au-
gust):106024.
Einabadi, B., Baboli, A., and Ebrahimi, M. (2019). Dy-
namic Predictive Maintenance in industry 4.0 based
on real time information: Case study in automotive
industries. IFAC-PapersOnLine, 52(13):1069–1074.
Hsu, J. Y., Wang, Y. F., Lin, K. C., Chen, M. Y., and Hsu, J.
H. Y. (2020). Wind Turbine Fault Diagnosis and Pre-
dictive Maintenance Through Statistical Process Con-
trol and Machine Learning. IEEE Access, 8:23427–
23439.
Hu, J., Zhang, L., and Liang, W. (2012). Opportunistic
predictive maintenance for complex multi-component
systems based on DBN-HAZOP model. Process
Safety and Environmental Protection.
Kanawaday, A. and Sane, A. (2018). Machine learning for
predictive maintenance of industrial machines using
IoT sensor data. Proceedings of the IEEE Interna-
tional Conference on Software Engineering and Ser-
vice Sciences, ICSESS, 2017-Novem(Figure 2):87–90.
Kusiak, A. and Verma, A. (2012). A Data-Mining Approach
to Monitoring. IEEE Transactions on Sustainable En-
ergy, 3(1):150–157.
Lee, H. H. Y. and Scott, D. (2009). Overview of mainte-
nance strategy, acceptable maintenance standard and
resources from a building maintenance operation per-
spective. Journal of Building Appraisal.
Nichenametla, A. N., Nandipati, S., and Waghmare, A. L.
(2017). Optimizing life cycle cost of wind turbine
blades using predictive analytics in effective mainte-
nance planning. In Proceedings - Annual Reliability
and Maintainability Symposium.
Paolanti, M., Romeo, L., Felicetti, A., Mancini, A., Fron-
toni, E., and Loncarski, J. (2018). Machine Learn-
ing approach for Predictive Maintenance in Industry
4.0. 2018 14th IEEE/ASME International Conference
on Mechatronic and Embedded Systems and Applica-
tions, MESA 2018, pages 1–6.
Sakib, N. and Wuest, T. (2018). Challenges and Opportu-
nities of Condition-based Predictive Maintenance: A
Review. In Procedia CIRP.
Tian, Z., Lin, D., and Wu, B. (2012). Condition based main-
tenance optimization considering multiple objectives.
Journal of Intelligent Manufacturing.
Uz, F. B. (2016). Predictive Maintenance Modelling Guide
Data Sets.
Vu, H. C., Do, P., Fouladirad, M., and Grall, A. (2020). Dy-
namic opportunistic maintenance planning for multi-
component redundant systems with various types of
opportunities. Reliability Engineering and System
Safety.
Yurek, O. E. and Birant, D. (2019). Remaining Useful Life
Estimation for Predictive Maintenance Using Feature
Engineering. Proceedings - 2019 Innovations in In-
telligent Systems and Applications Conference, ASYU
2019, pages 1–5.
Machine Learning and Optimization for Predictive Maintenance based on Predicting Failure in the Next Five Days
199