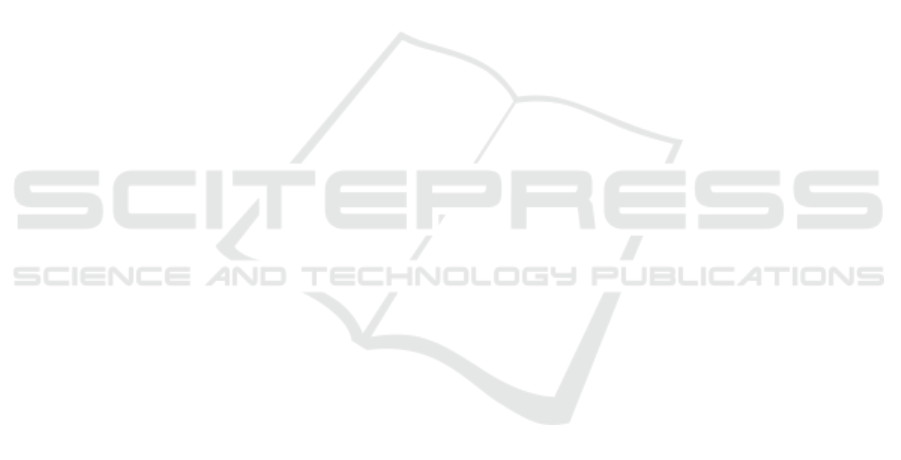
number of sectors (or zones) we use the more faster is
the ZBA approach. On the other hand, CBA remains
insensitive to the change of number of zones, however
PBA becomes slower. From Fig. 8, we could say that
as the number of car parks increases, the possibility of
finding a parking space increases more. From Fig. 9
and Fig. 10, we can deduce that even though CBA
approach is the fastest of the three approaches, the
agents of the CBA start to run out of steam compared
to the PBA, certainly because the number of agents is
more important. From these approaches we can infer
that the most suitable approach for the Cloud environ-
ment is the PBA. It is a model which is not the slow-
est amid the three approaches and which exchanges a
volume of data which is not the highest.
5 CONCLUSION
As mentioned, our distributed model uses the
VANETs that connect agents both for relaying the
parking status and for inter-agent negotiations. The
reservations are then relayed to a central server. By
doing so, we decrease the volume of data exchanged
in the Cloud. Contrarily, in the centralized case,
there is a central server which gathers parking re-
quests, real-time information (i.e., vehicle location,
traffic condition, users preferences), etc. At every pe-
riod of time T (related to the lifetime of the informa-
tion on the availability of spots) the central server re-
ceives thousands of variables about the status of all
car parks of the system, which could be excessively
time-consuming and potentially incur high cost and
slow-downs if there are huge amounts of data ex-
changed via the Cloud like in large car parks area. As
a perspective, our next task will be to investigate the
impact of our process on communication costs, and
show that our model transfers less data and is there-
fore less expensive than the centralized one.
REFERENCES
Ayala, D., Wolfson, O., Xu, B., Dasgupta, B., and Lin, J.
(2011). Parking slot assignment games. In Proceed-
ings of the 19th ACM SIGSPATIAL International Con-
ference on Advances in Geographic Information Sys-
tems, pages 299–308.
Bessghaier, N., Zargayouna, M., and Balbo, F. (2012). On-
line localized resource allocation application to urban
parking management. In 2012 IEEE/WIC/ACM Inter-
national Conferences on Web Intelligence and Intelli-
gent Agent Technology, volume 2, pages 67–74. IEEE.
Boudali, I. and Ouada, M. B. (2017). Smart parking reser-
vation system based on distributed multicriteria ap-
proach. Applied Artificial Intelligence, 31(5-6):518–
537.
Chou, S.-Y., Arifin, Z., and Dewabharata, A. (2018). Opti-
mal decision of reservation policy for private parking
sharing system. In 2018 International Conference on
Computer, Control, Informatics and its Applications
(IC3INA), pages 66–71. IEEE.
Chou, S.-Y., Lin, S.-W., and Li, C.-C. (2008). Dynamic
parking negotiation and guidance using an agent-
based platform. Expert Systems with applications,
35(3):805–817.
Farinelli, A., Rogers, A., Petcu, A., and Jennings, N. R.
(2008). Decentralised coordination of low-power em-
bedded devices using the max-sum algorithm. In 7th
International Joint Conference on Autonomous Agents
and Multiagent Systems (AAMAS 2008), Estoril, Por-
tugal, Volume 2, pages 639–646.
Fioretto, F., Pontelli, E., and Yeoh, W. (2018). Distributed
constraint optimization problems and applications: A
survey. Journal of Artificial Intelligence Research,
61:623–698.
Hedderich, M., Fastenrath, U., Isaac, G., and Bogenberger,
K. (2017). Adapting the a* algorithm for park spot
routing. Transportation Research Procedia, 27:1066–
1073.
Kazi, S., Nuzhat, S., Nashrah, A., and Rameeza, Q. (2018).
Smart parking system to reduce traffic congestion.
In 2018 International Conference on Smart City and
Emerging Technology (ICSCET), pages 1–4. IEEE.
L
´
eaut
´
e, T., Ottens, B., and Faltings, B. (2010). Ensuring
privacy through distributed computation in multiple-
depot vehicle routing problems. In Proceedings of the
ECAI’10 Workshop on Artificial Intelligence and Lo-
gistics (AILog’10).
L
´
eaut
´
e, T., Ottens, B., and Szymanek, R. (2009). Frodo 2.0:
An open-source framework for distributed constraint
optimization. In Proceedings of the IJCAI’09 Dis-
tributed Constraint Reasoning Workshop (DCR’09),
pages 160–164.
Picard, G., Balbo, F., and Boissier, O. (2018). Approches
multiagents pour l’allocation de courses
`
a une flotte de
taxis autonomes. Rev. d’Intelligence Artif., 32(2):223–
247.
Weiss, M., Lubin, B., and Seuken, S. (2017). Sats: A uni-
versal spectrum auction test suite. In Proceedings of
the 16th Conference on Autonomous Agents and Mul-
tiAgent Systems, pages 51–59.
Zeenat, R., Ashifuddin, M., and Marijn, J. (2018). A
multiple-criteria algorithm for smart parking: mak-
ing fair and preferred parking reservations in smart
cities. In Proceedings of the 19th Annual International
Conference on Digital Government Research: Gover-
nance in the Data Age, pages 1–9.
ICAART 2021 - 13th International Conference on Agents and Artificial Intelligence
204