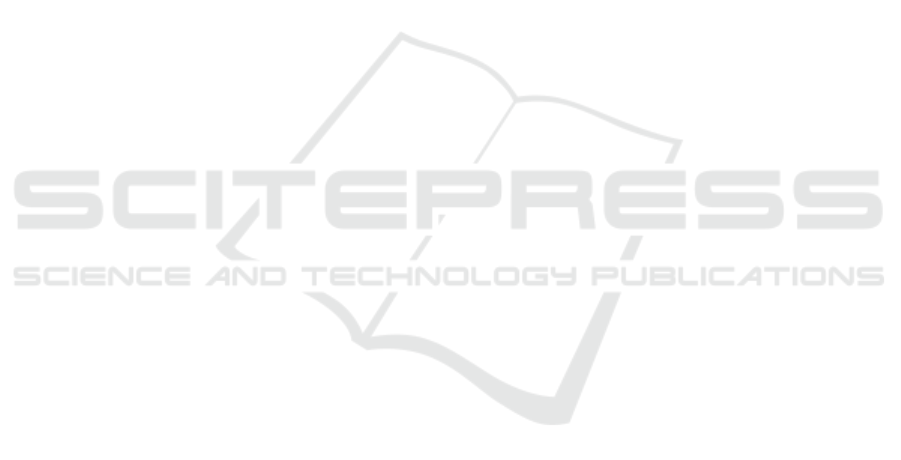
provided by a professional cook. These component
recipes were freely combined to valid recipes, with
one component from each set, considering the avail-
able devices (changing combinations of hob, steamer,
oven). The average number of tasks in a menu was
33.6 (C: 14.3, V: 4.6, M: 14.6). After the enrich-
ment of the recipes into a complete description of the
cooking process, the average task number changed to
44.3 (in case of multiple options the average num-
ber of tasks was counted). Multiple freely combined
recipes were prepared as system validation, both with
and without food, resulting in a functional cooking as-
sistance, in terms of planning, re-planning in case of
deviations, device control and automatic confirmation
of user interactions from the devices.
4 CONCLUSION
While regular (human readable) recipes look well-
structured and simple, the cooking process itself fre-
quently includes many pitfalls and unwritten (but nec-
essary) small pre- and post-actions which determine
the success of cooking. Therefore, today’s available
cooking assistants are designed either for a very spe-
cific device or for predefined hand-modeled recipes
which are completely decoupled from kitchen de-
vices. In comparison to other cooking assistants our
action templates enriches the recipe steps not only
with necessary information for visualization, plan-
ning, micro actions, and commands for device coor-
dination, but also with information about user-device
interaction and optional preparation alternatives. In
this paper, we propose action templates as a back-
bone for modeling component recipes that can be en-
riched and combined to full-blown descriptions of the
human-device interactions during a cooking process
and show its potential and benefits.
REFERENCES
An, Y., Cao, Y., Chen, J., Ngo, C.-W., Jia, J., Luan, H., and
Chua, T.-S. (2017). Pic2dish: A customized cook-
ing assistant system. In Proceedings of the 25th ACM
International Conference on Multimedia, MM ’17,
page 1269–1273, New York, NY, USA. Association
for Computing Machinery.
Badra, F., Bendaoud, R., Bentebibel, R., Champin, P.-A.,
Cojan, J., Cordier, A., Despres, S., Jean-Daubias, S.,
Lieber, J., Meilender, T., Mille, A., Nauer, E., Napoli,
A., and Toussaint, Y. (2008). Taaable: Text mining,
ontology engineering, and hierarchical classification
for textual case-based cooking.
Beetz, M., Stulp, F., Radig, B., Bandouch, J., Blodow, N.,
Dolha, M., Fedrizzi, A., Jain, D., Klank, U., Kresse, I.,
Maldonado, A., Marton, Z., Mosenlechner, L., Ruiz,
F., Rusu, R., and Tenorth, M. (2008). The assis-
tive kitchen — a demonstration scenario for cognitive
technical systems. pages 1 – 8.
Bergmann, R., Minor, M., M
¨
uller, G., and Schumacher, P.
(2017). Project ever: Extraction and processing of
procedural experience knowledge in workflows. In
ICCBR (Workshops), pages 137–146.
Damen, D., Doughty, H., Farinella, G. M., Fidler, S.,
Furnari, A., Kazakos, E., Moltisanti, D., Munro, J.,
Perrett, T., Price, W., and Wray, M. (2018). Scaling
egocentric vision: The epic-kitchens dataset. In Euro-
pean Conference on Computer Vision (ECCV).
De Smet, G. et al. (2016). Optaplanner user guide. Red
Hat and the community. URL http://www. optaplanner.
org. OptaPlanner is an open source constraint satis-
faction solver in Java.
Hamada, R., Okabe, J., Ide, I., Satoh, S., Sakai, S., and
Tanaka, H. (2005). Cooking navi: assistant for daily
cooking in kitchen. In Proceedings of the 13th annual
ACM international conference on Multimedia, pages
371–374.
Nauer, E. and Wilson, D. C. (2015). Computer cooking
contest.
Neumann, A., Elbrechter, C., Pfeiffer-Leßmann, N.,
K
˜
oiva, R., Carlmeyer, B., R
¨
uther, S., Schade, M.,
¨
Uckermann, A., Wachsmuth, S., and Ritter, H. J.
(2017). “kognichef”: A cognitive cooking assistant.
KI - K
¨
unstliche Intelligenz, 31(3):273–281.
Salvador, A., Hynes, N., Aytar, Y., Mar
´
ın, J., Ofli, F., Weber,
I., and Torralba, A. (2017). Learning cross-modal em-
beddings for cooking recipes and food images. pages
3068–3076.
Sato, A., Watanabe, K., and Rekimoto, J. (2014). Mimi-
cook: A cooking assistant system with situated guid-
ance. pages 121–124.
Sutton, D. (2018). Cooking Skills, the Senses, and Memory:
The Fate of Practical Knowledge*, pages 88–109.
Tenorth, M., Perzylo, A. C., Lafrenz, R., and Beetz, M.
(2012). The RoboEarth language: Representing and
Exchanging Knowledge about Actions, Objects, and
Environments. In IEEE International Conference on
Robotics and Automation (ICRA), St. Paul, MN, USA.
Best Cognitive Robotics Paper Award.
Yamakata, Y., Maeta, H., Kadowaki, T., Sasada, T., Ima-
hori, S., and Mori, S. (2017). Cooking recipe search
by pairs of ingredient and action —word sequence
v.s. flow-graph representation—. Transactions of The
Japanese Society for Artificial Intelligence, 32:71–79.
Recipe Enrichment: Knowledge Required for a Cooking Assistant
829