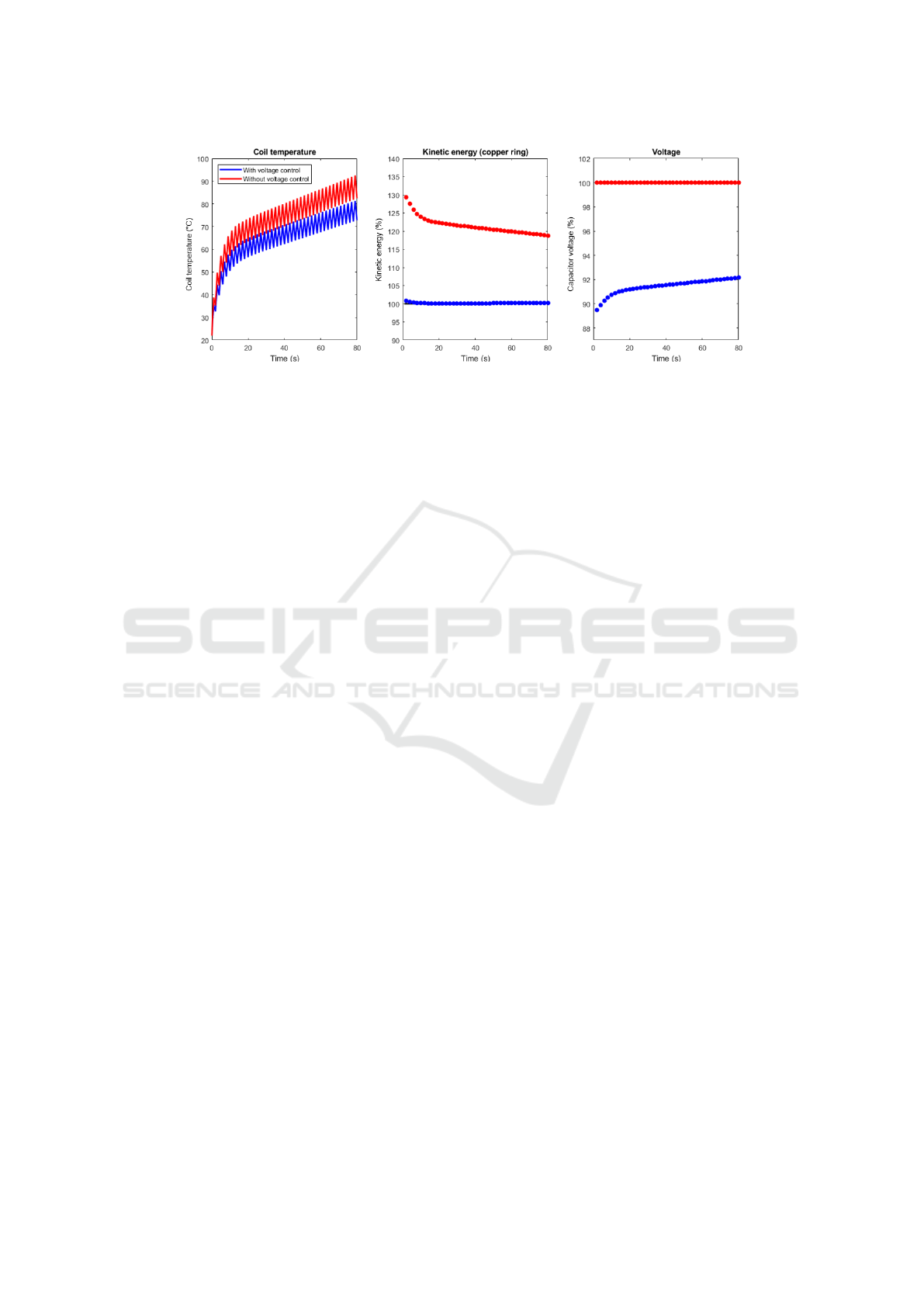
Figure 12: During 40 operation cycles, voltage control has a significant influence on the heat generation in the system. It leads
to a continuous character of the kinetic energy and requires less energy in the capacitor.
ation in the system leads to increasing coil tempera-
tures, although the system is already cooled. By using
a constant input voltage of 100% to make the system
reaching 100% kinetic energy of the conductive ring
even at the end of the lifetime, a lot of energy is un-
necessarily invested leading to longer charging times
in the capacitor. If voltage control is included, less
voltage is needed and the system can adapt to tem-
perature changes and decreasing capacitance due to
abrasion of the capacitor. The optimal performance
at 100% kinetic energy is achieved with errors of less
than 2% and, therefore, makes the whole system more
efficient and achieves reliably the desired optimal per-
formance point.
7 CONCLUSIONS
A virtual sensor is proposed for the temperature deter-
mination in the coil of an induction actuator to con-
trol its performance. Beside the introduced system
simulation model, the sensor can be used for predict-
ing the temperature of a coil when physical hardware
sensor measurements of the coil current and volt-
age are available. A validated and highly accurate
FEM model is used to generate training data for an
AI-based virtual sensor. The electromagnetic FEM
model takes hours to calculate the system response of
one single operation cycle. In contrast, a trained ML
model predicts the temperature of the coil within mil-
liseconds in places where no sensor can be integrated
without reducing the performance. When consider-
ing thousands of working cycles, excessive comput-
ing equipment would be needed to describe the tem-
perature accurately and efficiently with FEM models.
The virtual sensor therefore not only allows measure-
ments in places that are difficult to reach, but also en-
ables a fast and very accurate calculation method.
REFERENCES
Boulandet, R. (2016). Sensorless Measurement of the
Acoustic Impedance of a Loudspeaker. (1):7353–
7360.
Charkhgard, M. and Farrokhi, M. (2010). State-of-charge
estimation for lithium-ion batteries using neural net-
works and EKF. IEEE Transactions on Industrial
Electronics, 57(12):4178–4187.
Dong, E., Tian, P., Wang, Y., and Liu, W. (2011). The de-
sign and experimental analysis of high-speed switch
in 1.14kV level based on novel repulsion actuator.
2011 4th International Conference on Electric Utility
Deregulation and Restructuring and Power Technolo-
gies (DRPT), (50807006):767–770.
Galili, I., Kaplan, D., and Lehavi, Y. (2012). physics course.
337.
Gonzaga, J. C. B., Meleiro, L. A. C., Kiang, C., and Filho,
R. M. (2009). ANN-based soft-sensor for real-time
process monitoring and control of an industrial poly-
merization process. 33:43–49.
Guzm, C. H., Carrera, J. L., Dur, A., Berumen, J., Ortiz,
A. A., Guirette, O. A., Brizuela, J. A., and Fabio, G.
(2018). Implementation of Virtual Sensors for Mon-
itoring Temperature in Greenhouses Using CFD and
Control.
Hussein, A. A. (2018). A Sensorless Surface Tempera-
ture Measurement Method for Batteries using Artifi-
cial Neural Networks. pages 3560–3564.
Kumar, S., Tan, S., Zheng, L., and Kochmann, D. M.
(2020). Inverse-designed spinodoid metamaterials.
npj Computational Materials, 6(1):1–10.
Lim, D.-k., Woo, D.-k., Kim, I.-w., Shin, D.-k., Ro, J.-s.,
Chung, T.-k., and Jung, H.-k. (2013). Characteris-
tic Analysis and Design of a Thomson Coil Actuator
Using an Analytic Method and a Numerical Method.
49(12):5749–5755.
Vilchis-Rodriguez, D. S., Shuttleworth, R., Smith, A. C.,
and Barnes, M. (2019). Performance of high-power
thomson coil actuator excited by a current pulse train.
2019:3937–3941.
ICAART 2021 - 13th International Conference on Agents and Artificial Intelligence
836