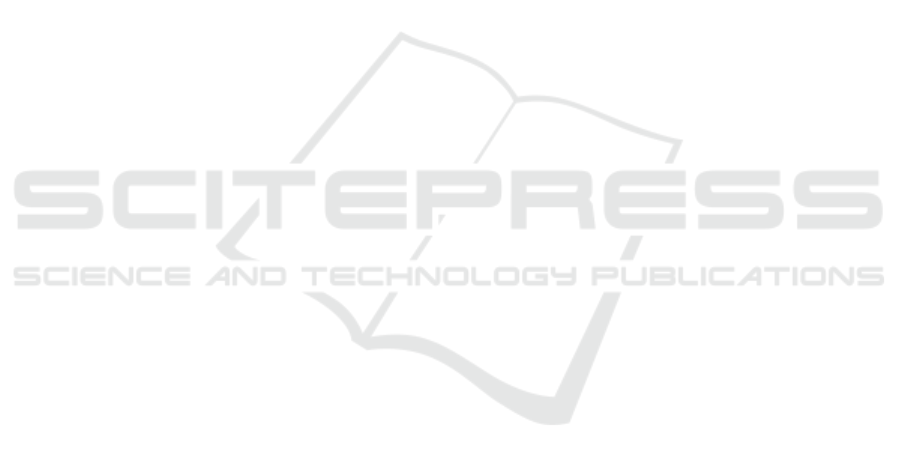
Cheng, R., Khojasteh, M. J., Ames, A. D., and Burdick,
J. W. (2020). Safe multi-agent interaction through ro-
bust control barrier functions with learned uncertain-
ties. arXiv preprint arXiv:2004.05273.
Ciesinski, F. and Gr
¨
oßer, M. (2004). On probabilistic com-
putation tree logic. In Validation of Stochastic Sys-
tems, pages 147–188.
Cizelj, I., Ding, X. C. D., Lahijanian, M., Pinto, A., and
Belta, C. (2011). Probabilistically safe vehicle control
in a hostile environment. IFAC Proceedings Volumes,
44(1):11803–11808.
Dehnert, C., Junges, S., Katoen, J.-P., and Volk, M. (2017).
A storm is coming: A modern probabilistic model
checker. In International Conference on Computer
Aided Verification, pages 592–600. Springer.
Fan, Y., Feng, G., Wang, Y., and Qiu, J. (2011). A novel
approach to coordination of multiple robots with com-
munication failures via proximity graph. Automatica,
47(8):1800–1805.
Garcia, J. and Fern
´
andez, F. (2012). Safe exploration
of state and action spaces in reinforcement learning.
Journal of Artificial Intelligence Research, 45:515–
564.
Garcia, J. and Fern
´
andez, F. (2015). A comprehensive sur-
vey on safe reinforcement learning. Journal of Ma-
chine Learning Research, 16(1):1437–1480.
Gerasimou, S., Calinescu, R., Shevtsov, S., and Weyns,
D. (2017). Undersea: an exemplar for engineering
self-adaptive unmanned underwater vehicles. In 2017
IEEE/ACM 12th International Symposium on Soft-
ware Engineering for Adaptive and Self-Managing
Systems (SEAMS), pages 83–89. IEEE.
Gerasimou, S., Calinescu, R., and Tamburrelli, G. (2018).
Synthesis of probabilistic models for quality-of-
service software engineering. Automated Software
Engineering, 25(4):785–831.
Gregory, J., Fink, J., Stump, E., Twigg, J., Rogers, J.,
Baran, D., Fung, N., and Young, S. (2016). Appli-
cation of multi-robot systems to disaster-relief scenar-
ios with limited communication. In Field and Service
Robotics, pages 639–653. Springer.
Kroening, D., Abate, A., and Hasanbeig, M. (2020). To-
wards verifiable and safe model-free reinforcement
learning. CEUR Workshop Proceedings.
Kwiatkowska, M. (2007). Quantitative verification: models
techniques and tools. In 6th Joint meeting of the Eu-
ropean software engineering conference and the ACM
SIGSOFT symposium on The foundations of software
engineering, pages 449–458.
Liu, Z., Chen, B., Zhou, H., Koushik, G., Hebert, M.,
and Zhao, D. (2020). Mapper: Multi-agent path
planning with evolutionary reinforcement learning
in mixed dynamic environments. arXiv preprint
arXiv:2007.15724.
Mason, G., Calinescu, R., Kudenko, D., and Banks, A.
(2018). Assurance in reinforcement learning using
quantitative verification. In Advances in Hybridiza-
tion of Intelligent Methods, pages 71–96. Springer.
Mason, G. R., Calinescu, R. C., Kudenko, D., and Banks, A.
(2017). Assured reinforcement learning with formally
verified abstract policies. In 9th International Confer-
ence on Agents and Artificial Intelligence (ICAART).
York.
Moldovan, T. M. (2012). Safe exploration in markov deci-
sion processes. arXiv preprint arXiv:1205.4810.
Parker, D. and Norman, G. (2014). Quantitative verifica-
tion: Formal guarantees for timeliness reliability and
performance. a Knowledge Transfer Report from the
London Mathematical Society and Smith Institute for
Industrial Mathematics and System Engineering.
Patel, P. G., Carver, N., and Rahimi, S. (2011). Tuning
computer gaming agents using q-learning. In 2011
Federated Conference on Computer Science and In-
formation Systems (FedCSIS), pages 581–588.
Portugal, D., Iocchi, L., and Farinelli, A. (2019). A ros-
based framework for simulation and benchmarking of
multi-robot patrolling algorithms. In Robot Operating
System (ROS), pages 3–28.
Portugal, D. and Rocha, R. P. (2016). Cooperative multi-
robot patrol with bayesian learning. Autonomous
Robots, 40(5):929–953.
Schwager, M., Dames, P., Rus, D., and Kumar, V. (2017).
A multi-robot control policy for information gather-
ing in the presence of unknown hazards. In Robotics
research, pages 455–472. Springer.
Serrano-Cuevas, J., Morales, E. F., and Hern
´
andez-Leal, P.
(2019). Safe reinforcement learning using risk map-
ping by similarity.
Shalev-Shwartz, S., Shammah, S., and Shashua, A. (2016).
Safe, multi-agent, reinforcement learning for au-
tonomous driving. arXiv preprint arXiv:1610.03295.
Xiao, D. and Tan, A.-H. (2008). Scaling up multi-agent
reinforcement learning in complex domains. In Int.
Conf. Web Intelligence and Intelligent Agent Technol-
ogy, volume 2, pages 326–329.
Yu, M., Yang, Z., Kolar, M., and Wang, Z. (2019). Conver-
gent policy optimization for safe reinforcement learn-
ing. In Advances in Neural Information Processing
Systems, pages 3127–3139.
Zhang, W., Bastani, O., and Kumar, V. (2019).
Mamps: Safe multi-agent reinforcement learning
via model predictive shielding. arXiv preprint
arXiv:1910.12639.
Zhu, C. et al. (2019). A q-values sharing framework for
multiple independent q-learners. In 18th Conf. Au-
tonomous Agents and MultiAgent Systems, volume 1,
pages 2324–2326.
Reinforcement Learning with Quantitative Verification for Assured Multi-Agent Policies
245