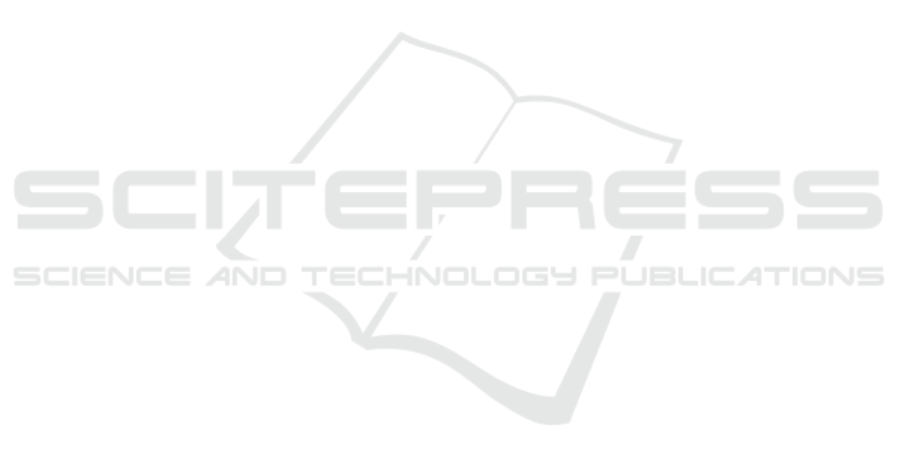
racy of 65.11% at a coverage of 63.85% (i.e. with
36.15% missing) by tenfold cross-validation, which
is very similar to the previous result. When we ap-
ply this model which was only trained on feedback
on the feedback from 2012, we get again coverage of
61.10% (38.90% missing) with an accuracy of only
49.13%. Even pooling all feedback from 2010 to
2013 and evaluating on 2014 does not improve this.
So, clearly our initial data is more useful than
feedback alone even when we correct for the un-
equal class distribution, perhaps because it did not
include noise. We therefore speculate that the non-
improvement of performance indicates a limitation
of the used feature set rather than lack of training
data. Perhaps orders of magnitude more training data
would be needed to enable higher coverage, or per-
haps the signal-noise-ratio of the audio samples with
background noise is simply too low.
6 CONCLUSION
We have implemented and deployed a system for
dance music genre classification, which determined
the correct dancing style with about 73% accuracy
and a coverage of 61% (i.e. reporting an unknown
class in 39% of cases). While the accuracy was as
expected, the coverage was below expectations. The
dancing style was reported in near-real-time – about
4s after recording a 3s sample, and later below 1s –
and was therefore suitable for the intended purpose of
helping novice dancing students to find the appropri-
ate dance for a given live or recorded music.
We have deployed the system for seven years, and
it was extensively used from 2011 to 2014 by about
50,000 users, each of which used it about 84 times per
year (7 times per month). Feedback by users agree
well with our previous estimate of the models’ per-
formance, which was most likely due to the fact that
– instead of analyzing the audio samples directly –
we recorded them with a variety of smartphone mi-
crophones in rooms similar to dancing halls, simu-
lating acoustic characteristics albeit not background
noise, before audio analysis. However we neglected
to account for and model realistic background noise
which might explain the observed lower coverage in
the field.
While our system was intended for continual
improvement by integrating users’ feedback, this part
did not work well. It may be that the initial feature
set which we chose after extensive experiments was
too restricted to enable a better performance, or the
feedback may be of insufficient quality. The latter
would however be surprising as the accuracy agrees
quite well with pre-deployment estimates.
ACKNOWLEDGEMENTS
We would like to thank all volunteer translators and
all others who have helped to improve our sys-
tem, provided feedback, dance music or test phones,
most notably Andreas H., Gregoire A., Christoph B.,
Ond
ˆ
rej J., Witono H., Melanie M., Paolo P., Martin P.,
Sonja S., Herbert S. and Arthur K.
REFERENCES
Arzt, A. and Widmer, G. (2010). Towards effective ‘any-
time’music tracking. In
˚
Agotnes, T., editor, Proceed-
ings of the Fifth Starting AI Researchers’ Symposium,
pages 24–29.
Chew, E., Volk, A., and Lee, C.-Y. (2005). Dance music
classification using inner metric analysis. In The next
wave in computing, optimization, and decision tech-
nologies, pages 355–370. Springer.
Dixon, S. (2007). Evaluation of the audio beat tracking
system beatroot. Journal of New Music Research,
36(1):39–50.
Dixon, S., Pampalk, E., and Widmer, G. (2003). Classifica-
tion of dance music by periodicity patterns. In Pro-
ceedings of the Fourth International Conference on
Music Information Retrieval, Baltimore (ML), USA.
Gouyon, F. and Dixon, S. (2004). Dance music classifi-
cation: A tempo-based approach. In Proceedings of
the 5th International Conference on Music Informa-
tion Retrieval, Barcelona, Spain.
Gouyon, F., Dixon, S., Pampalk, E., and Widmer, G.
(2004). Evaluating rhythmic descriptors for musical
genre classification. In Proceedings of the AES 25th
International Conference, pages 196–204.
Medhat, F., Chesmore, D., and Robinson, J. (2017). Auto-
matic classification of music genre using masked con-
ditional neural networks. In 2017 IEEE International
Conference on Data Mining (ICDM), pages 979–984.
IEEE.
Platt, J. (1999). Fast training of support vector machines us-
ing sequential minimal optimization. advances in ker-
nel methods—support vector learning (pp. 185–208).
AJ, MIT Press, Cambridge, MA.
Wang, A. (2003). An industrial-strength audio search algo-
rithm. In Choudhury, T. and Manus, S., editors, ISMIR
2003, 4th Symposium Conference on Music Informa-
tion Retrieval, pages 7–13.
Yang, R., Feng, L., Wang, H., Yao, J., and Luo, S. (2020).
Parallel recurrent convolutional neural networks based
music genre classification method for mobile devices.
IEEE Access, Preprint.
ICAART 2021 - 13th International Conference on Agents and Artificial Intelligence
898