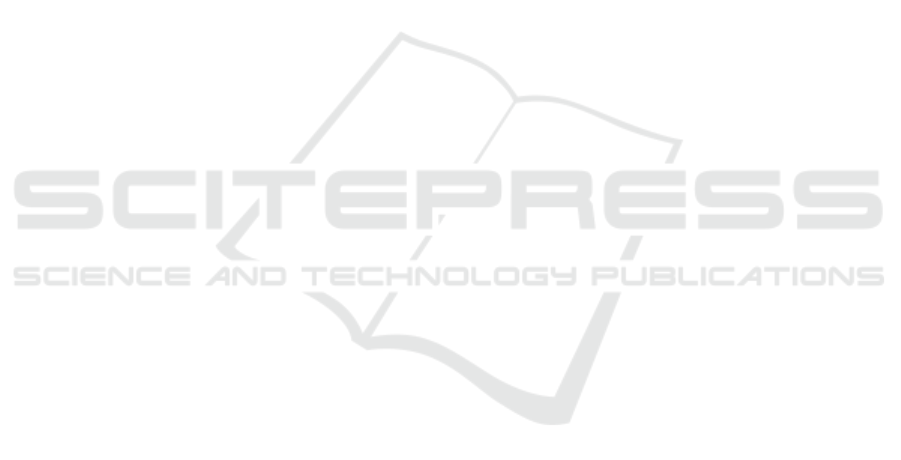
6 CONCLUSIONS AND FUTURE
WORK
We have presented a formulation of the Tail Assign-
ment Problem as a QUBO model to be solved using
QA based on the current commercial solution devel-
oped by D-Wave Systems. Our results demonstrate
that using an hybrid solver for the proposed QUBO
model may represent a considerable advantage in the
probability of finding valid non-expensive solutions
when compared with a classical solver. Nonetheless,
it is relevant to note that, even though it may show
encouraging results, quantum computation is in an
early stage and, therefore, the current limitations do
not allow scaling it to complete real-world datasets.
Throughout this study, we opted to implement mul-
tiple simplifications to narrow the scope of the prob-
lem in analysis. A non-consideration of minor main-
tenance tasks is not realistic as they have to occur in
a real scenario. Furthermore, a robust approach may
be a pivotal achievement as flight delays are frequent
and tight schedules can be significantly affected by
that. Additionally, to understand the effectiveness in
a deeper level of the proposed modelling technique,
when applied to multiple solvers using different sce-
narios, it would be important to run more tests using
different datasets. Finally, as HSS revealed to perform
better for solving this problem than using only a clas-
sical algorithm such as SA, further studies on hybrid
solvers could be relevant for a better understanding
on the real advantage of using such technique to solve
complex optimisation problems.
REFERENCES
Albash, T. and Lidar, D. A. (2018). Adiabatic quantum
computation. Reviews of Modern Physics, 90(1).
Chapuis, G., Djidjev, H., Hahn, G., and Rizk, G. (2019).
Finding maximum cliques on the d-wave quantum
annealer. Journal of Signal Processing Systems,
91(3):363–377.
Cruz-Santos, W., Venegas-Andraca, S. E., and Lanzagorta,
M. (2019). A QUBO Formulation of Minimum Multi-
cut Problem Instances in Trees for D-Wave Quantum
Annealers. Scientific Reports, 9(1):1–12.
Froyland, G., Maher, S. J., and Wu, C. L. (2013). The recov-
erable robust tail assignment problem. Transportation
Science, 48(3):351–372.
Gr
¨
onkvist, M. (2005). The tail assignment problem.
PhD thesis, Chalmers University of Technology and
G
¨
oteborg University.
Ikeda, K., Nakamura, Y., and Humble, T. S. (2019). Appli-
cation of quantum annealing to nurse scheduling prob-
lem. Scientific Reports, 9(1):12837.
Kadowaki, T. and Nishimori, H. (1998). Quantum an-
nealing in the transverse ising model. Phys. Rev. E,
58:5355–5363.
Lucas, A. (2014). Ising formulations of many NP problems.
Frontiers in Physics, 2(February):1–14.
Montoito, F. (2016). Application of the Simulated Anneal-
ing with Adaptive Local Neighborhood Search to the
Tail Assignment Problem The Case Study of TAP.
Master’s thesis, Instituto Superior T
´
ecnico, Universi-
dade de Lisboa.
Neukart, F., Compostella, G., Seidel, C., von Dollen, D.,
Yarkoni, S., and Parney, B. (2017). Traffic flow opti-
mization using a quantum annealer. Frontiers in ICT,
4:29.
Papalitsas, C., Andronikos, T., Giannakis, K.,
Theocharopoulou, G., and Fanarioti, S. (2019).
A QUBO model for the traveling salesman problem
with time windows. Algorithms, 12(11):1–18.
Ray, P., Chakrabarti, B. K., and Chakrabarti, A. (1989).
Sherrington-kirkpatrick model in a transverse field:
Absence of replica symmetry breaking due to quan-
tum fluctuations. Phys. Rev. B, 39:11828–11832.
Ruther, S., Boland, N., Engineer, F. G., and Evans, I.
(2017). Integrated aircraft routing, crew pairing, and
tail assignment: Branch-and-price with many pricing
problems. Transportation Science, 51(1):177–195.
Stollenwerk, T., Lobe, E., and Jung, M. (2019). Flight
Gate Assignment with a Quantum Annealer. Lecture
Notes in Computer Science (including subseries Lec-
ture Notes in Artificial Intelligence and Lecture Notes
in Bioinformatics), 11413 LNCS:99–110.
Tran, T. T., Do, M., Rieffel, E. G., Frank, J., Wang,
Z., O’Gorman, B., Venturelli, D., and Beck, J. C.
(2016). A hybrid quantum-classical approach to solv-
ing scheduling problems. In SOCS.
Ushijima-Mwesigwa, H., Negre, C. F. A., and Mniszewski,
S. M. (2017). Graph partitioning using quantum an-
nealing on the d-wave system. In Proceedings of the
2nd International Workshop on Post Moores Era Su-
percomputing, page 22–29, New York, USA. Associ-
ation for Computing Machinery.
Venturelli, D., Marchand, D. J. J., and Rojo, G. (2016). Job
Shop Scheduling Solver based on Quantum Anneal-
ing. arXiv:1506.08479 [quant-ph].
Vikst
˚
al, P., Gr
¨
onkvist, M., Svensson, M., Andersson, M.,
Johansson, G., and Ferrini, G. (2019). Applying the
Quantum Approximate Optimization Algorithm to the
Tail Assignment Problem. arXiv:1912.10499 [quant-
ph].
Yadav, K. K. (2017). An Effective and Efficient Solution
to Tail Assignment Problem. International Journal
of Advanced Research in Computer Science and Soft-
ware Engineering, 7(4):378–386.
ICAART 2021 - 13th International Conference on Agents and Artificial Intelligence
906