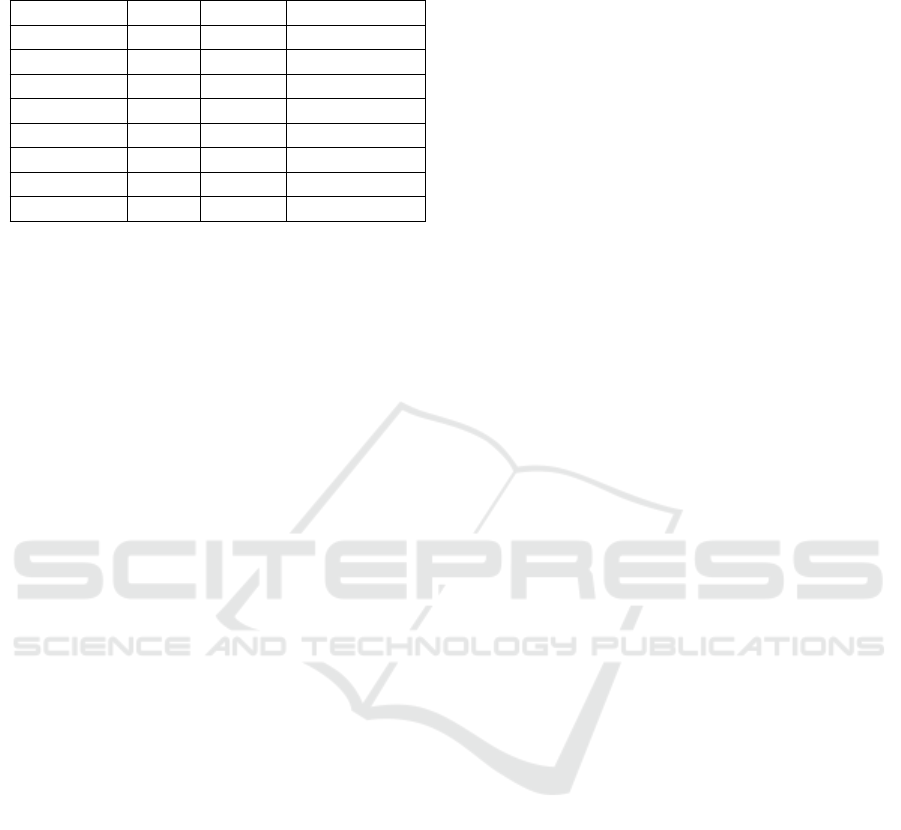
Table 4: Hyperparameter optimization for the GNN exper-
iment on the 5G vs Non-Conspiracy classification problem.
Batch Size Units Layers Accuracy
32 32 3 64.2% 8.4%
32 32 5 62.3% 8.0%
32 64 2 64.8% 7.2%
32 64 5 64.8% 8.9%
128 32 3 67.4% 9.0%
128 32 5 62.7% 7.4%
128 64 2 64.4% 6.7%
128 64 5 66.7% 7.9%
10 CONCLUSION
We have presented a new dataset of Twitter graphs
associated with the spread of misinformation related
to COVID-19, particularly conspiracy theories con-
nected to 5G. This dataset can be used to train graph-
based misinformation detection methods. The dataset
is comparatively small, which is due to the effort
required for manual labeling, and because very few
such misinformation tweets have a substantial num-
ber of retweets. We have used basic classifiers to
verify that ML-based classification is possible and to
establish a baseline accuracy that more sophisticated
systems can compare against. However, since the ul-
timate goal for such systems will be to moderate or
at least flag content in social media, we believe that
explainability will be a quality in addition to high ac-
curacy, a consideration that was also pointed out in
previous work (Reis et al., 2019).
In the future, we will pursue the approach of find-
ing candidate misinformation tweets among the sta-
tuses that have sizeable subgraphs associated with
them using natural language processing. The re-
sulting candidate statuses can then be labeled man-
ually. The labeling showed that the differences be-
tween misinformation spreading and other Tweets can
be very subtle. Thus we believe that manual labeling
remains necessary as current NLP methods are not ca-
pable of reliably identifying misinformation. How-
ever, such methods can be developed with the help of
the labeled datasets. We assume that hybrid methods
that combine graph and NLP based approaches will
be the key to obtain reliable misinformation detection
systems.
REFERENCES
Ahmed, S., Hinkelmann, K., and Corradini, F. (2019).
Combining machine learning with knowledge engi-
neering to detect fake news in social networks-a sur-
vey. In Proceedings of the AAAI 2019 Spring Sympo-
sium, volume 12.
Ali, H. S. and Kurasawa, F. (2020). #covid19: Social media
both a blessing and a curse during coronavirus pan-
demic. https://bit.ly/3bjVQgQ.
Breiman, L. (2001). Random forests. Machine learning,
45(1):5–32.
Burkhardt, J. M. (2017). History of fake news. Library
Technology Reports, 53(8):5–9.
Castelo, S., Almeida, T., Elghafari, A., Santos, A., Pham,
K., Nakamura, E., and Freire, J. (2019). A topic-
agnostic approach for identifying fake news pages. In
Companion Proceedings of The 2019 World Wide Web
Conference, WWW ’19, page 975980, New York, NY,
USA. Association for Computing Machinery.
Cui, L. and Lee, D. (2020). Coaid: Covid-19 healthcare
misinformation dataset.
Cui, L., Seo, H., Tabar, M., Ma, F., Wang, S., and Lee, D.
(2020). Deterrent: Knowledge guided graph attention
network for detecting healthcare misinformation. In
Proceedings of the 26th ACM SIGKDD International
Conference on Knowledge Discovery & Data Mining,
KDD ’20, page 492502, New York, NY, USA. Asso-
ciation for Computing Machinery.
Dai, E., Sun, Y., and Wang, S. (2020). Ginger cannot cure
cancer: Battling fake health news with a comprehen-
sive data repository. In Proceedings of the Interna-
tional AAAI Conference on Web and Social Media,
volume 14, pages 853–862.
de Beer, D. and Matthee, M. (2020). Approaches to iden-
tify fake news: A systematic literature review. In
Antipova, T., editor, Integrated Science in Digital
Age 2020, pages 13–22, Cham. Springer International
Publishing.
Dhoju, S., Main Uddin Rony, M., Ashad Kabir, M., and
Hassan, N. (2019). Differences in health news from
reliable and unreliable media. In Companion Proceed-
ings of The 2019 World Wide Web Conference, pages
981–987.
Errica, F., Podda, M., Bacciu, D., and Micheli, A. (2020).
A fair comparison of graph neural networks for graph
classification. In International Conference on Learn-
ing Representations.
European External Action Service (EEAS) (2020). Disin-
formation can kill. https://bit.ly/32FKlwb.
Flaxman, S., Goel, S., and Rao, J. M. (2016). Filter Bub-
bles, Echo Chambers, and Online News Consumption.
Public Opinion Quarterly, 80(S1):298–320.
Ghebreyesus, T. A. and Ng, N. (2020). How the who is
leading the fight against coronavirus misinformation.
Ghenai, A. and Mejova, Y. (2018). Fake cures: user-centric
modeling of health misinformation in social media.
Proceedings of the ACM on human-computer interac-
tion, 2(CSCW):1–20.
Hassan, N., Arslan, F., Li, C., and Tremayne, M. (2017).
Toward automated fact-checking: Detecting check-
worthy factual claims by claimbuster. In Proceedings
of the 23rd ACM SIGKDD International Conference
on Knowledge Discovery and Data Mining, KDD ’17,
WICO Graph: A Labeled Dataset of Twitter Subgraphs based on Conspiracy Theory and 5G-Corona Misinformation Tweets
265